Big Data Fundamentals
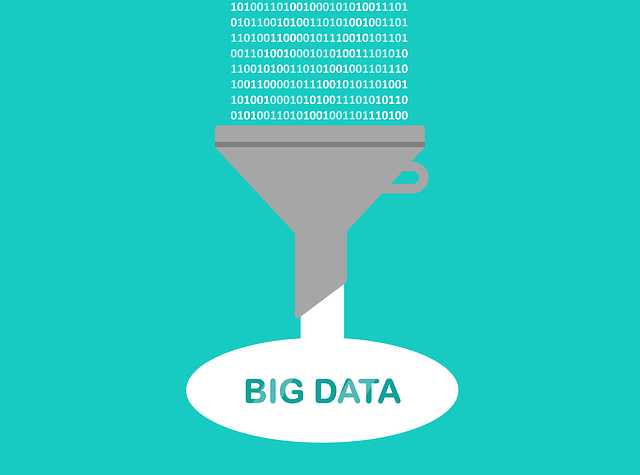
Course Objectives
· Define and describe Big Data and its role in the corporate world
· Understand the need for Distributed Computing
· Understand the role of Hadoop in a distributed computing setup
· Understanding the different install modes available for Hadoop
· Installing Hadoop in Standalone mode
· Installing Hadoop in Pseudo-Distributed mode
· Understanding the components of HDFS
· Moving files in and out of HDFS
· Managing replication strategies for data nodes
· Management strategies for failure data and name nodes
· Setting up a MapReduce job for a simple counting task
· Submitting a MapReduce job to Hadoop and monitoring it
· Understanding how YARN schedules tasks
· Introduction to basic technologies which work on Hadoop
------------------------------------------------------------------------------------------------------------
Course Description
Big Data Fundamentals online course get started with big data foundation concepts. Big Data Fundamentals online course intention is to provide a foundation to handle big chunk of data. Big Data Fundamentals online course will allow the participants to understand the benefits of understanding big data concepts.
Big Data Fundamentals online course is ideally developed for data analysts who wants to expertise in big data concepts and applications.
In the Big Data Fundamentals online training course, Uplatz provides an in-depth online training for the participants or learners to gain knowledge and able to manage large data efficiently with the help of emerging technology in real world. Uplatz provides appropriate teaching and expertise training to equip the participants for implementing the learnt concepts in an enterprise.
Big Data Fundamentals online training course machine learning introduction, fundamentals, architecture, technology and use-cases.
With the help of Big Data Fundamentals online course, the learners can discover:
-
Inhouse Terminology and concepts related to the Big Data Fundamentals
-
Describe and understand relationship with data
-
Describe data with the help of use-cases and architecture
-------------------------------------------------------------------------------------------------------------------
Big Data Fundamentals
- Module 01: Big Data Overview
- Module 02: Hadoop Introduction, HDFS and MapReduce
- Module 03: Hadoop Install and Configure
- Module 04: Hadoop Data Ingest using Apache NiFi
- Module 05: Data Processing using MapReduce framework
- Module 06: Managing and Scheduling Tasks using YARN Management System
- Module 07: HDFS data visualization using Microsoft Power BI
- Module 08: Latest trend technologies edge Big Data Solutions
-------------------------------------------------------------------------------------------------------------------
Big Data Fundamentals online certification course with the help of expert professionals training is recognized across the globe. Because of the increased adoption of the large data applications in various companies the participants are able to find the job opportunity easily. The leading companies hire big data engineer considering their skill of mastering data analytics and technology. Big Data Fundamentals online certification course is known for their knowledge in managing data analytics tools. After pursuing Big Data Fundamentals online certification course the participants can become as a machine learning analyst, Data analyst, business analyst, business analytics manager, Data scientist and can pursue a wide range of career paths.
------------------------------------------------------------------------------
------------------------------------------------------------------------------
Big Data Interview Questions and Answers
------------------------------------------------------------------------------
1) Explain “Big Data” and what are five V’s of Big Data?
“Big data” is the term for a collection of large and complex data sets, that makes it difficult to process using relational database management tools or traditional data processing applications. It is difficult to capture, curate, store, search, share, transfer, analyze, and visualize Big data. Big Data has emerged as an opportunity for companies. Now they can successfully derive value from their data and will have a distinct advantage over their competitors with enhanced business decisions making capabilities.
2) What is Hadoop and its components?
When “Big Data” emerged as a problem, Apache Hadoop evolved as a solution to it. Apache Hadoop is a framework which provides us various services or tools to store and process Big Data. It helps in analyzing Big Data and making business decisions out of it, which can’t be done efficiently and effectively using traditional systems.
