Machine Learning (basic to advanced)
Learn about Statistics, Artificial Intelligence, Deep Learning and Data mining.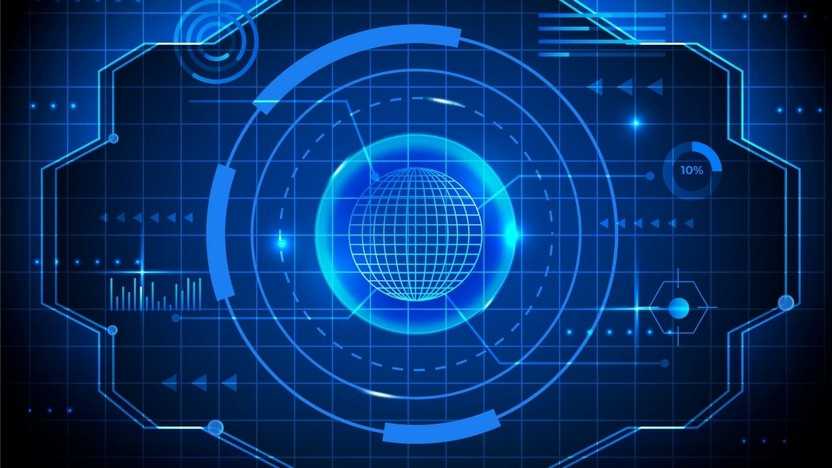
90% Started a new career BUY THIS COURSE (
USD 17 USD 41 )-
95% Got a pay increase and promotion
Students also bought -
-
- Career Path - Artificial Intelligence & Machine Learning Engineer
- 220 Hours
- USD 45
- 5212 Learners
-
- Data Science with Python
- 45 Hours
- USD 17
- 2931 Learners
-
- R Programming (basic to advanced)
- 20 Hours
- USD 17
- 361 Learners
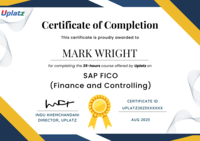
Machine Learning provides machines with the ability to learn autonomously based on experiences, observations, and analyzing patterns within a given data set without explicitly programming. When we write a program or a code for some specific purpose, we are actually writing a definite set of instructions that the machine will follow.
Whereas in machine learning, we input a data set through which the machine will learn by identifying and analyzing the patterns in the data set and learn to take decisions autonomously based on its observations and learnings from the dataset. The first step in machine learning basics is that we feed knowledge/data to the machine; this data is divided into two parts namely, training data and testing data.
Python is an easy to learn, powerful programming language. You can use Python when your data analysis tasks need to be integrated with web apps or if statistics code needs to be incorporated into a production database. Machine Learning can be an incredibly beneficial tool to uncover hidden insights and predict future trends. This Machine Learning with Python course will give you all the tools you need to get started with supervised and unsupervised learning.
Some of the practical applications of ML include:
1. Predictive Maintenance
Industry: Manufacturing, Energy
Application: Predicting equipment failures and scheduling maintenance to prevent downtime.
2. Fraud Detection
Industry: Finance, Banking
Application: Identifying unusual patterns and detecting fraudulent transactions.
3. Personalized Marketing
Industry: Retail, E-commerce
Application: Tailoring marketing campaigns based on customer behavior and preferences.
4. Recommendation Systems
Industry: Entertainment, E-commerce
Application: Suggesting products, movies, or music based on user preferences and past behavior.
5. Customer Support Automation
Industry: Various
Application: Using chatbots and virtual assistants to handle customer queries and support.
6. Image and Speech Recognition
Industry: Technology, Healthcare
Application: Facial recognition for security, medical image analysis, voice-activated assistants.
7. Natural Language Processing (NLP)
Industry: Healthcare, Legal, Customer Service
Application: Sentiment analysis, automated legal document review, and conversational AI.
8. Autonomous Vehicles
Industry: Automotive
Application: Self-driving cars and drones for transportation and delivery services.
9. Predictive Analytics in Healthcare
Industry: Healthcare
Application: Predicting disease outbreaks, patient risk assessment, and personalized treatment plans.
10. Supply Chain Optimization
Industry: Logistics, Retail
Application: Forecasting demand, optimizing inventory levels, and improving delivery routes.
This Machine Learning course will help you become a Machine Learning Engineer and/or a Data Scientist. At the end of the course you will receive a course completion certificate issued by Uplatz.
Course/Topic - Machine Learning (basic to advanced) - all lectures
-
In this session we will learn about introduction to Machine Learning. We will start by learning about the basics of Linear Algebra required to learn Machine Language. Further we will learn about Linear equations represented by Matrices and Vectors.
