Career Path - Data Science Manager
Mastering Leadership in Data Science: Confluence of technical expertise & strategic vision. Develop skills & acumen to implement data science projectsPreview Career Path - Data Science Manager course
Price Match Guarantee Full Lifetime Access Access on any Device Technical Support Secure Checkout   Course Completion Certificate94% Started a new career BUY THIS COURSE (
USD 45 USD 139 )-
98% Got a pay increase and promotion
Students also bought -
-
- Premium Career Track - Chief Data Officer (CDO)
- 600 Hours
- USD 55
- 4405 Learners
-
- Bundle Course - BI Tools (Tableau - Power BI - SAP BO)
- 75 Hours
- USD 27
- 954 Learners
-
- Microsoft Azure Fundamentals
- 40 Hours
- USD 17
- 3673 Learners
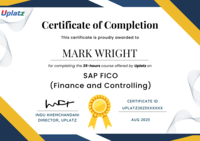
Courses included in the Data Science Manager Career Path Program are the following:
8) Data Visualization with Python
9) Data Visualization with R
14) Introduction to Cloud Computing
Responsibilities of a Data Science Manager:
- Strategic Planning: Define data science strategy aligned with business objectives, prioritize projects, and manage resources effectively.
- Team Leadership: Recruit, hire, mentor, and coach data scientists, fostering a collaborative and productive team environment.
- Communication & Collaboration: Bridge the gap between technical and business teams, translating complex data science concepts into understandable language for stakeholders.
- Project Management: Plan, monitor, and manage data science projects, ensuring they meet deadlines, budget constraints, and quality standards.
- Technical Expertise: While not necessarily coding experts, they need a solid understanding of data science principles, methods, and tools to assess feasibility, guide team efforts, and communicate effectively with the team.
- Problem-solving & Analytical Skills: Identify and solve complex business problems using data-driven approaches, interpreting results and drawing actionable insights.
- Business Acumen: Understand the organization's industry, competitors, and market trends to ensure data science initiatives drive genuine business value.
- Communication & Interpersonal Skills: Clearly communicate data insights and project progress to stakeholders at all levels, fostering trust and buy-in.
Required Skills of a Data Science Manager:
- Technical Skills - Programming languages (Python, R, etc.), Statistical analysis and modeling, Machine learning algorithms, Data visualization and communication tools, Cloud computing platforms
- Soft Skills - Leadership and team management, Communication and interpersonal skills, Strategic thinking and problem-solving, Project management and organizational skills, Business acumen and domain knowledge, Analytical and critical thinking
- Bonus Skills - Experience in a specific industry, Knowledge of ethical considerations in data science, Strong written and verbal communication skills
Course/Topic 1 - Python Programming - all lectures
-
In this lecture session we learn about introduction to python programming for beginners and also talk about features of python programming.
-
In this lecture session we learn about basic elements of python in python programming and also talk about features of elements of python.
-
In this lecture session we learn about installation of python in your system and also talk about the best way of installation of python for beginners.
-
In this lecture session we learn about input and output statements in python programming and also talk about features of input and output statements.
-
In this lecture session we learn about data types in python programming and also talk about all the data types in python programming.
-
In this lecture session we learn about operators in python and also talk about how we use operators in python programming.
-
In this lecture session we learn about different types of operators in python programming and also talk about features of operators in python.
-
In this lecture session we learn about type conversion in python programming and also talk about features of type conversion in python.
-
In this lecture session we learn about basic programming in python programming for beginners.
-
In this lecture session we learn about features of basic programming in python and also talk about the importance of programming in python.
-
In this lecture session we learn about math modules in python programming and also talk about features of math modules in python.
-
In this lecture session we learn about conditional statements in python and also talk about conditional statements in python programming.
-
In this lecture session we talk about basic examples of conditional statements in python.
-
In this lecture session we learn about greater and less then conditional statements in python programming.
-
In this lecture session we learn about nested IF Else statements and also talk about features of nested IF else statements.
-
In this lecture session we learn about looping in python in programming for beginners and also talk about looping in python.
-
In this lecture session we learn about break and continue keywords and also talk about features of break continue keywords.
-
In this lecture session we learn about prime number programs in python and also talk about functions of prime number programs in python.
-
In this lecture session we learn about while loop in python programming and also talk about features of while loop in python.
-
In this lecture session we learn about nested For loop in python programming and also talk about features of nested For loop.
-
In this lecture session we learn about features of nested for loop in python and also talk about the importance of nested For loop in python.
-
In this lecture session we learn about functions in python and also talk about different types of functions in pythons.
-
In this lecture session we learn about passing arguments to functions in python programming and also talk about features of passing arguments to functions
-
In this lecture session we learn about return keywords in python and also talk about features of return keywords in python.
-
In this lecture session we learn about calling a function in python programming and also talk about calling a function.
-
In this lecture session we learn about factors of calling a function in python programming and also talk about features of calling a function.
-
In this lecture session we learn about a program to swap 2 numbers using calling a function in python programming.
-
In this lecture session we learn about functions of arbitrary arguments in python programming and also talk about features of arbitrary arguments.
-
In this lecture session we learn about functions keywords arguments in python programming and also talk about features of keyword arguments.
-
In this lecture session we learn about functions default arguments in python programming and also talk about features of default argument.
-
In this lecture session we learn about global and local variables in python programming and also talk about features of global and local variables.
-
In this lecture session we learn about global and local keywords and also talk about features of global and local keywords.
-
In this lecture session we learn about strings in python programming and also talk about features of string in python.
-
In this lecture session we learn about string methods in python programming and also talk about features of string methods in python.
-
In this lecture session we learn about string functions in python and also talk about features of strings functions in python.
-
In this lecture session we learn about string indexing in python programming and also talk about features of string indexing in python programming.
-
In this lecture session we learn about introduction of lists in python programming and also talk about features of introduction to lists.
-
In this lecture session we learn about basics of lists python programming and also talk about features of basics of lists in python.
-
In this lecture session we learn about list methods and also talk about features of list method python programming.
-
In this lecture session we learn about linear search on list and also talk about features of linear search on list in brief.
-
In this lecture session we learn about the biggest and smallest number of the list and also talk about features of MAX and Min in a list.
-
In this lecture session we learn about the difference between 2 lists in python programming and also talk about features of 2 lists.
-
In this lecture session we learn about tuples in python programming and also talk about tuples in python programming.
-
In this lecture session we learn about introduction to sets in python and also talk about functions of introduction to sets in python.
-
In this lecture session we learn about set operations in python programming and also talk about features of set operation in brief.
-
In this lecture session we learn about set examples and also talk about features set examples.
-
In this lecture session we learn about introduction to dictionaries in python programming and also talk about featured dictionaries.
-
In this lecture session we learn about creating and updating dictionaries in python programming and also talk about features of creating and updating dictionaries.
-
In this lecture session we learn about deleting items in a dictionary in python programming and also talk about features of deleting items in a dictionary.
-
In this lecture session we learn about values and items in a dictionary in python programming and also talk about features of values and items in the dictionary.
-
In this lecture session we learn about dictionary methods in python programming and also talk about features of dictionary methods.
-
In this lecture session we learn about built in methods in python programming and also talk about features of built in methods in python.
-
In this lecture session we learn about lambda functions and also talk about features of lambda function in python programming.
-
In this lecture session we learn about file handling in python programming and also also talk about the importance of file handling in python.
-
In this lecture session we learn about file handling in python programming and also talk about features of file handling in python.
-
In this lecture session we learn about exception handling in python and also talk about features of exception handling in python.
-
In this lecture session we learn about exception handling examples in python programming.
-
In this lecture session we learn about python programs in python programming and also talk about features of python programs
-
In this lecture session we learn about the program of printing odd numbers in python programming and also talk about the best way of printing.
-
In this lecture session we learn about counting the number of vowels and consonants in a string and also talk about features of these programs.
-
In this lecture session we learn about python programs of swapping two numbers in a list by taking indexes as parameters.
-
In this lecture session we learn about bubble sort and also talk about features of bubble sort in brief.
-
In this lecture session we learn about operator precedence in python and also talk about features of operator precedence in python.
-
In this lecture session we learn about operator precedence in python and also talk about features of operator precedence types.
-
In this lecture session we learn about recursion in python and also talk about features of recursion in python.
-
In this lecture session we learn about binary search in python and also talk about features of binary search in python programming.
-
In this lecture session we learn about binary search in python and also talk about the importance of binary search in python.
-
In this lecture session we learn about object oriented programming and also talk about features of object oriented programming in brief.
-
In this lecture session we learn about factors and types of object oriented programming in python programming.
-
In this lecture session we learn about OOPS and procedural programming and also talk about features of OOPS and procedural programming in OOPS.
-
In this lecture session we learn about OOPS programs in python and also talk about the importance of OOPS.
-
In this lecture session we learn about inheritance in python programming and also talk about features of inheritance.
-
In these lecture sessions we learn about features of object creation in python programming and also talk about object creation in python.
-
In this lecture session we learn about OOPS terminology and functions and also talk about features of OOPS terminology and functions.
-
In this lecture session we learn about built in class attributes and garbage collection in python programming.
-
In this lecture session we learn about inheritance in python and also talk about features of inheritance in python.
-
In this lecture session we learn about the importance of inheritance in python programming and also talk about functions of inheritance.
-
In this lecture session we learn about programs in inheritance in python programming and also talk about features of inheritance in python.
-
In this lecture session we learn about polymorphism in python programming polymorphism and also talk about polymorphism in python.
-
In this lecture session we learn about features of polymorphism in python and also talk about the importance of polymorphism in python.
-
In this lecture session we learn about the time module in python and also talk about features time module in python in features.
-
In this lecture session we learn about the importance of time modules in python time module in python in brief.
-
In this lecture session we learn about the calendar module in python programming in brief.
-
In these lecture sessions we learn about calendar methods in python programming and also talk about the importance of calendar methods.
-
Class 28.1 - Boolean in Python
-
In this lecture session we learn about python iterators and also talk about features of python iterators in brief.
-
In this lecture session we learn about python programs and summary in python programming and also talk about python programs.
-
In this lecture sessions we learn about python programs and also talk about features of python programs and summary.
Course/Topic 2 - Python Programming (basic to advanced) - all lectures
-
This video comprehends the terms Python which is to develop by Guido van Rossum. Guido van Rossum started implementing Python in 1989. Python is a very simple programming language so even if you are new to programming, you can learn python without facing any issues.
-
This topic will cover, Installing Python which is generally easy, and nowadays many Linux and UNIX distributions include a recent Python. Even some Windows computers now come with Python already installed.
-
In this Python tutorial, we will learn about Python variables and data types which is being used in Python. We will also learn about converting one data type to another in Python and local and global variables in Python. So, let’s begin with Python variables and data types Tutorial.
-
In this topic you will learn about the data type which is an important concept. Variables can store data of different types, and different types can do different things.
-
This session will teach you about the Python defines type conversion functions to directly convert one data type to another which is useful in day to day and competitive programming. This article is aimed at providing information about certain conversion functions.
-
In this tutorial, you will learn about the keywords which is the reserved words in Python and identifiers names given to variables, functions, etc. We cannot use a keyword as a variable name, function name or any other identifier. They are used to define the syntax and structure of the Python language.
-
In this tutorial, we are going to learn how to take multiple inputs from the user in Python. The data entered by the user will be in the string format. So, we can use the split method to divide the user entered data.
-
This tutorial focuses on two built in functions print and input to perform Input and Output task in Python. Also, you will learn to import modules and use them in your program. Some of the functions like input and print are widely used for standard input and output operations respectively. Let us see the output section first.
-
This tutorial covers the different types of operators in Python, operator overloading, precedence and associativity. Just like in mathematics, programming languages like Python have operators. You can think of them as extremely simple functions that lie at the basis of computer science.
-
In this tutorial, you'll learn everything about different types of operators in Python, their syntax and how to use them with examples. Operators are special symbols in Python that carry out arithmetic or the logical computation. The value that the operator operates on is called the operand.
-
Previously, in our tutorial on Python Operators., Today, in this Python Bitwise Operators Tutorial, we will discuss Python Bitwise AND, OR, XOR, Left-shift, Right-shift, and 1’s complement Bitwise Operators in Python Programming. Along with this, we will discuss syntax and example of Python Bitwise Operators.
-
Today, we talk about Python decision making constructs. This includes Python if statements, if else statements, elif statement, nested if conditions and single statement conditions. We will understand these with syntax and example to get a clear understanding. So, let’s start the Python Decision Making Tutorial.
