Apache Spark and PySpark
Master Big Data Processing with Apache Spark & PySpark – Essentials for Data Engineering
95% Started a new career BUY THIS COURSE (
USD 12 USD 41 )-
89% Got a pay increase and promotion
Students also bought -
-
- Amazon Web Services (AWS)
- 28 Hours
- USD 12
- 940 Learners
-
- Data Engineering with Talend
- 17 Hours
- USD 12
- 540 Learners
-
- Data Governance
- 4 Hours
- USD 12
- 0 Learners
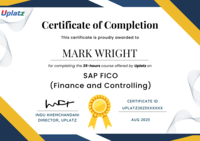
Apache Spark & PySpark Essentials – Self-Paced Online Course
Kickstart your career in big data and data engineering with this comprehensive training on Apache Spark and PySpark, the most powerful tools for large-scale data processing and real-time analytics. Delivered as a self-paced online course, this program includes high-quality video lectures, hands-on examples, and project-based learning. Upon completion, you will receive a Course Completion Certificate from Uplatz.
Apache Spark is an open-source, distributed computing engine designed for fast processing of big data across clusters. PySpark is its Python API, enabling Python developers to tap into Spark’s high-performance capabilities for data transformation, machine learning, and streaming.
This course gives a solid foundation in Spark architecture, RDDs, DataFrames, Spark SQL, and the PySpark programming model. You’ll also explore Spark’s integration with big data tools like Hadoop and Hive, making it ideal for aspiring data engineers, analysts, and backend developers.
By the end of this course, learners will be able to:
- Understand Big Data fundamentals and the need for distributed data processing.
- Learn the Apache Spark architecture, including driver, executors, DAG, and cluster modes.
- Master RDDs and DataFrames, and how to manipulate data efficiently in memory.
- Use PySpark to build scalable data pipelines using Python.
- Perform data transformations and actions using Spark's core APIs.
- Work with Spark SQL to query structured data using SQL-like syntax.
- Integrate Spark with HDFS, Hive, and other data sources for data ingestion and processing.
- Explore Spark MLlib for scalable machine learning workflows.
- Build and manage ETL pipelines and understand Spark’s role in modern data engineering.
- Optimize Spark jobs with caching, partitioning, and tuning techniques.
Apache Spark and PySpark Essentials for Data Engineering - Course Syllabus
This course is designed to provide a comprehensive understanding of Spark and PySpark, from basic concepts to advanced implementations, to ensure you well-prepared to handle large-scale data analytics in the real world. The course includes a balance of theory, hands-on practice including project work.
- Introduction to Apache Spark
- Introduction to Big Data and Apache Spark, Overview of Big Data
- Evolution of Spark: From Hadoop to Spark
- Spark Architecture Overview
- Key Components of Spark: RDDs, DataFrames, and Datasets
- Installation and Setup
- Setting Up Spark in Local Mode (Standalone)
- Introduction to the Spark Shell (Scala & Python)
- Basics of PySpark
- Introduction to PySpark: Python API for Spark
- PySpark Installation and Configuration
- Writing and Running Your First PySpark Program
- Understanding RDDs (Resilient Distributed Datasets)
- RDD Concepts: Creation, Transformations, and Actions
- RDD Operations: Map, Filter, Reduce, GroupBy, etc.
- Persisting and Caching RDDs
- Introduction to SparkContext and SparkSession
- SparkContext vs. SparkSession: Roles and Responsibilities
- Creating and Managing SparkSessions in PySpark
- Working with DataFrames and SparkSQL
- Introduction to DataFrames
- Understanding DataFrames: Schema, Rows, and Columns
- Creating DataFrames from Various Data Sources (CSV, JSON, Parquet, etc.)
- Basic DataFrame Operations: Select, Filter, GroupBy, etc.
- Advanced DataFrame Operations
- Joins, Aggregations, and Window Functions
- Handling Missing Data and Data Cleaning in PySpark
- Optimizing DataFrame Operations
- Introduction to SparkSQL
- Basics of SparkSQL: Running SQL Queries on DataFrames
- Using SQL and DataFrame API Together
- Creating and Managing Temporary Views and Global Views
- Data Sources and Formats
- Working with Different File Formats: Parquet, ORC, Avro, etc.
- Reading and Writing Data in Various Formats
- Data Partitioning and Bucketing
- Hands-on Session: Building a Data Pipeline
- Designing and Implementing a Data Ingestion Pipeline
- Performing Data Transformations and Aggregations
- Introduction to Spark Streaming
- Overview of Real-Time Data Processing
- Introduction to Spark Streaming: Architecture and Basics
- Advanced Spark Concepts and Optimization
- Understanding Spark Internals
- Spark Execution Model: Jobs, Stages, and Tasks
- DAG (Directed Acyclic Graph) and Catalyst Optimizer
- Understanding Shuffle Operations
- Performance Tuning and Optimization
- Introduction to Spark Configurations and Parameters
- Memory Management and Garbage Collection in Spark
- Techniques for Performance Tuning: Caching, Partitioning, and Broadcasting
- Working with Datasets
- Introduction to Spark Datasets: Type Safety and Performance
- Converting between RDDs, DataFrames, and Datasets
- Advanced SparkSQL
- Query Optimization Techniques in SparkSQL
- UDFs (User-Defined Functions) and UDAFs (User-Defined Aggregate Functions)
- Using SQL Functions in DataFrames
- Introduction to Spark MLlib
- Overview of Spark MLlib: Machine Learning with Spark
- Working with ML Pipelines: Transformers and Estimators
- Basic Machine Learning Algorithms: Linear Regression, Logistic Regression, etc.