3) What are HDFS and YARN?
HDFS (Hadoop Distributed File System) is the storage unit of Hadoop. It is responsible for storing different kinds of data as blocks in a distributed environment. It follows master and slave topology.
YARN (Yet Another Resource Negotiator) is the processing framework in Hadoop, which manages resources and provides an execution environment to the processes.
4) What are the real-time industry applications of Hadoop?
Hadoop, well known as Apache Hadoop, is an open-source software platform for scalable and distributed computing of large volumes of data. It provides rapid, high performance, and cost-effective analysis of structured and unstructured data generated on digital platforms and within the enterprise. It is used in almost all departments and sectors today.
Here are some of the instances where Hadoop is used:
- Managing traffic on streets
- Streaming processing
- Content management and archiving e-mails
- Processing rat brain neuronal signals using a Hadoop computing cluster
- Fraud detection and prevention
- Advertisements targeting platforms are using Hadoop to capture and analyze click stream, transaction, video, and social media data
- Managing content, posts, images, and videos on social media platforms
- Analyzing customer data in real time for improving business performance
- Public sector fields such as intelligence, defense, cyber security, and scientific research
- Getting access to unstructured data such as output from medical devices, doctor’s notes, lab results, imaging reports, medical correspondence, clinical data, and financial data
5) How is Hadoop different from other parallel computing systems?
Hadoop is a distributed file system that lets you store and handle massive amounts of data on a cloud of machines, handling data redundancy.
The primary benefit of this is that since data is stored in several nodes, it is better to process it in a distributed manner. Each node can process the data stored on it instead of spending time on moving the data over the network.
On the contrary, in the relational database computing system, we can query data in real time, but it is not efficient to store data in tables, records, and columns when the data is huge.
Hadoop also provides a scheme to build a column database with Hadoop HBase for runtime queries on rows.
6) In what all modes Hadoop can be run?
Hadoop can be run in three modes:
- Standalone mode:The default mode of Hadoop, it uses local file system for input and output operations. This mode is mainly used for the debugging purpose, and it does not support the use of HDFS. Further, in this mode, there is no custom configuration required for mapred-site.xml, core-site.xml, and hdfs-site.xml files. This mode works much faster when compared to other modes.
- Pseudo-distributed mode (Single-node Cluster):In this case, you need configuration for all the three files mentioned above. In this case, all daemons are running on one node, and thus both Master and Slave nodes are the same.
Fully distributed mode (Multi-node Cluster): This is the production phase of Hadoop (what Hadoop is known for) where data is used and distributed across several nodes on a Hadoop cluster. Separate nodes are allotted as Master and Slave.
7) Explain the major difference between HDFS block and InputSplit.
In simple terms, a block is the physical representation of data while split is the logical representation of data present in the block. Split acts as an intermediary between the block and the mapper.
Suppose we have two blocks:
Block 1: ii nntteell
Block 2: Ii ppaatt
Now considering the map, it will read Block 1 from ii to ll but does not know how to process Block 2 at the same time. Here comes Split into play, which will form a logical group of Block 1 and Block 2 as a single block.
It then forms a key–value pair using InputFormat and records reader and sends map for further processing with InputSplit. If you have limited resources, you can increase the split size to limit the number of maps. For instance, if there are 10 blocks of 640 MB (64 MB each) and there are limited resources, you can assign ‘split size’ as 128 MB. This will form a logical group of 128 MB, with only 5 maps executing at a time.
However, if the ‘split size’ property is set to false, the whole file will form one InputSplit and is processed by a single map, consuming more time when the file is bigger.
8) What is distributed cache? What are its benefits?
Distributed cache in Hadoop is a service by MapReduce framework to cache files when needed.
Once a file is cached for a specific job, Hadoop will make it available on each DataNode both in system and in memory, where map and reduce tasks are executing. Later, you can easily access and read the cache file and populate any collection (like array, hashmap) in your code.
Benefits of using distributed cache are as follows:
- It distributes simple, read-only text/data files and/or complex types such as jars, archives, and others. These archives are then un-archived at the slave node.
- Distributed cache tracks the modification timestamps of cache files, which notify that the files should not be modified until a job is executed.
9) Explain the difference between NameNode, Checkpoint NameNode, and Backup Node.
