Artificial Intelligence, Data Science, and Machine Learning with Python
Master AI, Data Science & Machine Learning with Python – From Fundamentals to Advanced Projects
95% Started a new career BUY THIS COURSE (
USD 12 USD 41 )-
89% Got a pay increase and promotion
Students also bought -
-
- Data Science with R
- 25 Hours
- USD 12
- 479 Learners
-
- Power BI (basic to advanced)
- 30 Hours
- USD 12
- 186 Learners
-
- Discount Offer - Any 10 Video Courses with Lifetime Access
- 250 Hours
- USD 112
- 210 Learners
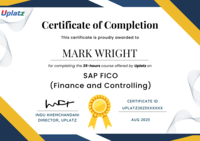
Artificial Intelligence, Data Science & Machine Learning with Python | Self-Paced Online Course
Accelerate your career in the most in-demand fields of Artificial Intelligence (AI), Data Science, and Machine Learning (ML) through this in-depth, self-paced course built entirely around the power and versatility of Python. Featuring pre-recorded video tutorials, hands-on coding exercises, and real-world projects, this course gives you the skills to analyze data, build machine learning models, and develop intelligent applications.
Python is the leading programming language in data science and AI, thanks to its rich ecosystem of libraries like NumPy, Pandas, Scikit-learn, TensorFlow, and PyTorch. This course offers step-by-step guidance from data processing and visualization to implementing supervised and unsupervised learning algorithms, natural language processing (NLP), deep learning, and AI-powered solutions.
Whether you're an aspiring data scientist, AI engineer, or ML developer, this course delivers the practical foundation and tools required to break into the tech industry's most exciting domains.
By the end of this course, learners will be able to:
- Understand the fundamentals of AI, data science, and machine learning.
- Work with Python libraries such as NumPy, Pandas, Matplotlib, Seaborn, Scikit-learn, TensorFlow, and Keras.
- Perform data preprocessing, data cleaning, and exploratory data analysis (EDA).
- Build and evaluate machine learning models including regression, classification, and clustering.
- Implement deep learning models using neural networks and TensorFlow/Keras.
Apply Natural Language Processing (NLP) for text classification, sentiment analysis, and chatbot development. - Develop AI projects such as image recognition, recommendation systems, and prediction engines.
- Work on real-world datasets from healthcare, finance, e-commerce, and more.
- Understand model deployment, API creation with Flask, and model performance monitoring.
- Prepare for roles in data science, AI development, and machine learning engineering.
Course Curriculum: Artificial Intelligence, Data Science & Machine Learning with Python
Master the essential skills in AI, Data Science, and Machine Learning with hands-on Python training. This comprehensive course covers everything from Python programming to advanced ML algorithms, ensuring you gain practical expertise for real-world applications.
Course Modules
1. Introduction to AI, Data Science & Python Setup
- Overview of AI and Data Science
- Key components and industry applications
- Setting up Python with Anaconda, Jupyter Notebook, and Spyder IDE
2. Python Programming Fundamentals
- Variables, operators, and data structures (lists, tuples, dictionaries)
- Control flow (loops, conditionals, functions)
- Lambda functions, recursion, and error handling
3. Data Importing & Cleaning
- Importing data from CSV, Excel, SQL, APIs, and web scraping
- Handling missing values, outliers, and inconsistencies
- Data manipulation with NumPy & Pandas
4. Exploratory Data Analysis (EDA) & Statistics
- Descriptive statistics (mean, median, variance, skewness)
- Univariate, bivariate, and multivariate analysis
- Probability distributions (Normal, Binomial, Poisson)
5. Data Visualization
- Creating plots with Matplotlib & Seaborn (histograms, scatter plots, box plots)
- Interactive visualizations with Plotly
- Storytelling with data
6. Statistical Inference & Hypothesis Testing
- Confidence intervals, p-values, and Z/T-tests
- ANOVA, Chi-square tests, correlation analysis
- Bayesian probability & sampling techniques
7. Machine Learning & Predictive Modeling
Supervised Learning:
- Regression (Linear, Polynomial, Logistic)
- Classification (Decision Trees, SVM, KNN, Naive Bayes)
Unsupervised Learning:
- Clustering (K-Means, Hierarchical, DBSCAN)
- Dimensionality Reduction (PCA, LDA)
- Ensemble Methods (Random Forest, Boosting, Bagging)
8. Capstone Project
- End-to-end real-world project applying all concepts
- Problem-solving with AI/ML pipelines
- Model deployment insights
Upon successfully completing the Artificial Intelligence, Data Science, and Machine Learning with Python course, learners will receive a Course Completion Certificate from Uplatz, validating their expertise in building AI and ML models using Python.
This certificate strengthens your professional profile and supports career growth in data science, AI engineering, machine learning, and advanced analytics.
With the demand for AI and data science professionals growing rapidly, completing this course will position you for roles in top organizations across industries like finance, healthcare, e-commerce, and technology. Career paths include:
- Data Scientist
- Machine Learning Engineer
- AI Developer
- Data Analyst
- Python Developer
- Deep Learning Specialist
- Business Intelligence Analyst
Professionals with strong Python-based AI and data skills are essential to companies aiming to build smart, data-driven solutions.
1. What is the difference between AI, machine learning, and data science?
AI focuses on creating intelligent systems, ML is a subset that involves algorithms learning from data, and data science includes data analysis, visualization, and model building.
2. Why is Python used in AI and data science?
Python offers simplicity, readability, and powerful libraries like Pandas, NumPy, and TensorFlow, making it ideal for AI, ML, and data analysis tasks.
3. What is supervised vs. unsupervised learning?
Supervised learning uses labeled data to train models (e.g., regression, classification), while unsupervised learning finds hidden patterns in unlabeled data (e.g., clustering).
4. What is overfitting and how can it be prevented?Overfitting occurs when a model performs well on training data but poorly on new data. It can be reduced using cross-validation, regularization, or pruning.
5. What is a confusion matrix?
It’s a table that evaluates classification models by showing the true vs. predicted values across categories, helping measure accuracy, precision, recall, and F1 score.
6. How does a neural network work?
Neural networks simulate the human brain, with layers of nodes (neurons) connected by weights that adjust during training to recognize complex patterns.
7. What is TensorFlow used for?
TensorFlow is an open-source framework used to build and deploy deep learning and machine learning models efficiently.
8. What are the steps in a data science project?
Data collection, cleaning, exploration, feature engineering, model building, evaluation, and deployment.
9. What is cross-validation?
A technique for assessing model performance by dividing the data into multiple training and testing sets to ensure generalization.
10. How do you deploy a machine learning model?
You can deploy using Flask or FastAPI to expose the model via an API, or use cloud platforms like AWS SageMaker, Azure ML, or GCP AI Platform.
1. Is this course beginner-friendly?
Yes! The course starts with the basics of Python, data science, and ML, making it suitable for beginners and professionals alike.
2. Do I need prior programming experience?
Basic familiarity with programming is helpful, but all essential Python concepts are taught as part of the course.
3. What tools and libraries will I learn?
Python, Jupyter Notebook, Pandas, NumPy, Matplotlib, Seaborn, Scikit-learn, TensorFlow, Keras, and Flask.
4. Is the course project-based?
Yes, learners will work on real-world projects and datasets throughout the course.
5. Will I get a certificate?
Absolutely! You’ll receive a Course Completion Certificate from Uplatz upon finishing all modules and assessments.