-
In this session you will learn about the if elif else which are conditional statements that provide you with the decision making that is required when you want to execute code based on a particular condition. The if elif else statement used in Python helps automate that decision making process.
-
In this session, you'll learn the different variations of for loop, for loop is used for iterating over a sequence that is either a list, a tuple, a dictionary, a set, or a string. This is less like the for keyword in other programming languages, and works more like an iterator method as found in other object-orientated programming languages.
-
In this session, you will learn to create a while loop in Python. Loops are used in programming to repeat a specific block of code. In this article, you will learn to create a while loop in Python. Loops are used in programming to repeat a specific block of code.
-
In this session, we show how to create an infinite loop in Python. An infinite loop that never ends it never breaks out of the loop. So, whatever is in the loop gets executed forever, unless the program is terminated. For certain situations, an infinite loop may be necessary.
-
In this video, you will learn how to make the computer execute a group of statements over and over if certain criterion holds. The group of statements being executed repeatedly is called a loop.
-
In this session, you'll learn about the different numbers used in Python, how to convert from one data type to the other, and the mathematical operations supported in Python. Python supports integers, floats and complex numbers.
-
In the tutorial on strings in Python, you learned how to define strings objects that contain sequences of character data. Processing character data is integral to programming. It is a rare application that doesn’t need to manipulate strings at least to some extent.
-
As discussed in the above tutorial, strings in Python are immutable and thus updating or deleting an individual character in a string is not allowed, which means that changing a particular character in a string is not supported in Python. Although, the whole string can be updated and deleted.
-
In this session, we'll learn everything about Python lists, how they are created, slicing of a list, adding or removing elements from them and so on. The list is a most versatile datatype available in Python which can be written as a list of comma-separated values items between square brackets. Important thing about a list is that items in a list need not be of the same type.
-
In this tutorial, learn how to update list element using Python. Use the index position and assign the new element to change any element of List. You can change the element of the list or item of the list with the methods given here.
-
In that tutorial of Python Functions, we discussed user-defined functions in Python. But that isn’t all, a list of Python built-in functions that we can toy around with. In this tutorial on Built-in functions in Python, we will see each of those, we have 67 of those in Python 3.6 with their Python Syntax and examples.
-
In this tutorial, you'll learn everything about Python tuples. More specifically, what are tuples, how to create them, when to use them and various methods you should be familiar with. A tuple in Python is similar to a list. The difference between the two is that we cannot change the elements of a tuple once it is assigned whereas we can change the elements of a list.
-
This session teaches you the tuple in Python which are immutable sequences, you cannot update them. You cannot add, change, remove items (elements) in tuples.Tuple represent data that you don't need to update, so you should use list rather than tuple if you need to update it. However, if you really need to update tuple, you can convert it to list, update it, and then turn it back into tuple.
-
In this tutorial, you'll learn everything about Python dictionaries how they are created, accessing, adding, removing elements from them and various built in methods. Python dictionary is an unordered collection of items. Each item of a dictionary has a pair. Dictionaries are optimized to retrieve values when the key is known.
-
In this session we will teach you the dictionary which is a data type similar to arrays, but works with keys and values instead of indexes. Each value stored in a dictionary can be accessed using a key, which is any type of object a string, a number, a list, etc. instead of using its index to address it.
-
In this session we will teach you the Python for beginners training course which is a lead the students from the basics of writing and running Python scripts to more advanced features such as file operations, regular expressions, working with binary data, and using the extensive functionality of Python modules. Extra emphasis is placed on features unique to Python, such as tuples, array slices, and output formatting.
-
In this video, you will learn to manipulate date and time in Python with the help of examples. Python has a module named datetime to work with dates and times. Let's create a few simple programs related to date and time before we dig deeper.
-
In this session, you'll learn about functions, what a function is, the syntax, components, and types of functions. Also, you'll learn to create a function in Python. In Python, a function is a group of related statements that performs a specific task. Functions help break our program into smaller and modular chunks.
-
In this video we will learn, the function which use the same variable and object. Pass by Value. In pass by value the function is provided with a copy of the argument object passed to it by the caller. That means the original object stays intact and all changes made are to a copy of the same and stored at different memory locations.
-
In this tutorial, you'll learn about the anonymous function, also known as lambda functions. You'll learn what they are, their syntax and how to use them with examples.
-
In this tutorial we will teach you the module which is a piece of software that has a specific functionality. Like, when building a ping pong game, one module would be responsible for the game logic, and another module would be responsible for drawing the game on the screen. Each module is a different file, which can be edited separately.
-
This session teaches you the Python rename method which is used to rename a file or directory. This method is a part of the python module and comes extremely handy.
-
In this tutorial, you'll learn about Python file operations. More specifically, opening a file, reading from it, writing into it, closing it, and various file methods that you should be aware of.
-
In this tutorial we will learn about program for files in Python which provides us with an important feature for reading data from the file and writing data into a file. Mostly, in programming languages, all the values or data are stored in some variables which are volatile in nature.
-
In this session we will tell you the method that you the current position within the file; in other words, the next read or write will occur at that many bytes from the beginning of the file. The seek method changes the current file position.
-
In this tutorial, you'll learn how to handle exceptions in your Python program using try, except and finally statements with the help of examples. Python has many built-in exceptions that are raised when your program encounters an error (something in the program goes wrong).
-
In this tutorial, you will learn about different types of errors and exceptions that are built-in to Python. They are raised whenever the Python interpreter encounters errors.
-
In this video we will teach you about the Exception handling in Python which is very similar to Java. The code, which harbors the risk of an exception, is embedded in a try block.
-
In this tutorial, you will learn about the core functionality of Python objects and classes. You'll learn what a class is, how to create it and use it in your program.
-
In this session you will learn about the programming in Python (object-oriented programming) for some time, then you have definitely come across methods that have self as their first parameter. Let us first try to understand what this recurring self-parameter is.
-
This tutorial teaches you about the regular expression which is a special sequence of characters that helps you match or find other strings or sets of strings, using a specialized syntax held in a pattern. Regular expressions are widely used in UNIX world.
-
In this tutorial we will learn about the python search which is a method of the module That is Syntax of search () re. search (pattern, string). It is similar to re. match () but it doesn’t limit us to find matches at the beginning of the string only. Unlike in re. match () method, here searching for pattern ‘Tutorials’ in the string ‘TP Tutorials Point TP’ will return a match.
-
This workshop will introduce GUI programming in Python, it is a is a popular language for elementary programming but it not so easy to write programs with a graphical user interface (GUI).
-
In this tutorial, we will learn how to develop graphical user interfaces by writing some Python GUI examples using the Tkinter package. Tkinter package is shipped with Python as a standard package, so we don’t need to install anything to use it.
-
This session teaches you about the frame widgets which is a rectangular region on the screen. The frame widget is mainly used as a geometry master for other widgets, or to provide padding between other widgets.
Course/Topic 3 - R Programming - all lectures
-
In this lecture session we learn about basic introduction of R programming for beginners and also talk about basic functions of R programming for beginners.
-
In this tutorial we learn about how we install r programming in our software and also talk about the best way of installing R programming for beginners.
-
In this lecture session we learn about R's basic data structures including the vector, list, matrix, data frame, and factors. Some of these structures require that all members be of the same data type (e.g. vectors, matrices) while others permit multiple data types (e.g. lists, data frames). Objects may have attributes, such as name, dimension, and class.
-
In this lecture session we learn about A vector is the basic data structure in R, or we can say vectors are the most basic R data objects.
-
In this lecture session we learn about R is an ideal tool when it comes to data wrangling. It allows the usage of several preprocessed packages that makes data wrangling a lot more easier. This is one of the main reasons as to why R is preferred in the Data Science community.
-
In this lecture session we learn about R packages are a collection of R functions, compiled code and sample data. They are stored under a directory called "library" in the R environment. By default, R installs a set of packages during installation. More packages are added later, when they are needed for some specific purpose.
-
In this tutorial we learn about R is an open-source programming language that is widely used as a statistical software and data analysis tool.
-
In this lecture session we learn that R can be used as a powerful calculator by entering equations directly at the prompt in the command console. Simply type your arithmetic expression and press ENTER. R will evaluate the expressions and respond with the result.
-
In this tutorial we learn about Conditional statements are those statements where a hypothesis is followed by a conclusion. It is also known as an " If-then" statement.
-
In this tutorial we learn about In coding, you ask your computer to check conditions by writing conditional statements. Conditional statements are the way computers can make decisions.
-
In this lecture session we learn about It is a type of control statement that enables one to easily construct a loop that has to run statements or a set of statements multiple times. For loop is commonly used to iterate over items of a sequence.
-
In this lecture session we learn about Repeat loop, unlike other loops, doesn't use a condition to exit the loop instead it looks for a break statement that executes if a condition within the loop body results to be true.
-
In this lecture session we learn that Sum of n natural numbers can be defined as a form of arithmetic progression where the sum of n terms are arranged in a sequence with the first term being.
-
In this lecture session we learn about The formula to find the sum of n terms in AP is Sn = n/2 (2a+(n−1)d), in which a = first term, n = number of terms, and d = common difference between consecutive terms.
-
In this tutorial we learn about A switch statement that allows a variable to be tested for equality against a list of values. Each value is called a case, and the variable being switched on is checked for each case.
-
In this lecture session we learn about Data preprocessing, a component of data preparation, describing any type of processing performed on raw data to prepare it for another data processing procedure.
-
In this tutorial we learn about Data preprocessing is essential before its actual use. Data preprocessing is the concept of changing the raw data into a clean data set. The dataset is preprocessed in order to check missing values, noisy data, and other inconsistencies before executing it to the algorithm.
-
In this lecture session we learn about Factor in R is a variable used to categorize and store the data, having a limited number of different values. It stores the data as a vector of integer values. Factor in R is also known as a categorical variable that stores both string and integer data values as levels.
-
In this tutorial we learn about A data frame is a table or a two-dimensional array-like structure in which each column contains values of one variable and each row contains one set of values from each column.
-
In this tutorial we learn about In R we use merge() function to merge two dataframes in R. This function is present inside the join() function of the dplyr package.
-
In this lecture session we learn about The R merge function allows merging two data frames by common columns or by row names. This function allows you to perform different database (SQL) joins, like left join, inner join, right join or full join, among others.
-
In this tutorial we learn about The two data frames must have the same variables, but they do not have to be in the same order.
-
In this lecture session we learn about merge is a generic function whose principal method is for data frames: the default method coerces its arguments to data frames and calls the "data. frame" method. By default the data frames are merged on the columns with names they both have, but separate specifications of the columns can be given by.
-
In this lecture session we learn about The functions which are already created or defined in the programming framework are known as a built-in function. R has a rich set of functions that can be used to perform almost every task for the user.
-
In this lecture session we learn about Melting and Casting are one of the interesting aspects in R programming to change the shape of the data and further, getting the desired shape.
Course/Topic 4 - R Programming (basic to advanced) - all lectures
-
In this lecture session we learn about basic introduction to R programming and also talk about some key features of R programming.
-
In this lecture session we learn about the setup of R language in your system and also talk about the importance of R programming.
-
In this lecture session we learn about variables and data types in R language and also talk about types of variables and data types in R programming.
-
In this lecture session we learn about uses of variable and data types in our programs and also talk about some key features of variables and data types.
-
In this lecture session we learn about input - output features and also talk about features of input - output features.
-
In this lecture session we learn about posted function () in input output features and also talk about features of posted functions().
-
In this lecture session we learn about operators in R and also talk about features of operators in R programming.
-
In this lecture session we learn about different types of operators in R language and also talk about features of all types of operators in R.
-
In this lecture session we learn about vectors in data structure in R programming and also talk about features of vectors in data structures in brief.
-
In this lecture session we learn about the importance of vectors in data structure and also talk about vectors in data structures in brief.
-
In this lecture session we learn about list data structure in R programming and also talk about features of list in data structure.
-
In this lecture session we learn about more operations on the list and also talk about features of List in data structures in brief.
-
In this lecture session we learn about matrix in R programming and also talk about features of matrix in data structure in R language.
-
In this lecture session we learn about matrix in R programming and also talk about features of matrix in data structure in R language.
-
In this lecture session we learn about matrix data structure in R programming and also talk about some key features of matrix and data structure.
-
In this lecture session we learn about arrays in R programming and also talk about features of arrays.
-
In this lecture session we learn about different types of arrays in data structure and also talk about features of Arrays in data structure in brief.