- NameNode is the core of HDFS that manages the metadata—the information of which file maps to which block locations and which blocks are stored on which DataNode. In simple terms, it’s the data about the data being stored. NameNode supports a directory tree-like structure consisting of all the files present in HDFS on a Hadoop cluster. It uses the following files for namespace:
- fsimage file: It keeps track of the latest Checkpoint of the namespace.
- edits file: It is a log of changes that have been made to the namespace since Checkpoint.
- Checkpoint NameNode has the same directory structure as NameNode and creates Checkpoints for namespace at regular intervals by downloading the fsimage, editing files, and margining them within the local directory. The new image after merging is then uploaded to NameNode. There is a similar node like Checkpoint, commonly known as the Secondary Node, but it does not support the ‘upload to NameNode’ functionality.
- Backup Node provides similar functionality as Checkpoint, enforcing synchronization with NameNode. It maintains an up-to-date in-memory copy of the file system namespace and doesn’t require getting hold of changes after regular intervals. The Backup Node needs to save the current state in-memory to an image file to create a new Checkpoint.
10) How is Hadoop related to Big Data?
When we talk about Big Data, we talk about Hadoop. So, this is another Big Data interview question that you will definitely face in an interview.
Hadoop is an open-source framework for storing, processing, and analyzing complex unstructured data sets for deriving insights and intelligence.
11) What do you mean by commodity hardware?
This is yet another Big Data interview question you’re most likely to come across in any interview you sit for.
Commodity Hardware refers to the minimal hardware resources needed to run the Apache Hadoop framework. Any hardware that supports Hadoop’s minimum requirements is known as ‘Commodity Hardware.’
12) Define and describe the term FSCK.
FSCK stands for Filesystem Check. It is a command used to run a Hadoop summary report that describes the state of HDFS. It only checks for errors and does not correct them. This command can be executed on either the whole system or a subset of files.
13) What is the purpose of the JPS command in Hadoop?
The JPS command is used for testing the working of all the Hadoop daemons. It specifically tests daemons like NameNode, DataNode, ResourceManager, NodeManager and more.
14) Name the different commands for starting up and shutting down Hadoop Daemons.
(this is one of the most important Big Data interview questions to help the interviewer gauge your knowledge of commands)
To start all the daemons:
./sbin/start-all.sh
To shut down all the daemons:
./sbin/stop-all.sh
15) Why do we need Hadoop for Big Data Analytics?
In most cases, Hadoop helps in exploring and analyzing large and unstructured data sets. Hadoop offers storage, processing and data collection capabilities that help in analytics.
16) Explain the different features of Hadoop.
Open-Source – Hadoop is an open-sourced platform. It allows the code to be rewritten or modified according to user and analytics requirements.
Scalability – Hadoop supports the addition of hardware resources to the new nodes.
Data Recovery – Hadoop follows replication which allows the recovery of data in the case of any failure.
Data Locality – This means that Hadoop moves the computation to the data and not the other way round. This way, the whole process speeds up.
17) Define the Port Numbers for NameNode, Task Tracker and Job Tracker.
NameNode – Port 50070
Task Tracker – Port 50060
Job Tracker – Port 50030
18) Define DataNode. How does NameNode tackle DataNode failures?
DataNode stores data in HDFS; it is a node where actual data resides in the file system. Each DataNode sends a heartbeat message to notify that it is alive. If the NameNode does not receive a message from the DataNode for 10 minutes, the NameNode considers the DataNode to be dead or out of place and starts the replication of blocks that were hosted on that DataNode such that they are hosted on some other DataNode. A BlockReport contains a list of the all blocks on a DataNode. Now, the system starts to replicate what were stored in the dead DataNode.
The NameNode manages the replication of the data blocks from one DataNode to another. In this process, the replication data gets transferred directly between DataNodes such that the data never passes the NameNode.
19) What are the core methods of a Reducer?
The three core methods of a Reducer are as follows:
1. setup(): This method is used for configuring various parameters such as input data size and distributed cache.
public void setup (context)
2. reduce(): Heart of the Reducer is always called once per key with the associated reduced task.
public void reduce(Key, Value, context)
3. cleanup(): This method is called to clean the temporary files, only once at the end of the task.
public void cleanup (context)
20) What is a SequenceFile in Hadoop?
Extensively used in MapReduce I/O formats, SequenceFile is a flat file containing binary key–value pairs. The map outputs are stored as SequenceFile internally. It provides Reader, Writer, and Sorter classes. The three SequenceFile formats are as follows:
1. Uncompressed key–value records
2. Record compressed key–value records—only ‘values’ are compressed here
3. Block compressed key–value records—both keys and values are collected in ‘blocks’ separately and compressed. The size of the ‘block’ is configurable
21) What will you do when NameNode is down?