- Hands-on Session: Machine Learning with Spark MLlib
- Implementing a Machine Learning Model in PySpark
- Hyperparameter Tuning and Model Evaluation
- Hands-on Exercises and Project Work
- Optimization Techniques in Practice
- Extending the Mini-Project with MLlib
- Real-Time Data Processing and Advanced Streaming
- Advanced Spark Streaming Concepts
- Structured Streaming: Continuous Processing Model
- Windowed Operations and Stateful Streaming
- Handling Late Data and Event Time Processing
- Integration with Kafka
- Introduction to Apache Kafka: Basics and Use Cases
- Integrating Spark with Kafka for Real-Time Data Ingestion
- Processing Streaming Data from Kafka in PySpark
- Fault Tolerance and Checkpointing
- Ensuring Fault Tolerance in Streaming Applications
- Implementing Checkpointing and State Management
- Handling Failures and Recovering Streaming Applications
- Spark Streaming in Production
- Best Practices for Deploying Spark Streaming Applications
- Monitoring and Troubleshooting Streaming Jobs
- Scaling Spark Streaming Applications
- Hands-on Session: Real-Time Data Processing Pipeline
- Designing and Implementing a Real-Time Data Pipeline
- Working with Streaming Data from Multiple Sources
- Capstone Project - Building an End-to-End Data Pipeline
- Project Introduction
- Overview of Capstone Project: End-to-End Big Data Pipeline
- Defining the Problem Statement and Data Sources
- Data Ingestion and Preprocessing
- Designing Data Ingestion Pipelines for Batch and Streaming Data
- Implementing Data Cleaning and Transformation Workflows
- Data Storage and Management
- Storing Processed Data in HDFS, Hive, or Other Data Stores
- Managing Data Partitions and Buckets for Performance
- Data Analytics and Machine Learning
- Performing Exploratory Data Analysis (EDA) on Processed Data
- Building and Deploying Machine Learning Models
- Real-Time Data Processing
- Implementing Real-Time Data Processing with Structured Streaming
- Integrating Streaming Data with Machine Learning Models
- Performance Tuning and Optimization
- Optimizing the Entire Data Pipeline for Performance
- Ensuring Scalability and Fault Tolerance
- Industry Use Cases and Career Preparation
- Industry Use Cases of Spark and PySpark
- Discussing Real-World Applications of Spark in Various Industries
- Case Studies on Big Data Analytics using Spark
- Interview Preparation and Resume Building
- Preparing for Technical Interviews on Spark and PySpark
- Building a Strong Resume with Big Data Skills
- Final Project Preparation
- Presenting the Capstone Project for Resume and Instructions help
After successfully completing the Apache Spark & PySpark Essentials for Data Engineering course, learners will receive a Course Completion Certificate from Uplatz, validating their expertise in distributed data processing and Python-based big data development.
This certification highlights your skills in high-demand technologies used in data engineering, ETL development, and real-time analytics, and prepares you for further certifications in Spark or cloud-based data engineering tracks.
Proficiency in Spark and PySpark is a must-have for modern data engineering roles. This course unlocks several career paths, including:
- Data Engineer
- Big Data Developer
- Spark Developer
- ETL Engineer
- Data Analyst (Big Data)
- Machine Learning Engineer (Spark MLlib)
Industries such as finance, retail, healthcare, tech, media, and telecom use Spark for large-scale data analysis, streaming, and batch processing—creating continuous demand for Spark-skilled professionals.
1. What is Apache Spark and how does it differ from Hadoop?
Apache Spark is a distributed data processing engine that offers in-memory computation for faster analytics, unlike Hadoop’s MapReduce, which relies on disk I/O.
2. What are RDDs in Spark?
RDDs (Resilient Distributed Datasets) are the fundamental data structure in Spark, representing immutable distributed collections of objects that can be processed in parallel.
3. What is PySpark?
PySpark is the Python API for Apache Spark, allowing users to write Spark applications using Python.
4. What is the difference between RDD and DataFrame?
RDD is low-level and gives full control, whereas DataFrame is a higher-level abstraction optimized for performance and is easier to use with SQL-like syntax.
5. How does Spark achieve fault tolerance?
Spark achieves fault tolerance through lineage information in RDDs, which allows it to recompute lost data without relying on data replication.
6. What is Spark SQL?
Spark SQL allows querying structured data using SQL-like commands. It integrates seamlessly with DataFrames and datasets.
7. How is data partitioned in Spark?
Spark partitions data based on keys, hash functions, or custom logic, allowing parallel processing across nodes in a cluster.
8. What is lazy evaluation in Spark?
Spark delays execution until an action is called. This optimization strategy allows Spark to build efficient execution plans.
9. How do you optimize a PySpark job?
Use techniques like broadcasting small datasets, caching/persisting data, tuning partition sizes, and minimizing data shuffles.
10. Can Spark handle streaming data?
Yes, using Spark Structured Streaming, Spark can process real-time data streams with fault tolerance and scalability.
1. What is Apache Spark used for?
Apache Spark is used for big data processing, real-time stream analysis, machine learning, and large-scale ETL operations.
2. Is prior Python knowledge required for this course?
Basic Python knowledge is helpful, especially for the PySpark section, but all PySpark concepts are taught from the ground up.
3. Who should take this course?
Aspiring data engineers, big data developers, Python programmers, and analysts looking to handle large-scale data efficiently.
4. Is this course beginner-friendly?
Yes, it starts with Spark fundamentals and gradually moves to advanced data engineering use cases.
5. What learning format is used?
Self-paced video lessons with examples, hands-on labs, and guided projects you can complete at your convenience.
6. Will I receive a certificate?
Yes, upon course completion, you will receive a Course Completion Certificate from Uplatz.