-
In this lecture session we learn about data frame in R programming and also talk about function of data frame.
-
In this lecture session we learn about data frame features in R programming and also talk about the importance of data structure.
-
In this lecture session we learn about the importance of Data frame in brief and also talk about function of data frame in R programming.
-
In this lecture session we learn about data frame key features of data frame in data structure in brief.
-
In this lecture session we learn about factors data structures in R programming and also talk about the importance of factors.
-
In this lecture session we learn about factors of data structure in R programming and also talk about different types of factors in R language.
-
In this lecture session we learn about decision making in R programming and also talk about features of decision making in R.
-
In this lecture session we learn about different types of decision making statements and also talk about features of all decision statements.
-
In this lecture session we learn about decision making using integers and also talk about functions of integers.
-
In this lecture session we learn about Loops in R programming and also talk about factors of Loops in R language.
-
In this lecture session we learn about functions of Loops and why we need Loop statement in R programming and also talk about key features of Loop statement.
-
In this lecture session we learn about different types of Loops in R programming and also talk about features of For loop, while loop and do while loop.
-
In this lecture session we learn about functions in R programming language and also talk about features of functions in R language.
-
In this lecture session we learn about different types of functions in R programming and also talk about the importance of functions.
-
In this lecture session we learn about string in R programming and also talk about features of string function in R.
-
In this lecture session we learn about why we need strings in R programming and also talk about the importance of strings.
-
In this lecture session we learn about packages in R programming and also talk about features of packages in R.
-
In this lecture session we learn about data and file management in R programming and also talk about functions of data and file management.
-
In this lecture session we learn about how we manage the data and file in R programming and also talk about the importance of data and file management.
-
In this lecture session we learn about Line chart in R programming and also talk about features of line chart in brief.
-
In this lecture session we learn about scatterplot in R language and also talk about functions of scatters plot.
-
In this lecture session we learn about Pie chart in R programming and also talk about features of Pie Chart in brief.
-
In this lecture session we learn about bar charts in R language and also talk about features of Bar chart in brief.
-
In this lecture session we learn about how we use bar charts in R programming and also talk about features of Bar charts.
-
In this lecture session we learn about histogram in R programming and also talk about features of histogram.
-
In this lecture session we learn about Boxplots in R programming and also talk about features of Boxplot in R language.
Course/Topic 5 - SQL Programming with MySQL Database - all lectures
-
In this video get an in-depth introduction to the terminology, concepts, and skills you need to understand database objects, administration, security, and management tools. Plus, explore T-SQL scripts, database queries, and data types
-
In this video learn basic of SQL Programming and overview the SQL basic commands and how we use these commands in SQL Programming. This SQL tutorial will teach you basics on how to use SQL in MySQL, SQL Server, MS Access, Oracle, Sybase, Informix, Postgres, and other database systems.
-
In this video we talk about DDL (DATA DEFINATION LANGUAGE) and also cover all the basic techniques of DDL.In this video we will learn about the SQL commands – DDL, DML, DCL; SQL Constraints – Keys, Not Null, Check , Default, and also MYSQL Hands-on and basic Querying
-
In this video session we learn SQL commands and how to use these commands like select command, insert command, delete command etc. In this video we will learn about hands-on experience on the terminal, creating database, Tables and manipulating data.
-
In this video we learn about SQL Basic and Aggregate Functions and also cover different functions of SQL. This tutorial teaches us about clauses and the update command. We will also cover making records, updating and modifying rows.
-
In this session we talk about SQL Regular Expression and we also cover all techniques of SQL Regular Expression.This tutorial teaches us about clauses and the update command. We will also cover making records, updating and modifying rows and EML commands.
-
In this video we learn about SQL Comparison Clauses and how we use Comparison Clauses in SQL. This tutorial covers Comparison Operators by relating values by a mathematical symbol which is used to compare two values. Learn about comparison operators result - TRUE, FALSE, or UNKNOWN
-
In this session we learn about SQL String and also cover all types of string in SQL and how we can use SQL Strings. In this video we will learn about the basic string functions such as concat_ws, file format, and insert function, L-case, u case, and lower case. We will also learn about basic functions such as upper functions.
-
In this session we cover advance level string function and also cover all different commands we use in SQL String Function. This video is a sequel for string functions tutorial. In this tutorial we will learn few most useful string functions such as spaces and null issue as well, L-Pad command.
-
In this SQL String function part 3 we learn select Repeat function and Select Replace function and also cover different between Select Repeat function and Select Replace function. This tutorial is another sequel to string functions, however, these functions are used less and not used that frequently. We will further learn here about the repeat function, absolute function, ceiling, and floor and down functions.
-
In this session we learn about SQL Numeric Functions and how we use Numeric functions in SQL. In this video, we will be covering numerical functions. Learn about the basic date functions and also about truncate functions.
-
In this video session we learn about SQL Numeric Function and also cover the basic functionality of SQL Numeric Function. SQL Data Functions. In this video we will learn about few more Date functions. We will further look into the day function option as well. This tutorial covers basic querying over a single table.
-
: In this video we talk about SQL Joins Introduction and Demonstration and basic join’s function and how to make table using joins. In this tutorial learn about joints in SQL. This tutorial teaches us how to connect two different tables with joints. We will also cover the topic of querying two or more tables and about subquery .
-
In this lecture last session we talk about MySQL Workbench and procedures and Views and MySQL Workbench functionality. In this tutorial learn about SQL in automating things. This tutorial covers stroll procedure, functions and views which are helpful for automation purposes in SQL.
Course/Topic 6 - Data Science with Python - all lectures
-
In this video tutorial we will get introduced to Data Science and the integration of Python in Data Science. Furthermore, we will look into the importance of Data Science and its demand and the application of Data Science.
-
In this video we will learn, all the concepts of Python programming related to Data Science. We will also learn about the Introduction to Python Programing, what is Python Programming and its History, Features and Application of Python along with its setup. Further we will see how to get started with the first python program.
-
This video talks about the Variable and Data Types in Python Programming. In this session we will learn What is variable, the declaration of variable and variable assignment. Further we will see the data types in python, checking data types and data type conversions.
-
This tutorial will help you to understand Data Types in python in depth. This video talks about the data types such as numbers, sequence type, Boolean, set and dictionary.
-
This tutorial talks about the Identifier, keyword, reading input and output formatting in Data Science. We will learn about what is an identifier and keywords. Further we will learn about reading input and taking multiple inputs from a user, Output formatting and Python end parameter.
-
This tutorial talks about taking multiple inputs from user and output formatting using format method, string method and module operator.
-
This tutorial talks about the Operators and type of operators. In this session we will learn about the types of operators such as arithmetic, Relational and Assignment Operators.
-
This tutorial talks further about the part 2 of operators and its types. In this session we will learn about the types of operators such as Logical, Membership, Identity and Bitwise Operators.
-
In this video you will learn about the process of decision making in Data Science. Furthermore, this tutorial talks about different types of decision-making statements and its application in Data Science.
-
In this video tutorial we will learn about the Loops in Python programing. We will cover further the different types of Loops in Python, starting with: For Loop.
-
In this session we will cover the further part of loops in Python programming. The type of loops explained in this video is: While loop and nested loop.
-
In this session we will cover the further part of loops in Python programming. The type of loops explained in this video is: break, continue and pass loops
-
In this video tutorial we will start explaining about the lists in Python Programming. This tutorial talks about accessing values in the list and updating the list in Data Science.
-
In this video tutorial we will look into the further parts about the lists in Python Programming. Deleting list elements, basic list operations, built in functions and methods and the features which are covered in this session.
-
This tutorial will cover the basics on Tuples and Dictionary function in Data Science. We will learn about accessing and deleting tuple elements. Further we will also cover the basic tuples operations and the built in tuple functions and its methods. At the end we will see the differences in list and tuple.
-
This tutorial will cover the advanced topics on Tuples and Dictionary function in Data Science. Further in this session we will learn about the Python Dictionary, how to access, update and delete dictionary elements. Lastly we will cover built in functions and methods.
-
In this session we will learn about the functions and modules used in Data science. After watching this video, you will be able to understand what is a function, the definition of function and calling a function.
-
In this session we will learn about the further functions and modules used in Data science. After watching this video, you will be able to understand the ways to write a function, Types of functions, Anonymous Functions and Recursive functions.
-
In this session we will learn about the advanced functions and modules used in Data science. After watching this video, you will be able to understand what is a module, creating a module, import statement and locating modules.
-
This tutorial talks about the features of working with files. In this video we will learn about opening and closing file, the open function, the file object Attributes, the close method, reading and writing files.
-
This tutorial talks about the advanced features of working with files. In this video we will learn about file positions, renaming and deleting files.
-
In this session we will learn about the regular expression. After this video you will be able to understand what is a regular expression, meta characters, match function, search function, Re- match vs research, split function and sub function.
-
This video introduces you to the Data Science Libraries. In this video you will learn about the Data science libraries: libraries for data processing, modelling and data visualization.
-
In this session we will teach about the components of python ecosystem in Data Science. This video talks about the Components of Python Ecosystem using package Python distribution Anaconda and jupyter notebook.
-
This tutorial talks about the basics of analyzing data using numpy and pandas. The Pandas module mainly works with the tabular data, whereas the NumPy module works with the numerical data. We will further see what is Numpy and why we use numpy.
-
This tutorial talks about the later part of analyzing data using numpy and pandas. In this tutorial we will learn how to install numpy.
-
This tutorial talks about the advanced part of analyzing data using numpy and pandas. In this session we will learn what is Pandas and the key features of Pandas. We will also learn about the Python Pandas environment setup.
-
This tutorial talks about the advanced part of analyzing data using numpy and pandas. In this session we will learn about Pandas data structure with example.
-
This the last session on Analysing Data using Numpy and Pandas. In this session we will learn data analysis using Pandas
-
In this video tutorial we will learn about the Data Visualization using Matpotlib. This video talks about what is data visualisation, introduction to matplotlib and installation of matplotlib.
-
In this session we will see the part 2 of Data Visualization with Matplotlib. This video talks about the types of data visualization charts and line chart scatter plot
-
This tutorial covers part 3 of Data Visualization with Matplotlib. This session covers the types of data visualisation charts: bar chart histogram, area plot pie chart and box plot contour plot.
-
This session talks about the Three-Dimensional Plotting with Matplotlib . In this we will learn about plot 3D scatter, plot 3D contour and plot 3D surface plot.
-
In this tutorial we will cover basics of Data Visualisation with Seaborn. Further we will cover Introduction to seaborn, seaborn functionalities, how to install seaborn and the different categories of plot in seaborn
-
In this tutorial we will cover the advanced topics of Data Visualisation with Seaborn. In this video we will see about exploring seaborn plots.
-
Introduction to Statistical Analysis is taught in this video. We will learn what is statistical analysis and introduction to math and statistics for data science. Further we will learn about the terminologies in statistics for data science and categories in statistics, its correlation and lastly mean median and mode quartile.
-
This video course talks about the basics of Data Science methodology. We will learn how to reach from problem to approach.
-
In this session we will see Data Science Methodology from requirements to collection and from understanding to preparation.
-
In this session we will learn advanced Data Science Methodology from modelling to evaluation and from deployment to feedback.
-
This video tutorial talks about the - Introduction to Machine Learning and its Types. In this session we will learn what is machine learning and the need for machine learning. Further we will see the application of machine learning and different types of machine learning. We will also cover topics such as supervised learning, unsupervised learning and reinforcement learning.
-
This video tutorial talks about the basics of regression analysis. We will cover in this video linear regression and implementing linear regression.
-
This video tutorial talks about the further topics of regression analysis. In this video we will learn about multiple linear regression and implementing multiple linear regression.
-
This video tutorial talks about the advanced topics of regression analysis. In this video we will learn about polynomial regression and implementing polynomial regression.
-
In this session we will learn about the classification in Data science. We will see what is classification, classification algorithms and Logistic regression. Also we will learn about implementing Logistic regression.
-
In this session we will learn about the further topics of classification in Data science, such as decision tree and implementing decision tree.
-
In this session we will learn about the advanced topics of classification in Data science, such as support vendor machine and implementing support vector machine.
-
This tutorial will teach you about what is clustering and clustering algorithms. Further we will learn what K means clustering and how does K means clustering work and also about implementing K means clustering.
-
In this session we will see the further topics of clustering, such as hierarchical clustering, agglomerative hierarchical clustering, how does agglomerative hierarchical clustering Work and divisive hierarchical clustering.