The NameNode recovery process involves the following steps to make the Hadoop cluster up and running:
1. Use the file system metadata replica (FsImage) to start a new NameNode.
2. Then, configure the DataNodes and clients so that they can acknowledge this new NameNode, that is started.
3. Now the new NameNode will start serving the client after it has completed loading the last checkpoint FsImage (for metadata information) and received enough block reports from the DataNodes.
22) How is HDFS fault tolerant?
When data is stored over HDFS, NameNode replicates the data to several DataNode. The default replication factor is 3. You can change the configuration factor as per your need. If a DataNode goes down, the NameNode will automatically copy the data to another node from the replicas and make the data available. This provides fault tolerance in HDFS.
23) What is the use of RecordReader in Hadoop?
Though InputSplit defines a slice of work, it does not describe how to access it. Here is where the RecordReader class comes into the picture, which takes the byte-oriented data from its source and converts it into record-oriented key–value pairs such that it is fit for the Mapper task to read it. Meanwhile, InputFormat defines this Hadoop RecordReader instance.
24) What is Speculative Execution in Hadoop?
One limitation of Hadoop is that by distributing the tasks on several nodes, there are chances that few slow nodes limit the rest of the program. There are various reasons for the tasks to be slow, which are sometimes not easy to detect. Instead of identifying and fixing the slow-running tasks, Hadoop tries to detect when the task runs slower than expected and then launches other equivalent tasks as backup. This backup mechanism in Hadoop is speculative execution.
It creates a duplicate task on another disk. The same input can be processed multiple times in parallel. When most tasks in a job comes to completion, the speculative execution mechanism schedules duplicate copies of the remaining tasks (which are slower) across the nodes that are free currently. When these tasks are finished, it is intimated to the JobTracker. If other copies are executing speculatively, Hadoop notifies the TaskTrackers to quit those tasks and reject their output.
Speculative execution is by default true in Hadoop. To disable it, we can set mapred.map.tasks.speculative.execution and mapred.reduce.tasks.speculative.execution
JobConf options to false.
25) What happens if you try to run a Hadoop job with an output directory that is already present?
It will throw an exception saying that the output file directory already exists.
To run the MapReduce job, you need to ensure that the output directory does not exist in the HDFS.
To delete the directory before running the job, we can use shell:
Hadoop fs –rmr /path/to/your/output/
Or the Java API:
FileSystem.getlocal(conf).delete(outputDir, true);
26) How can you debug Hadoop code?
First, we should check the list of MapReduce jobs currently running. Next, we need to see that there are no orphaned jobs running; if yes, we need to determine the location of RM logs.
1. Run:
ps –ef | grep –I ResourceManager
Then, look for the log directory in the displayed result. We have to find out the job ID from the displayed list and check if there is any error message associated with that job.
2. On the basis of RM logs, we need to identify the worker node that was involved in the execution of the task.
3. Now, we will login to that node and run the below code:
ps –ef | grep –iNodeManager
4. Then, we will examine the Node Manager log. The majority of errors come from the user-level logs for each MapReduce job.
27) What are active and passive “NameNodes”?
In HA (High Availability) architecture, we have two NameNodes – Active “NameNode” and Passive “NameNode”.
- Active “NameNode” is the “NameNode” which works and runs in the cluster.
- Passive “NameNode” is a standby “NameNode”, which has similar data as active “NameNode”.
When the active “NameNode” fails, the passive “NameNode” replaces the active “NameNode” in the cluster. Hence, the cluster is never without a “NameNode” and so it never fails.
28) Why does one remove or add nodes in a Hadoop cluster frequently?
One of the most attractive features of the Hadoop framework is its utilization of commodity hardware. However, this leads to frequent “DataNode” crashes in a Hadoop cluster. Another striking feature of Hadoop Framework is the ease of scale in accordance with the rapid growth in data volume. Because of these two reasons, one of the most common task of a Hadoop administrator is to commission (Add) and decommission (Remove) “Data Nodes” in a Hadoop Cluster.
Read this blog to get a detailed understanding on commissioning and decommissioning nodes in a Hadoop cluster.
29) What happens when two clients try to access the same file in the HDFS?
When the first client contacts the “NameNode” to open the file for writing, the “NameNode” grants a lease to the client to create this file. When the second client tries to open the same file for writing, the “NameNode” will notice that the lease for the file is already granted to another client, and will reject the open request for the second client.
30) Can NameNode and DataNode be a commodity hardware?
The smart answer to this question would b