-
This video tutorial talks about the advanced topics of clustering, such as implementation of agglomerative hierarchical clustering.
-
This video will help you to understand basics of Association rule learning. In this session we will learn about the Apriori algorithm and the working of Apriori algorithm.
-
This video will help you to understand advanced topics of Association rule learning such as implementation of Apriori algorithm.
-
This is a session on the practical part of Data Science application. In this example we will see problem statement, data set, exploratory data analysis.
-
This is a session on the practical part of Data Science application.
-
This is a session on the practical part of Data Science application. In this we will see the implementation of the project.
-
This is a session on the practical part of Data Science application
-
This is a session on the practical part of Data Science application
Course/Topic 7 - Data Science with R - all lectures
-
In this lecture session we learn about introduction of data science and also talk about features of data science in R.
-
In this lecture session we learn about data collection and management and also talk about features of data collection and management in data science with R.
-
In this lecture session we learn about model deployment and maintenance and also talk about functions of model deployment and maintenance in data science with R.
-
In this lecture session we learn about setting expectations and also talk about factors of setting expectations in brief.
-
In this lecture session we learn about loading data into R and also talk about features of loading data into R and also talk about the importance of loading data into R.
-
In this lecture session we learn about exploring data in data science and machine learning and also talk about features of exploring data in data science and machine learning.
-
In this lecture session we learn about features of exploring data using R and also talk about factors of exploring data using R.
-
In this lecture session we learn about benefits of data cleaning and also talk about features of benefits of data cleaning.
-
In this lecture session we learn about cross validation in R and also talk about features of validation in data science with R.
-
In these lecture sessions we learn about data transformation in data science with R and also talk about features of data transformation in brief.
-
In this lecture session we learn about modeling methods in data science with R and also talk about the importance of modeling methods.
-
In this lecture session we learn about solving classification problems and also talk about features of solving classification problems in brief.
-
In this lecture session we learn about working without known targets in data science with r and also talk about features of working without known targets.
-
In this lecture session we learn about evaluating models in data science with R and also talk about features of evaluating models in brief.
-
In this lecture session we learn about confusion matrix in indian accounting standards and also talk about features of confusion matrix.
-
In this lecture session we learn about introduction to linear regression and also talk about features of linear regression in indian accounting standards.
-
In this lecture session we learn about linear regression in R and also talk about features and functions of linear regression in brief.
-
In this lecture session we learn about linear regression in R in data science with r and also talk about features of linear regression in R language.
-
In this lecture session we learn about simple and multiple regression in data science with r and also talk about the basic difference between simple and multiple regression in brief.
-
In this lecture session we learn about linear and logistic regression in data science with r language and also talk about functions of linear and logistics regressions.
-
In this lecture session we learn about support vector machines (SVM) in R and also talk about features of support vector machines in data science with R language.
-
In this lecture session we learn about factors of support vectors machines in data science with R and also talk about features of support vectors machines.
-
In this lecture session we learn about unsupervised methods in data science with R and also talk about functions of unsupervised methods in data science.
-
In this lecture session we learn about clustering in data science with R language and also talk about features of clustering in data science.
-
In this lecture session we learn about K-means algorithms in R and also talk about all types of algorithms in data science with R language.
-
In this lecture session we learn about hierarchical clustering in data science with R language and also talk about features of hierarchical clustering.
-
In this lecture session we learn about libraries in data science with R and also talk about libraries of hierarchical clustering in brief.
-
In this lecture session we learn about the dendrogram of diana and also talk about all types of clustering in data science with R.
-
In this lecture session we learn about market basket analysis in data science with R and also talk about features of market basket analysis in data science with R.
-
In this lecture session we learn about MBA and association rule mining in data science with r language.
-
In this lecture session we learn about implementing MBA in data science with R and also talk about implementing MBA.
-
In this lecture session we learn about association rule learning in data science with R and also talk about features of association rule learning.
-
In this lecture session we learn about decision tree algorithms in data science with R and also talk about features of tree algorithms.
-
In this lecture session we learn about exploring advanced methods in tree algorithms in data science with R and also talk about features of exploring advanced methods.
-
In this lecture session we learn about using kernel methods and also talk about features of using kernel methods in data science with R.
-
In this lecture session we learn about documentation and deployment and also talk about features of documentation and deployment in data science with R.
Course/Topic 8 - Data Visualization in Python - all lectures
-
In this first video tutorial on Data Visualization in Python course, you will get a brief introduction and overview on what is data visualization, its importance, benefits and the top python libraries for Data Visualization like Matplotlib, Plotly and Seaborn.
-
In this first part of the video on Matplotlib, you will learn both the theoretical and the practical knowledge on Matplotlib, which is one of the most popular and top python libraries for Data Visualization. You will get a complete introduction to Matplotlib, the installation of Matplotlib with pip, the basic plotting with Matplotlib and the Plotting of two or more lines in the same plot.
-
In this second part of the Matplotlib video tutorial, you will learn how to add labels and titles like plt.xlabel and plt.ylabel along with understanding how to create lists and insert functions onto it. All this can be seen explained it detail by the instructor by taking examples for it.
-
In this tutorial, you will learn about 2 important python libraries namely; Numpy and Pandas. Along with the theoretical concepts, you will also get practical implementation on various topics related to these two such as what is Numpy and what is its use, the installation of Numpy along with example, what is pandas and its key features, with the installation of Python Pandas and finally the Data Structure with examples of Pandas.
-
In this second part of the Numpy and Pandas tutorial, you will learn the complete overview of Pandas like its history, its key features, the installation process of Pandas, Pandas Data Structure and within it the Data Frame and syntax to create Data Frame. All this will be explained in detail by the instructor.
-
In this third part of the video tutorial on Numpy and Pandas, you will learn about creating Data Frame from Dictionary. Also, you will understand how to read CSV Files with Pandas using practical examples by the Instructor.
-
In this tutorial, you will learn about the different Data Visualization Tools such as Bar Chart, Histogram and the Pie Chart. You will get a complete understanding of what is these tools, why and how to use these 3 tools, the syntax for creating Bar Chart, Histogram and the Pie Chart and different programs for creating these data visualization tools. In the first part of the video, you will learn about the Bar Chart and in the subsequent videos, you will learn about the Histogram and the Pie Chart.
-
In this second part of the Data Visualization Tools video, you will learn about the complete overview of Histogram like what is Histogram, how to create Histogram and many others with the help of practical examples by the instructor.
-
In this third and final part of the Data Visualization Tools video, you will learn about the Pie Chart-what is Pie Chart, how to create the Pie Chart and how to create the syntax for Pie Chart? All these questions will be explained in detail by the instructor by taking practical examples. Further, you will understand the concept of Autoptic parameter in Pie Chart.
-
In this first part of the video tutorial on more data visualization tools, you will learn about some additional data visualization tools apart from Bar Chart, Histogram and Pie Chart such as Scatter Plot, Area Plot, STACKED Area Plot and the Box Plot. The first part of this tutorial consists of mainly the Scatter Plot, the theoretical concepts associated with it such as what is Scatter Plot, the syntax for creating Scatter Plot and creating Scatter Plot with examples.
-
In this second part of the video tutorial, you will learn and understand what is Area Plot, creating Area Plot with Function and Syntax and creating Area Plot with examples. All these will be seen explained in detail by the instructor. Further, you will also learn and understand the concept associated with the STACKED Area Plot.
-
In this final part of the video tutorial, you will learn about the Box Plot; which is also known as Whisker Plot, how to create Box Plot, its syntax and arguments used like Data & Notch, the parameters used in Box Plot such as vert, patch artist and widths. These will be seen explained in detail by the instructor.
-
In this first video tutorial on Advanced Data Visualization Tools, you will learn about the Waffle Chart – its definition, complete overview, the syntax and programs to create Waffle Chart and the step-by-step procedure to create the Waffle Chart. All these will be seen explained in detail by the instructor.
-
In this second part of the video tutorial on Advanced Data Visualization Tools, you will learn about the Word Cloud-its definition, the reason why Word Cloud is used, what are the modules needed in generating the Word Cloud in Python, how to install Word Cloud and how to create Word Cloud with the help of some examples.
-
In this tutorial, you will learn and understand about the concept of Heat Map and how one can create the Heat Map along with the help of the parameter camps. This will be seen explained in detail by the instructor.
-
In this first part of the video tutorial on Specialized Data Visualization Tools, you will learn about the Bubble Chart; its definition and how to create bubble charts with the help of different examples.
-
In this video, you will learn about the Contour Plots; which is also sometimes referred to as Level Plots. Along with understanding the whole theoretical concept of Contour Plots, you will also learn how to create Contour Plots with practical examples as will be seen explaining by the instructor in details.
-
In this third part of the video on Specialized Data Visualization Tools, you will learn about the Quiver Plot and how to create the Quiver Plot by taking different examples. This will be seen explained in complete details by the instructor.
-
In this video on Specialized Data Visualization Tools, you will learn about 3D plotting in Matplotlib and also the 3D Line Plot used in Data Visualization with the help of different practical examples and how to create it. This will be seen explained in detailed by the instructor throughout the tutorial.
-
In this tutorial, you will learn about the 3D Scatter Plot and how to create a 3D Scatter Plot. The instructor will be seen explaining this in complete details with the help of different examples.
-
In this tutorial, you will learn and understand the 3D Contour Plot, what is the function used in creating the 3D Contour Plot and how it can be created; which will be explained in detail by the instructor with the help of examples.
-
In this last part of the video tutorial on Specialized Data Visualization Tools, you will learn about the 3D Wireframe Plot and the 3D Surface Plot, along with creating the same with the help of different examples, seen explained in detail by the instructor.
-
In this tutorial, you will learn about Seaborn, which is another very important Python library. Through this video, you will get an introduction to Seaborn, along with some important features of it, functionalities of Seaborn, Installation of Seaborn, the different categories of plot in Seaborn and some basic type of plots one can create using Seaborn like Distribution Plot.
-
In this second part of the video on Seaborn Library, you will learn and understand some basic plots using Seaborn Library like the Line Plot. Here, the instructor will be seen explaining in detail the Seaborn Line Plot and with a detailed example of how to create Seaborn Line Plot with random data.
-
This is a continuation video of creating the Line Plot with some more examples using the Seaborn library. Along with this, you will also learn about the Lmplot and the function used for creating the Lmport. This can be seen explained in detailed by the instructor with practical examples.
-
In this tutorial, you will learn about Data Visualization using Seaborn library. Under this, you will learn the Strip Plot, how to create the strip plot and the program used to create the Strip Plot. This will be shown by the Instructor with detailed examples like Strip plot using inbuilt data-set given in Seaborn and others.
-
In this video, you will learn about the Swarm Plot; its definition, complete overview and how you can create the Swarm Plot. This can be seen explained in detail by the instructor with examples like visualization of “fmri” dataset using swarm plot().
-
In this tutorial, you will learn a complete overview on Plotting Bivariate Distribution along with the concepts of Hexbin Plot, Kernel Density Estimation (KDE) and the Reg Plots. You will understand many of the in-depth concepts on these, with detailed explanation by the instructor with examples.
-
In this tutorial, you will learn about the Pair Plot Function in Visualizing Pairwise Relationship under Seaborn library. You will understand the complete overview of Pair Plot Function, the syntax for using it, the parameters used like hue, palette, kind and diag kind. This will be seen explained in detail by the instructor with the help of examples.
-
In this tutorial, you will learn about the Box Plot, Violin Plots and the Point Plots – their definitions and how to create them which will be seen explained in detail by the instructor throughout the video.
Course/Topic 9 - Data Visualization in R - all lectures
-
In this introductory tutorial on Data Visualization in R Programming, you will learn about what is data visualization, the type of graph or chart one should select for data visualization, what is the importance and benefits of data visualization and finally what are the applications of data visualization.
-
In this video, you will learn how to work on the Histogram, which falls under different Chart types used in Data Visualization in R Programming; along with working on the bar chart, box plot and heat map. You will be seeing a detailed explanation by the instructor on the complete workaround of these by taking different examples.
-
In this video, you will learn what is density plot and how you can create the density plot by taking different examples for it. You will also learn about the different applications being used in the density plot under Data Visualization with R Programming.
-
In this tutorial, you will learn about Data Visualization with GGPLOT2 Package where inside it you will learn the overview of GGPLOT2, iteratively building plots, univariate distributions and bar plot, annotation with GGPLOT2, axis manipulation and the density plot. You will get a complete understanding of the theoretical concept along with the implementation of each of these.
-
In this second part of the video tutorial, you will learn about Plotting with GGPLOT2 and building your plots iteratively, along with the importance of the ‘+’ symbol and its use in the GGPLOT2 work process. You will be seeing a detailed explanation from the instructor by taking different examples.
-
In this video you will learn about the complete theoretical and practical implementation of Univariate Distribution and Bar Plot, which can be seen explained in complete details by the instructor throughout the tutorial.
-
In this tutorial, you will learn about annotation with ggplot2, along with geom text () and adding labels with geom label () with complete explanation on this by the instructor with the help of different examples.
-
In this tutorial, you will learn about Axis Manipulation with ggplot2, its complete overview and in-depth concepts along with the different functions used during the process. You will be seeing explaining the topic in complete details by the instructor by taking examples and working in R studio.
-
In this section, you will learn about Text Mining and Word Cloud, along with the Radar Chart, Waffle Chart, Area Chart and the Correlogram. In this first part of the video, you will learn about the Text Mining and Word Cloud, the different reasons behind using Word Cloud for text data, who is using Word Clouds and the various steps involved in creating word clouds.
-
In this video, you will learn how to execute data using redline function. Also, you will understand the usage of corpus function and content transformer function. Further, you will understand about the text stemming, Term Document Matrix function and the Max word’s function.
-
In this tutorial, you will learn about the Radar Chart, the function used in the Radar Chart which is gg Radar (), scales, mapping and the use label. Along with this, you will also learn how to create Radar Chart in R studio. Moreover, you will learn about the Waffle Chart in R and how to create vector data in Waffle Chart with the help of different examples.
-
In this last part of the session, you will learn about the Area Chart, its in-depth concepts and how to work on it. This will be seen explained in detail by the instructor. Moreover, you will also learn about the Correlogram in R, the correlation matrix, Mt cars and the work around on different visualization methods been used.
-
This is a project tutorial titled Visualizing COVID-19 where you will see the different scenarios being explained by the tutor on visualizing COVID-19 data and how it can be done through Data Visualization in R process. In this first part, you will understand the complete overview of the project, its description and the different tasks associated with it being done by the ggplot.
-
In this second part of the project video, you will learn about the “Annotate” process and the number of COVID cases being reported in China with the help of Data Visualization. You will be seeing the task performed on the dataset being provided by the WHO along with understanding the tribble function and how it will help during the entire work process.
-
In this last part of the session, you will understand the work around of the task being done with the help of plot. You will see a detailed explanation by the instructor seeking help of few examples to explain the complete process of plotting in respect to the COVID-19 project being implemented.
Course/Topic 10 - Business Intelligence and Data Analytics - all lectures
-
In this lecture session we discuss about Bi concepts, examples and application of business intelligence and data analytics and also cover other concepts of BI.
-
In this lecture session we learn about basic concepts of BI and also cover factors of business intelligence in brief.
-
In this lecture session we learn about data warehouse data access and data dashboarding and also cover presentation in BI.
-
In this lecture session we learn about product database, advertise database and customer demographic database and also cover data analyst concepts.
-
In this lecture session we learn about basic introduction of business intelligence and also cover factors of business intelligence in brief.
-
In this lecture session we learn about introduction of predictive modeling and also cover functions of predictive modeling in brief.
-
In this lecture session we learn about data related to customer services and also talk about customer relation databases in brief.
-
In this lecture session we learn about introduction of NoSQL and also cover basic functions of NoSQL in business intelligence.
-
In this lecture session we learn about graph stores and also talk about the advantages and disadvantages of graph stores in BI.
-
In this lecture session we learn about hierarchical clustering in business intelligence and also talk about clustering factors in BI.
-
In this lecture session we learn about introduction of salesforce in business intelligence and also talk about some basic uses of salesforce.
-
In this lecture session we learn about introduction to NLP and also cover what is natural language processing in artificial intelligence.
-
In this lecture session we learn about natural language processing speech to text conversion and also cover the importance of natural language processing.
-
In this lecture session we learn about introduction of apache server in business intelligence and also talk about basics of apache server.
-
In this lecture session we learn about deep drive into business intelligence and also talk about factors or deep drive in business intelligence.
-
In this lecture session we learn about data warehousing in business intelligence and data analytics and also talk about factors and features of data warehousing.
-
In this lecture session we learn about types of data in business intelligence and also talk about different types of data in BI.
-
In this lecture session we learn about mobile BI and also talk about open source BI software replacing vendor offering.
-
In this lecture session we learn about real time BI in business intelligence and also talk about factors of real time BI in brief.
-
In this lecture session we learn about data analytics comprehensively and also talk about functions of data analytics.
-
In this lecture session we talk about data analytics vs business analytics and also talk about the importance of data analytics.
-
In this lecture session we learn about Embedded analytics and also talk about functions of Embedded analytics in data analytics.
-
In this lecture session we learn about collection analytics and also cover the importance of collection analytics.
-
In this lecture session we learn about survival analytics and also cover duration analytics in brief.
-
In this lecture session we learn about machine learning techniques and also cover the importance and factors of machine learning techniques in business intelligence.
-
In this lecture session we learn about geospatial predictive analytics and also talk about functions of geospatial predictive analytics in business intelligence.
-
In this lecture session we learn about cohort analysis in data analyst and we also cover functions and importance of cohort analysis.
-
In this lecture session we learn about data mining in business intelligence and also talk about data mining functions and why we need data mining in business intelligence.
-
In these lecture sessions we learn about anomaly detection and also talk about functions of anomaly detection in brief.
-
In these lecture sessions we learn about statistically sound association and also talk about factors of statistically sound association in business intelligence.
-
In this lecture session we learn about cluster analysis. We’ll cover all types of cluster analysis in brief and also cover the importance of cluster analysis in business analysis.
-
In this lecture session we learn about DBSCAN in business intelligence and also talk about DBSCAN functions and importance.
-
In this lecture session we learn about regression models in business intelligence and also talk about the function of regression models.
-
In this lecture session we learn about extraction based summarization in business intelligence and also cover all types of summarization in data analyst.
-
In this lecture session we learn about machine learning in BI and also talk about factors and importance of machine learning in brief.
-
In this lecture session we learn about machine learning vs BI we also discuss the basic difference between machine learning and business intelligence.
-
In this lecture session we learn about how ml can make BI better and also talk about ml basic functions.
-
In this lecture session we learn about data warehousing and also talk about how we manage data warehousing in business intelligence.
-
In this lecture session we learn about data warehousing in business intelligence and data analytics and also talk about factors and features of data warehousing.
-
In this lecture session we learn about data mart in business intelligence and also talk about data mart function.
-
In this lecture session we learn about data dimensions in business intelligence and also cover all types of data dimension in BI.
-
In this lecture session we learn about data dimension in business intelligence and also cover functions and importance of data dimension.
-
In this lecture session we learn about data vault modeling in business intelligence and also cover different types of vault modeling in brief.
-
In this lecture session we learn about links and satellites and also cover the importance and factors of links and satellites in business intelligence.
Course/Topic 11 - Machine Learning with Python - all lectures
-
In this lecture session we learn about basic introduction to machine learning and also talk about This machine learning process starts with feeding them good quality data and then training the machines by building various machine learning models using the data and different algorithms.
-
In this lecture session we learn about types of machine learning in machine learning and also talk about their primary three types of machine learning we also explore and understand the different types of machine learning.
-
In this lecture session we learn about Supervised, Unsupervised, and Reinforcement Learning in brief and also talk about some features and factors of Supervised, Unsupervised, and Reinforcement machine Learning.
-
In this lecture session we learn about The primary rationale for adopting Python for machine learning is because it is a general purpose programming language that you can use both for research and development and in production. In this post you will discover the Python ecosystem for machine learning.
-
In this tutorial we learn about components of python ML Ecosystem in machine learning and also talk about features and factors of Object-Oriented Language: One of the key features of python is Object-Oriented programming. Python supports object-oriented language and concepts of classes, object encapsulation, etc.
-
In this tutorial we learn about what pandas is in machine learning and also talk about the pandas package of the most important tool in machine learning and all different tools in brief.
-
In this lecture session we learn about The most common data format for ML projects is CSV and it comes in various flavors and varying difficulties to parse. In this section, we are going to discuss three common approaches in Python to load CSV data files .
-
In this tutorial we learn about regression analysis helps one understand how the typical value of the dependent variable (or 'criterion variable') changes when any one of the independent variables is varied, while the other independent variables are held fixed and also talk about different types of Regression analysis.
-
In this tutorial we learn about how Linear regression is used to predict the value of a continuous dependent variable with the help of independent variables. Logistic and also talk about linear regression is both a statistical and a machine learning algorithm. Linear regression is a popular and uncomplicated algorithm used in data science and machine learning.
-
In this lecture session we learn about the scikit-learn library in machine learning and also talk about what Scikit-Learn is, how it’s used, and what its basic terminology is. While Scikit-learn is just one of several machine learning libraries available in Python, it is one of the best known. The library provides many efficient versions of a diverse number of machine learning algorithms.
-
In this lecture session we learn about creating a train and test dataset in machine learning and also talk about The test data set contains data you are going to apply your model to. In contrast, this data doesn’t have any "expected" output. During the test phase of machine learning, this data is used to estimate how well your model has been trained and to estimate model properties.
-
In this tutorial we learn about multiple regression is the extension of ordinary least-squares (OLS) regression because it involves more than one explanatory variable. Multiple linear regression (MLR), also known simply as multiple regression, is a statistical technique that uses several explanatory variables to predict the outcome of a response variable.
-
In this lecture session we learn about examples of multiple linear regression in machine learning and also talk about features and functions of Linear regression that can only be used when one has two continuous variables—an independent variable and a dependent variable.
-
In this tutorial we learn about Polynomial Regression is a regression algorithm that models the relationship between a dependent (y) and independent variable (x) as nth degree polynomial. The Polynomial Regression equation is given below: It is also called the special case of Multiple Linear Regression in ML.
-
In this lecture session we learn about classification in machine learning as a supervised learning approach and also talk about attempts to learn between a set of variables and a target set of variables of a test.
-
In this tutorial we learn about Logistic regression models to help you determine a probability of what type of visitors are likely to accept the offer — or not. As a result, you can make better decisions about promoting your offer or make decisions about the offer itself and also talk about The three types of logistic regression are: Binary logistic regression is the statistical technique used to predict the relationship between the dependent variable (Y) and the independent variable (X), where the dependent variable is binary in nature. For example, the output can be Success/Failure, 0/1 , True/False, or Yes/No.
-
In this lecture session we learn about what KNN K-nearest neighbors (KNN) is a type of supervised learning algorithm used for both regression and classification. KNN tries to predict the correct class for the test data by calculating the KNN
-
In this lecture session we learn about encoding data columns in machine learning Encoding is the process of converting the data or a given sequence of characters, symbols, alphabets etc., into a specified format, for the secured transmission of data. Decoding is the reverse process of encoding which is to extract the information from the converted format. Data Encoding.
-
In this tutorial we learn about decision trees in machine learning. Decision tree is the most powerful and popular tool for classification and prediction. A Decision tree is a flowchart like tree structure, where each internal node denotes a test on an attribute, each branch represents an outcome of the test, and each leaf node (terminal node) holds a class label.
-
In this lecture session we learn about Support Vector Machine Algorithm. Support Vector Machine (SVM) is a supervised machine learning algorithm used for both classification and regression. Though we say regression problems as well, it's best suited for classification.
-
In this lecture session we learn about An Overview of Clustering in the Cloud. Computer clusters, and in particular Kubernetes clusters, have seen a substantial rise in adoption in the last decade. Startups and tech giants alike are leveraging cluster-based architectures to deploy and manage their applications in the cloud.
-
In this lecture session we learn about Cluster analysis is an essential human activity. Cluster analysis is used to form groups or clusters of the same records depending on various measures made on these records. The key design is to define the clusters in ways that can be useful for the objective of the analysis.
-
In this lecture session we learn about Hierarchical clustering begins by treating every data point as a separate cluster. Then, it repeatedly executes the subsequent steps: Merge the 2 maximum comparable clusters. We need to continue these steps until all the clusters are merged together. In Hierarchical Clustering, the aim is to produce a hierarchical series of nested clusters.
-
In this tutorial we learn about implementation of Agglomerates hierarchical clusters in machine learning and also talk about features of hierarchical clusters.
-
In this tutorial we learn about Association Rule Learning is a rule-based machine learning technique that is used for finding patterns (relations, structures etc.) in datasets. By learning these patterns we will be able to offer some items to our customers.
-
In this tutorial we learn about Data Mining enables users to analyze, classify and discover correlations among data. One of the crucial tasks of this process is Association Rule Learning. An important part of data mining is anomaly detection, which is a procedure of search for items or events that do not correspond to a familiar pattern.
-
In this lecture session we learn that Recommender systems are so commonplace now that many of us use them without even knowing it. Because we can't possibly look through all the products or content on a website, a recommendation system plays an important role in helping us have a better user experience, while also exposing us to more inventory we might not discover otherwise.
-
In this lecture session we learn about Recommender Function. An important component of any of these systems is the recommender function, which takes information about the user and predicts the rating that user might assign to a product, for example. Predicting user ratings, even before the user has actually provided one, makes recommender systems a powerful tool.
-
In this lecture session we learn about Collaborative filtering is a difference of memory-based reasoning especially well appropriated to the application of supporting personalized recommendations. A collaborative filtering system begins with a history of personal preferences.
-
In this tutorial we learn about implementation of move recommender systems in machine learning and also talk about features and functions of implementation of move recommender systems in brief.
Course/Topic 12 - Machine Learning (basic to advanced) - all lectures
-
In this session we will learn about introduction to Machine Learning. We will start by learning about the basics of Linear Algebra required to learn Machine Language. Further we will learn about Linear equations represented by Matrices and Vectors.
-
In this module we will learn about the computational roots of matrices. We will learn how to multiply matrix with scalar and vector. We will learn about addition and subtraction of matrices.
-
In this module we will learn about Num-Pie Linear Algebra to work on Python. It further includes the understanding of the use of functions - #dot, #vdot, #inner, #matmul, #determinant, #solve, #inv. Basic examples of the #dot, #vdot functions will be discussed.
-
In this module we will learn about how the #inner function work in a two-dimension array. We will also learn its usage in #dot and #vdot. We will see explanation of the functions solving examples.
-
In this module we will learn about using #matmul function. We will learn about normal product and stack of arrays. We will also learn how to check the dimensions of the array and how to make it compatible.
-
In this module we will learn about the #determinant function. The basics of the #determinant function will be explained. Examples will be solved with explanations to understand it.
-
In this module we will learn what a Determinant is. We will also learn about how to find a Determinant. We will further learn how to find the Determinant of a 2*2 and 3*3 matrix learn about the basics of #inv function.
-
In this module we will learn about the #inv function. We will learn about how to find the inverse of a matrix. We will also learn how to find the Identity matrix for the inverse.
-
In this module we will discuss about the inverse of a matrix. We will understand what an Inverse is. We will further learn how the Inverse of a matrix is found.
-
In this module we will learn about the difference of the dot( ) and the inner( ). We will see examples of dot( ) and inner( ), We will also learn about the dissimilarities between the dot( ) and inner( ) with the help of examples.
-
In this module we will learn about numpy matrix. We will learn the different ways of creating a matrix. We will also learn about a vector as a matrix and its multiplication with matrix.
-
In this module we will learn about the #numpy.vdot( ) function. This module is a continuation of the previous module. We will also learn about the #numpy,inner( ) function.
-
In this module we will understand the different concepts like Rank, Determinant, Trace, etc, of an array. Then we will learn how to find the item value of a matrix. We will also learn about the matrix and vector products.
-
In this module we will learn about the matrix and vector products. We will learn about how it works on imaginary and complex numbers. We will also get an understanding of matmul( ), inner( ), outer( ), linalg.multi_dot( ), tensordot( ), einsom( ), einsum_path( ),linalg.matrix_power( ).
-
In this module we will learn about the basics of #inverse of a matrix. We will understand what an Inverse is. We will also see examples of inverse of a matrix and learn how to calculate it.
-
In this module we will learn about the basics of Python. We will also learn about the Packages needed by the machine language. We will further learn the basics of numpy, scipy, pandas, skikit-learn, etc. needed machine learning and data science.
-
In this module we will understand about SciPy. We will also learn about SkiKit-learn and Theano. We will further learn about TensorFlow, Keras, PyTorch, Pandas, Matplotlib.
-
In this module we will see examples of the topics discussed in the previous module. We will also start the basics of Python. We will also solve some basic problems.
-
In this module we will continue the basic problems of Python. We will also understand about Operators. We will also see the different operators and its applications.
-
In this module we will continue learning the different Operators. We will also learn about Advanced Data types. We will learn and understand the different data types and about Sets.
-
In this module we will learn about list. We will see the different functions of list. We will also learn about Jupyter notebook.
-
In this module we will learn about #condition statements in Python in brief. We will also learn about the applications of #condition statements We will solve some examples to understand the #condition statements.
-
In this module we will learn about the Loop in Pyhton. We will also learn about the different kinds of loops. We will see examples of For loop, and break keyword.
-
In this module we will continue with the #for loop. We will also learn about the continue keyword. We will solve examples for the usage of the keywords.
-
In this module we will learn about Functions in Python. We will solve examples using different functions. We will understand how functions work.
-
In this module we will learn about arguments in functions. We will also solve examples to understand the usage of arguments in functions. We will also learn about #call by reference in Python.
-
In this module we will learn about strings. We will also learn about types of arguments for functions in python. We will also see the usage of the different types of arguments.
-
In this module we will learn about default arguments. We will also learn about variable arguments. We will solve examples to understand it better.
-
In this module we will learn about the remaining arguments. We will understand about default and variable arguments better. We will also learn about keyword arguments.
-
In this module we will learn about built-in functions. We will also learn about the different built-in functions in python. We will solve examples to understand the functions better.
-
In this module we will continue the previous functions. We will also learn about other built-in functions. We will also learn about bubble sort in python.
-
In this module we will learn about the scope of variable in function. We will also learn about the different variables and its usage. We will solve examples using the different variables to understand it better.
-
In this module we will learn about the math module in python. We will learn about the different inbuilt functions that deal with math functions. We will solve problems using the different math functions.
-
In this module we will continue with the previous lecture. We will also learn about the different arguments in functions. We will also learn about call by reference in python.
-
In this module we will continue with the previous lecture. We will also start mathplotlib in python. We will learn the different types of mathplotlib by using jupyter.
-
In this module we will learn about loan calculator using tkinter. We will also learn how to use the loan calculator. We will solve an example to understand its usage.
-
In this module we will continue with the previous lecture. We will learn how to compute payments using functions. We will also learn about the function getmonthlypayment.
-
In this module we will learn about numpy function. We will also learn about mathematical and logical operations using numpy. We will also be explained about different numpy arrays.
-
In this module we will continue with the previous lecture. We will learn about different numpy attributes. We will solve examples using the different attributes and slicing an array.
-
In this module we will learn about advanced slicing of an array. We will use jupyter to do array slicing. We will understand detail how array slicing works.
-
In this module we will learn about using jupyter notebook online. We will also learn about ranges. We will learn about creating arrays from ranges. We will also learn about linear space.
-
In this module we will learn about the average function. We will also learn about the different averages. We will solve examples to understand the function.
-
In this module we will learn about generating random strings and passwords. We will also learn about generating a string of lower and upper case letters. We will solve examples using the different strings.
-
In this module we will learn about generating strings. We will also learn about upper case letters and only printing specific letter. We will also learn about alpha numeric letters.
-
In this module we will learn about the unique function. We will continue using arrays. We will solve example using unique functions in arrays.
-
In this module we will learn about array manipulation function, We will learn about the delete function in numpy. We will solve examples for better understanding.
-
In this module we will learn about the insert function in numpy. We will also learn about flattened array. We will solve examples.
-
In this module we will learn about examples with two dimension arrays. We will also learn about the ravel function. We will also learn about the rollaxis function, swapaxes function.
-
In this module we will learn about statistical functions. We will also learn about min and max values. We will solve examples using the functions.
-
In this module we will learn about functions for rounding. We will also learn about round off function, floor function and ceil function. We will solve examples using the functions.
-
In this module we will learn about numpy append function. We will also learn about resize function. We will solve examples.
-
In this module we will learn about numpy nonzero function. We will also learn about the where function. We will solve examples using the different functions.
-
In this module we will learn about matrix library. We will also learn about the different matlib functions We will solve different examples using the matlib function. vvvv
-
In this module we will learn about the basic operations that can be done on numpy arrays. We will also learn about arithmetic operations and functions. We will do examples with arithmetic operations.
-
In this module we will learn about numpy filter array. We will do programs on numpy filter array. We will solve examples using the filter array.
-
In this module we will learn about array manipulation functions. We will see how the array manipulation functions work. We will learn about the different manipulation functions.
-
In this module we will learn about broadcasting function in numpy. We will also learn about reshape in numpy. We will also learn about removing function in numpy.
-
In this module we will learn about indexing. We will also learn about slicing. We will solve examples to understand the concept.
-
In this module we will learn about numpy append function. We will also learn about resize function. We will solve examples using the functions.
-
In this module we will learn about conversion of numpy dtypes to native python types. We will also learn to create 4*4 matrix in which 0 and 1 are staggered with zero on the main diagonal. We will also learn to create 10*10 matrix elements on the borders will be equal to 1 and inside 0.
-
In this module we will learn how to use a python program to find the maximum and minimum value of a flattened array. We will also see the function called flat and flatten to make the array flattened. We will learn about function import numpy as np and array-np.arrange( )
-
In this module we will learn how to generate a random string of a given length. Tutor will address the issues faced in generating random strings. Further in the video, we will discuss the various ways in which generation of a random staring can be performed.
-
In this video we will be covering on creating a simple project. We will see the practical on how tutor creates a simple project. We will also see some examples on how to create a simple project. The video talks about how to get common items between 2 python numpy arrays.
-
In this video we will talk about another function in python programming called the split function. The function split divides the arrays into sub arrays. The split() method splits a string into an array of substrings. The split() method returns the new array. The split() method does not change the original string. If (" ") is used as separator, the string is split between words.
-
This video is a sequel of explanation of spilt function. We will discuss the three types of split functions – 1. Normal split, 2. Horizontal split and 3. Vertical Split. Further we will discuss the roles of split function and what do they do.
-
In this video we will learn about the numpy filter array. We will further see what is filtering of array. Getting some elements out of an existing array and creating a new array out of them is called filtering of array, using a bullion index list.
-
In this video we will learn about an important topic in Python, i.e Python file handling. We will see what is a file and the type of executable files. Further we will see what is output and how to view the output. Different access modes that can be opened with the file.
-
In this video we will see an example on how to open and file in view mode, by giving the name of the file. File statement in Python is used in exception handling to make the code cleaner and much more readable. It simplifies the management of common resources like file streams. ... with open ( 'file_path' , 'w' ) as file : file .
-
This video is a continuation of file system tutorial. Here we will see to use the append mode and what is append mode. Python has a built-in open() function to open a file. This function returns a file object, also called a handle, as it is used to read or modify the file accordingly. We can specify the mode while opening a file. In mode, we specify whether we want to read r , write w or append a to the file.
-
In this module we will start a new topic known as random module which is a very important part in numpy. Further we will discuss the functionalities of random module to generate random numbers.
-
In this module we will see how to generate the arrays on float and hot generate a single floating value from 0 to 1. Further we will see taking array as a parameter and randomly return one of the values.
-
In this module we will learn the random module in continuations. The random is the module present in the numpy library. This module contains simple random generation methods.
-
In this module how random module contains functions used to generate random numbers. We will also see some permutations and distribution functions.
-
In this module we will see the choice functions and the different variants of choice function. Further we will see how to randomly select multiple choices from the list. Random.sample or random.choices are the functions used to select multiple choices or set.
-
In this module we will see the difference between the sample function and the choices functions. Further, we will do a random choice from asset with Python, by converting it to tuple.
-
In this module we will learn about the random Boolean in Python, using random.choice. In order to generate Random boolean, we use the nextBoolean() method of the java. util. Random class. This returns the next random boolean value from the random generator sequence
-
In this module we will learn about the library available in python that is called Pandas. We will see how Pandas is one of the important tools available in Python. Further we will see how Pandas makes sense to list the things.
-
In this module we will learn about the basics of Pandas. Further we will see how this an important tool for Data scientist and Analysts and how pandas is the back bone of most of the data projects.
-
This module is a sequel of the previous tutorial on Pandas. In this module we will see practical project on pandas using series and dataframes. Lastly we will learn how to handle duplicate and how to handle information method and shape attribute.
-
In this video we will see about column clean and how to clean the column. Further we will see how to rename the columns by eliminating symbols and other different ways.
-
In this module we will learn about how to work with the missing values or null values. Further we will see if the dataset is inconsistent or has some missing values then how to deal with the missing values when exploring the data.
-
In this video we will see how to perform the imputation on column, i.e., metascore which has some null values. Further we will see how to use describe function on the genre column of the dataset.
-
In this module we will learn about the frequency of columns. Further we will see about the functio0n called value counts. The value counts function when used on the genre column tells us the frequency of all the columns.
-
In this video we will learn about the methods of slicing, selecting and extracting. If these methods are not followed properly then we will receive attribute errors. Further we will learn to manipulate and extract data using column headings and index locations.
-
2.7 MATPLOTLIB BASICS
-
2.7.1 MATPLOTLIB BASICS
-
2.7.2 MATPLOTLIB BASICS
-
2.7.3 MATPLOTLIB BASICS
-
2.7.4 MATPLOTLIB BASICS
-
2.7.5 MATPLOTLIB BASICS
-
2.7.6 MATPLOTLIB BASICS
-
2.7.7 MATPLOTLIB BASICS
-
2.7.8 MATPLOTLIB BASICS
-
2.7.9 MATPLOTLIB BASICS
-
2.7.9.1 MATPLOTLIB BASICS
-
2.7.9.11 MATPLOTLIB BASICS
-
2.8 AGE CALCULATOR APP
-
2.8.1 AGE CALCULATOR APP
-
2.8.2 AGE CALCULATOR APP
-
2.8.3 AGE CALCULATOR APP
-
3.1 MACHINE LEARNING BASICS
-
3.1.1 MACHINE LEARNING BASICS
-
3.1.2 MACHINE LEARNING BASICS
-
3.1.3 MACHINE LEARNING BASICS
-
3.1.4 MACHINE LEARNING BASICS
-
3.1.5 MACHINE LEARNING BASICS
-
3.1.6 MACHINE LEARNING BASICS
-
3.1.7 MACHINE LEARNING BASICS
-
3.1.8 MACHINE LEARNING BASICS
-
3.1.9 MACHINE LEARNING BASICS
-
3.1.9.1 MACHINE LEARNING BASICS
-
3.2 MACHINE LEARNING BASICS
-
4.1 TYPES OF MACHINE LEARNING
-
4.1.1 TYPES OF MACHINE LEARNING
-
4.1.2 TYPES OF MACHINE LEARNING
-
4.1.3 TYPES OF MACHINE LEARNING
-
4.1.4 TYPES OF MACHINE LEARNING
-
4.1.5 TYPES OF MACHINE LEARNING
-
4.1.6 TYPES OF MACHINE LEARNING
-
5.1 TYPES OF MACHINE LEARNING
-
5.1.1 TYPES OF MACHINE LEARNING
-
5.1.2 TYPES OF MACHINE LEARNING
-
5.1.3 TYPES OF MACHINE LEARNING
-
5.1.4 TYPES OF MACHINE LEARNING
-
5.1.5 TYPES OF MACHINE LEARNING
-
5.1.6 TYPES OF MACHINE LEARNING
-
5.1.7 TYPES OF MACHINE LEARNING
-
5.1.8 TYPES OF MACHINE LEARNING
-
5.2 MULTIPLE REGRESSION
-
5.2.1 MULTIPLE REGRESSION
-
5.2.2 MULTIPLE REGRESSION
-
5.2.3 MULTIPLE REGRESSION
-
5.2.4 MULTIPLE REGRESSION
-
5.2.5 MULTIPLE REGRESSION
-
5.2.6 MULTIPLE REGRESSION
-
5.2.7 MULTIPLE REGRESSION
-
5.3 KNN INTRO
-
5.3.1 KNN ALGORITHM
-
5.3.2 KNN ALGORITHM
-
5.3.3 INTRODUCTION TO CONFUSION MATRIX
-
5.3.4 INTRODUCTION TO SPLITTING THE DATASET USING TRAINTESTSPLIT
-
5.3.5 KNN ALGORITHM
-
5.3.6 KNN ALGORITHM
-
5.4 INTRODUCTION TO DECISION TREE
-
5.4.1 INTRODUCTION TO DECISION TREE
-
5.4.2 DECISION TREE ALGORITHM
-
5.4.3 DECISION TREE ALGORITHM
-
5.4.4 DECISION TREE ALGORITHM
-
5.5 UNSUPERVISED LEARNING
-
5.5.1 UNSUPERVISED LEARNING
-
5.5.2 UNSUPERVISED LEARNING
-
5.5.3 UNSUPERVISED LEARNING
-
5.5.4 AHC ALGORITHM
-
5.5.5 AHC ALGORITHM
-
5.6 KMEANS CLUSTERING
-
5.6.1 KMEANS CLUSTERING
-
5.6.2 KMEANS CLUSTERING
-
5.6.3 DBSCAN ALGORITHM
-
5.6.4 DBSCAN PROGRAM
-
5.6.5 DBSCAN PROGRAM
Course/Topic 13 - Deep Learning Foundation - all lectures
-
In this session we will learn about the introduction to Deep Learning. This video talks about Deep Learning as a series introduction and what is a neural network. Furthermore, we will talk about the 3 reasons to go deep and your choice of Deep net.
-
In this video tutorial we will discuss about the neural networks and the 3 reasons to go Deep. Further we will also learn about the use of GPU in artificial intelligence and your choice of deep net.
-
In this session we will learn about the deep learning models basics. After this video you will be able to understand the concept of restricted Boltzmann machines and deep belief network. Furthermore, you will learn about the convolution neural network and recurrent neural network.
-
In this video course further topics of Deep learning models. After this video you will be able to understand the convolution neural network and its characteristics in detail.
-
In this video course further topics of Deep learning models. After this video you will be able to understand the recurrent neural network and its characteristics.
-
In this session the tutor talks about the basic Additional Deep Learning Models. In this video you will learn about Auto encoders, Recursive neural tensor network and generative adversarial networks
-
This session is in continuation to the previous session. In this video we will learn about the Recursive Neural Tensor Network in detail and hierarchical structure of data.
-
In this Additional Deep Learning Models tutorial, we will proceed with the Generative Adversarial Networks (GAN) and its uses.
-
In this video the tutor explains the Platforms and Libraries of Deep Learning. We will start with what is a deep net platform, H2O.ai and Dato Graph Lab. Further we will see what is a Deep Learning Library and Theano and Caffe. We will also cover a bit of Keras and TensorFlow.
-
This tutorial will cover the further part of DatoGraph Lab and its history. Further we wil see the benefits and uses of DatoGraph Lab.
-
This tutorial will cover the further part of DatoGraph Lab and its history.
-
In this video we will cover the further topics of Deep Learning platform and Libraries such as what is a Deep Learning Library? when and how to use Theano and Caffe as Deep Learning Library.
-
In previous video we have leant about Theano and Caffe Deep Learning Library. In this video we will learn about the TensorFlow (free and open source library) as a Deep Learning Library and building Deep Learning Models.
-
In this video we will learn about the last type of Library i.e. Keras. Keras is an open source neural network library and runs on top of Theano or TensorFlow. We will further see the advantages of Keras in Deep Learning.
Course/Topic 14 - Cloud Computing Basics - all lectures
-
In this lecture session we learn about cloud computing, which means storing and accessing data over the internet instead of a hard disk. It is defined as a service that provides users to work over the internet.
-
In this lecture session we learn about cloud computing is the delivery of computing services—including servers, storage, databases, networking, software, analytics, and intelligence—over the Internet (“the cloud”) to offer faster innovation, flexible resources, and economies of scale.
-
In this lecture session we learn about Cloud computing is a general term for anything that involves delivering hosted services over the internet. These services are divided into three main categories or types of cloud computing.
-
In this tutorial we learn about the importance of cloud computing can be found in using services like Google Drive and Apple iCloud. The use of these services allows documents, contacts, pictures, and a whole lot more online.
The career path of a Data Science Manager typically involves progression through various roles within the field of data science and analytics. Here's a general overview of the career path and potential job prospects for a Data Science Manager:
Career Path
-
Data Analyst/Intern
- Entry-level position focusing on foundational data analysis skills.
-
Data Scientist
- Develops advanced analytical skills, engages in statistical modeling, machine learning, and data visualization.
-
Senior Data Scientist
- Takes on more complex projects and responsibilities, often involved in mentoring junior team members.
-
Lead Data Scientist
- Leads projects and teams, responsible for project outcomes and team performance.
-
Data Science Manager
- Takes on a managerial role, overseeing multiple teams or a department.
- Responsible for setting the strategic direction for data science initiatives.
-
Director of Data Science
- Provides strategic leadership for the entire data science function within an organization.
-
Head of Data Science or Chief Data Scientist
- Highest-ranking data science role in many organizations, responsible for the overall success of data science initiatives.
Job Prospects
-
Data Science Manager
- As a Data Science Manager, job prospects are abundant as organizations increasingly recognize the value of data-driven decision-making.
-
Head of Analytics
- Some professionals in this role may take on broader responsibilities, overseeing not only data science but also analytics across various domains.
-
Director of Data Strategy
- Individuals may transition into roles focused on shaping and implementing overall data strategies for organizations.
-
Chief Data Officer (CDO)
- The role of a Chief Data Officer involves a strategic approach to managing and leveraging data assets across an organization.
-
Consultant/Advisor
- Experienced Data Science Managers may transition into consultancy roles, providing guidance to other organizations on their data science strategies.
-
Executive Leadership
- Depending on the organizational structure, Data Science Managers may ascend to executive leadership roles, such as Chief Analytics Officer or Chief Information Officer.
-
Entrepreneurship
- Some professionals choose to start their own data science consulting firms or tech startups, leveraging their expertise and leadership skills.
Skills Enhancement
To enhance job prospects and stay competitive in the field, Data Science Managers should continuously:
- Stay updated on emerging technologies and trends in data science.
- Expand leadership and management skills through courses or certifications.
- Foster a strong professional network within the data science community.
- Contribute to industry publications, attend conferences, and participate in relevant forums.
- Pursue advanced degrees or certifications in business or data-related fields.
Job prospects for Data Science Managers are promising, given the increasing reliance on data-driven insights across industries. Continuous learning and a proactive approach to professional development are key to staying at the forefront of this dynamic field. Additionally, building a strong professional network and staying engaged with industry advancements can open up various opportunities in the evolving landscape of data science and analytics.
Some of the key Data Science Manager role interview questions are given below.
1. What experience do you have in leading and managing data science teams?
I have X years of experience in leading and managing data science teams in various industries, including [mention specific industries]. I have successfully overseen projects from inception to deployment, mentored team members, and ensured alignment with business goals.
2. Can you describe your approach to building and structuring a data science team?
My approach involves assessing the organization's needs, defining clear roles and responsibilities, identifying necessary skill sets, fostering a collaborative environment, and promoting continuous learning and development among team members.
3. How do you prioritize and allocate resources within a data science team to meet project deadlines and objectives?
I prioritize projects based on their strategic importance, resource availability, and potential impact on the organization. I collaborate with stakeholders to establish clear objectives, timelines, and deliverables and regularly monitor progress to ensure alignment with deadlines and objectives.
4. What strategies do you employ to foster collaboration between data science teams and other departments within the organization?
I encourage open communication, cross-functional collaboration, and knowledge sharing between data science teams and other departments. I organize regular meetings, workshops, and brainstorming sessions to facilitate collaboration and ensure alignment with business objectives.
5. How do you ensure that data science projects are aligned with the organization's overall goals and objectives?
I work closely with key stakeholders to understand business needs, identify opportunities for leveraging data science, and define clear project objectives and success criteria. I regularly communicate progress and outcomes to ensure alignment with organizational goals.
6. Can you describe a challenging project you managed as a data science manager and how you addressed any obstacles or setbacks?
Certainly. In a previous role, we faced challenges with data quality and availability, which impacted project timelines. To address this, I collaborated with IT and data engineering teams to improve data collection processes and implemented quality assurance measures to ensure reliable insights.
7. How do you stay updated on the latest trends and advancements in data science and technology?
I actively participate in industry conferences, webinars, and workshops, engage with professional networks, and encourage continuous learning and development within my team. I also leverage online resources, research papers, and academic publications to stay informed about emerging trends and advancements.
8. What metrics or KPIs do you use to measure the performance and effectiveness of your data science team?
Key metrics and KPIs I use include project delivery timelines, accuracy of predictive models, business impact of data-driven insights, team productivity, and employee satisfaction and retention rates.
9. How do you handle conflicts or disagreements within your data science team?
I address conflicts or disagreements openly and constructively, fostering a culture of respect, empathy, and collaboration. I encourage team members to voice their concerns, listen actively to different perspectives, and facilitate consensus-building discussions to resolve conflicts.
10. Can you provide an example of a successful data science project you managed, including its impact on the organization?
One notable project I managed involved developing a predictive analytics model to optimize inventory management. By accurately forecasting demand and identifying supply chain inefficiencies, we reduced inventory costs by X% and improved on-time delivery rates by X%.
11. How do you ensure that your data science team maintains high ethical standards and complies with relevant regulations?
I emphasize the importance of ethical conduct and regulatory compliance through training, awareness programs, and clear policies and guidelines. I also conduct regular audits and reviews to ensure adherence to ethical principles and legal requirements.
12. What strategies do you employ to attract and retain top talent in the data science field?
I offer competitive compensation packages, opportunities for professional growth and development, a supportive work environment, and recognition for achievements. I also promote a culture of innovation, collaboration, and diversity to attract and retain top talent.
13. How do you approach performance evaluations and feedback sessions with your data science team members?
I conduct regular performance evaluations based on predefined goals and objectives, providing constructive feedback and coaching to support professional growth and development. I encourage two-way communication and set clear expectations for performance improvement when necessary.
14. What steps do you take to ensure that data science projects are scalable and can be deployed effectively across the organization?
I collaborate with IT and infrastructure teams to design scalable architectures and workflows, prioritize modular and reusable components, and document best practices and standards for deployment. I also conduct thorough testing and validation to ensure reliability and scalability.
15. How do you manage risks associated with data privacy, security, and compliance in data science projects?
I work closely with legal, compliance, and cybersecurity teams to identify potential risks and implement appropriate safeguards, such as data encryption, access controls, anonymization techniques, and compliance with relevant regulations such as GDPR and CCPA.
16. Can you describe your experience in implementing machine learning models in production environments?
I have experience in deploying machine learning models in production environments using containerization technologies, orchestration platforms, and continuous integration/continuous deployment (CI/CD) pipelines. I ensure robust monitoring, version control, and scalability to support real-time inference and decision-making.
17. How do you assess the ROI (Return on Investment) of data science initiatives and communicate their value to senior management?
I quantify the ROI of data science initiatives by measuring their impact on key business metrics such as revenue growth, cost savings, customer satisfaction, and operational efficiency. I present findings using clear and compelling data-driven narratives to senior management, demonstrating the value of data science investments.
18. What role do you see data science playing in driving innovation and competitive advantage for organizations?
Data science plays a critical role in driving innovation and competitive advantage by uncovering actionable insights, identifying market trends, optimizing processes, and enhancing decision-making across all business functions.
19. How do you approach building and maintaining partnerships with external vendors, research institutions, or industry collaborators?
I establish and nurture strategic partnerships with external vendors, research institutions, and industry collaborators to leverage complementary expertise, access cutting-edge technologies, and stay ahead of industry trends. I prioritize transparency, mutual respect, and shared goals in all partnerships.
20. Can you share your experience in managing data science projects with tight deadlines and high stakes?
In my previous role, I managed a data science project with a tight deadline for regulatory compliance. I implemented agile methodologies, streamlined workflows, and allocated resources effectively to meet the deadline while maintaining high-quality standards and ensuring compliance with regulations.
21. How do you assess the business impact and value proposition of potential data science projects before initiating them?
I conduct thorough feasibility studies, stakeholder interviews, and cost-benefit analyses to assess the business impact and value proposition of potential data science projects. I prioritize projects based on their alignment with strategic objectives, potential ROI, and feasibility of implementation.
22. What strategies do you employ to ensure that data science projects are aligned with customer needs and preferences?
I actively engage with customers and stakeholders throughout the project lifecycle, gathering feedback, identifying pain points, and aligning project objectives with customer needs and preferences. I prioritize user-centric design and usability testing to ensure customer satisfaction and adoption.
23. How do you approach building a culture of innovation and experimentation within your data science team?
I foster a culture of innovation and experimentation by encouraging curiosity, risk-taking, and learning from failures. I allocate time and resources for research and development projects, hackathons, and innovation challenges to explore new ideas and technologies.
24. Can you discuss a time when you had to make difficult decisions as a data science manager, and how you handled the situation?
In a previous role, I had to make a difficult decision to reallocate resources from a low-impact project to a high-impact initiative with greater strategic importance. I communicated openly with stakeholders, justified the decision based on data-driven insights, and ensured a smooth transition to minimize disruption.
25. How do you balance short-term project goals with long-term strategic initiatives as a data science manager?
I prioritize short-term project goals to deliver immediate value and meet business needs while also allocating resources towards long-term strategic initiatives that align with the organization's vision and objectives. I regularly reassess priorities and adjust resource allocation as needed to maintain alignment with strategic goals.
26. What measures do you take to ensure diversity, equity, and inclusion within your data science team?
I actively promote diversity, equity, and inclusion within my team by fostering a welcoming and inclusive work environment, recruiting and retaining diverse talent, providing opportunities for professional growth and advancement, and addressing biases and stereotypes through awareness and education.
27. How do you handle situations where data science projects encounter unexpected challenges or roadblocks?
I approach unexpected challenges or roadblocks with a problem-solving mindset, seeking input from team members, stakeholders, and subject matter experts to identify solutions and mitigate risks. I communicate transparently with all stakeholders and adjust project plans as necessary to overcome obstacles and achieve objectives.
28. Can you discuss your experience in leading cross-functional teams on complex data science projects?
I have led cross-functional teams on complex data science projects that involved collaboration with departments such as IT, marketing, finance, and operations. I facilitated communication, managed dependencies, and promoted alignment with project goals to ensure successful outcomes.
29. How do you ensure that your data science team stays motivated and engaged, especially during challenging projects?
I recognize and celebrate team achievements, provide opportunities for skill development and career growth, solicit feedback and input from team members, and promote a positive work-life balance to foster motivation and engagement.
30. What role does storytelling play in communicating data-driven insights and recommendations to non-technical stakeholders?
Storytelling plays a crucial role in communicating data-driven insights by transforming complex information into compelling narratives that resonate with non-technical stakeholders. I use visualizations, anecdotes, and real-world examples to convey insights in a clear and accessible manner.
31. How do you handle situations where there is disagreement between data-driven insights and intuition or prior beliefs?
I approach disagreements between data-driven insights and intuition with open-mindedness and curiosity, seeking to understand underlying assumptions and motivations. I encourage constructive debate, evidence-based decision-making, and empirical validation to reconcile differences and reach consensus.
32. Can you discuss your experience in implementing data governance frameworks and best practices within organizations?
I have experience in implementing data governance frameworks and best practices to ensure data quality, integrity, and security within organizations. This involves establishing policies, procedures, and controls for data management, access, and usage, as well as promoting a culture of data stewardship and accountability.
33. How do you approach building partnerships and collaborations with external stakeholders such as vendors, clients, or research institutions?
I approach building partnerships and collaborations with external stakeholders by identifying mutual goals and interests, establishing clear communication channels, fostering trust and transparency, and ensuring alignment with strategic objectives and values.
34. Can you discuss a time when you had to advocate for additional resources or support for a data science project, and how you approached the situation?
In a previous role, I advocated for additional resources for a data science project by presenting a business case that outlined the potential ROI, strategic importance, and resource requirements. I engaged with senior management, addressed concerns, and negotiated for necessary support to ensure project success.
35. How do you ensure that data science projects are aligned with legal and regulatory requirements, especially in sensitive areas such as privacy or security?
I work closely with legal, compliance, and cybersecurity teams to understand and address legal and regulatory requirements relevant to data science projects. This includes implementing data anonymization techniques, access controls, and encryption measures to protect sensitive information and ensure compliance with laws such as GDPR and HIPAA.
36. What strategies do you employ to foster a culture of continuous learning and development within your data science team?
I encourage continuous learning and development within my team by providing access to training resources, certifications, workshops, and conferences. I support participation in research projects, industry events, and online communities to stay updated on emerging trends and technologies.
37. How do you approach delegating tasks and responsibilities within your data science team?
I delegate tasks and responsibilities based on team members' skills, interests, and availability, while also considering project priorities and deadlines. I provide clear instructions, set expectations, and empower team members to take ownership of their work while offering guidance and support as needed.
38. Can you discuss your experience in leading data-driven initiatives that have resulted in measurable business impact?
I have led data-driven initiatives that have resulted in measurable business impact, such as revenue growth, cost savings, process efficiencies, and improved customer satisfaction. By leveraging data science techniques and insights, we have been able to drive strategic decision-making and achieve tangible results for the organization.
39. How do you approach building trust and credibility with stakeholders who may be skeptical of data-driven approaches or unfamiliar with data science concepts?
I approach building trust and credibility with stakeholders by demonstrating the value of data-driven approaches through clear communication, tangible results, and real-world examples. I listen to stakeholders' concerns, address misconceptions, and tailor messaging to their needs and preferences to foster understanding and buy-in.
40. Can you discuss your experience in managing data science projects with cross-border or international teams?
I have managed data science projects with cross-border or international teams, which requires effective communication, cultural sensitivity, and coordination across different time zones and languages. I promote inclusivity, respect diversity, and leverage technology tools to facilitate collaboration and overcome geographical barriers.
41. How do you approach building a roadmap for data science initiatives that align with the organization's strategic priorities?
I collaborate with senior leadership and key stakeholders to understand the organization's strategic priorities, identify opportunities for leveraging data science, and define clear objectives and milestones for data science initiatives. I develop a roadmap that outlines project timelines, resource requirements, and expected outcomes to ensure alignment with strategic goals.
42. What measures do you take to ensure that data science projects are scalable and sustainable in the long term?
I design data science projects with scalability and sustainability in mind by leveraging modular architectures, standardized processes, and automation tools. I prioritize documentation, knowledge sharing, and training to empower team members and ensure continuity of operations over time.
43. Can you discuss your experience in leading data science teams through organizational change or transition periods?
I have led data science teams through organizational change or transition periods by providing clarity, stability, and support during times of uncertainty. I communicate transparently, address concerns proactively, and emphasize the team's role in driving positive outcomes amidst change.
44. How do you approach building and maintaining relationships with internal stakeholders such as executives, business leaders, and department heads?
I approach building and maintaining relationships with internal stakeholders by understanding their needs and priorities, communicating effectively, delivering value through data-driven insights, and building trust and credibility through consistent performance and reliability.
45. Can you discuss your experience in developing data-driven strategies to address complex business challenges or opportunities?
I have experience in developing data-driven strategies to address complex business challenges or opportunities, such as market expansion, customer segmentation, product optimization, and risk management. By leveraging data science techniques and analytics, we have been able to formulate actionable insights and drive strategic decision-making.
46. How do you approach managing competing priorities and demands from multiple stakeholders within the organization?
I prioritize competing priorities and demands by assessing their strategic importance, resource requirements, and potential impact on the organization. I collaborate with stakeholders to negotiate timelines, set expectations, and allocate resources effectively to ensure alignment with organizational goals.
47. What steps do you take to ensure that data science projects are aligned with industry best practices and standards?
I stay updated on industry best practices and standards through continuous learning and engagement with professional networks and communities. I incorporate relevant methodologies, frameworks, and tools into project workflows and ensure compliance with established standards and guidelines.
48. Can you discuss your experience in managing data science projects with interdisciplinary teams or external consultants?
I have managed data science projects with interdisciplinary teams or external consultants, which requires effective communication, coordination, and collaboration across different expertise areas. I establish clear roles and responsibilities, facilitate knowledge sharing, and foster a collaborative environment to drive successful project outcomes.
49. How do you approach building a diverse and inclusive data science team, and why is diversity important in the field of data science?
I prioritize diversity and inclusion in hiring and team building by actively seeking candidates from diverse backgrounds, experiences, and perspectives. Diversity is important in data science because it fosters creativity, innovation, and empathy, leading to better problem-solving and decision-making outcomes.
50. What do you see as the future trends and challenges in the field of data science, and how do you prepare your team to adapt and thrive in a rapidly evolving landscape?
I see future trends and challenges in data science including advancements in artificial intelligence, machine learning, automation, and ethics. To prepare my team, I encourage continuous learning, experimentation, and adaptation to new technologies and methodologies, while also fostering a culture of resilience, agility, and collaboration to navigate uncertainties and seize opportunities in the evolving landscape.