-
In this module we will learn about the computational roots of matrices. We will learn how to multiply matrix with scalar and vector. We will learn about addition and subtraction of matrices.
-
In this module we will learn about Num-Pie Linear Algebra to work on Python. It further includes the understanding of the use of functions - #dot, #vdot, #inner, #matmul, #determinant, #solve, #inv. Basic examples of the #dot, #vdot functions will be discussed.
-
In this module we will learn about how the #inner function work in a two-dimension array. We will also learn its usage in #dot and #vdot. We will see explanation of the functions solving examples.
-
In this module we will learn about using #matmul function. We will learn about normal product and stack of arrays. We will also learn how to check the dimensions of the array and how to make it compatible.
-
In this module we will learn about the #determinant function. The basics of the #determinant function will be explained. Examples will be solved with explanations to understand it.
-
In this module we will learn what a Determinant is. We will also learn about how to find a Determinant. We will further learn how to find the Determinant of a 2*2 and 3*3 matrix learn about the basics of #inv function.
-
In this module we will learn about the #inv function. We will learn about how to find the inverse of a matrix. We will also learn how to find the Identity matrix for the inverse.
-
In this module we will discuss about the inverse of a matrix. We will understand what an Inverse is. We will further learn how the Inverse of a matrix is found.
-
In this module we will learn about the difference of the dot( ) and the inner( ). We will see examples of dot( ) and inner( ), We will also learn about the dissimilarities between the dot( ) and inner( ) with the help of examples.
-
In this module we will learn about numpy matrix. We will learn the different ways of creating a matrix. We will also learn about a vector as a matrix and its multiplication with matrix.
-
In this module we will learn about the #numpy.vdot( ) function. This module is a continuation of the previous module. We will also learn about the #numpy,inner( ) function.
-
In this module we will understand the different concepts like Rank, Determinant, Trace, etc, of an array. Then we will learn how to find the item value of a matrix. We will also learn about the matrix and vector products.
-
In this module we will learn about the matrix and vector products. We will learn about how it works on imaginary and complex numbers. We will also get an understanding of matmul( ), inner( ), outer( ), linalg.multi_dot( ), tensordot( ), einsom( ), einsum_path( ),linalg.matrix_power( ).
-
In this module we will learn about the basics of #inverse of a matrix. We will understand what an Inverse is. We will also see examples of inverse of a matrix and learn how to calculate it.
-
In this module we will learn about the basics of Python. We will also learn about the Packages needed by the machine language. We will further learn the basics of numpy, scipy, pandas, skikit-learn, etc. needed machine learning and data science.
-
In this module we will understand about SciPy. We will also learn about SkiKit-learn and Theano. We will further learn about TensorFlow, Keras, PyTorch, Pandas, Matplotlib.
-
In this module we will see examples of the topics discussed in the previous module. We will also start the basics of Python. We will also solve some basic problems.
-
In this module we will continue the basic problems of Python. We will also understand about Operators. We will also see the different operators and its applications.
-
In this module we will continue learning the different Operators. We will also learn about Advanced Data types. We will learn and understand the different data types and about Sets.
-
In this module we will learn about list. We will see the different functions of list. We will also learn about Jupyter notebook.
-
In this module we will learn about #condition statements in Python in brief. We will also learn about the applications of #condition statements We will solve some examples to understand the #condition statements.
-
In this module we will learn about the Loop in Pyhton. We will also learn about the different kinds of loops. We will see examples of For loop, and break keyword.
-
In this module we will continue with the #for loop. We will also learn about the continue keyword. We will solve examples for the usage of the keywords.
-
In this module we will learn about Functions in Python. We will solve examples using different functions. We will understand how functions work.
-
In this module we will learn about arguments in functions. We will also solve examples to understand the usage of arguments in functions. We will also learn about #call by reference in Python.
-
In this module we will learn about strings. We will also learn about types of arguments for functions in python. We will also see the usage of the different types of arguments.
-
In this module we will learn about default arguments. We will also learn about variable arguments. We will solve examples to understand it better.
-
In this module we will learn about the remaining arguments. We will understand about default and variable arguments better. We will also learn about keyword arguments.
-
In this module we will learn about built-in functions. We will also learn about the different built-in functions in python. We will solve examples to understand the functions better.
-
In this module we will continue the previous functions. We will also learn about other built-in functions. We will also learn about bubble sort in python.
-
In this module we will learn about the scope of variable in function. We will also learn about the different variables and its usage. We will solve examples using the different variables to understand it better.
-
In this module we will learn about the math module in python. We will learn about the different inbuilt functions that deal with math functions. We will solve problems using the different math functions.
-
In this module we will continue with the previous lecture. We will also learn about the different arguments in functions. We will also learn about call by reference in python.
-
In this module we will continue with the previous lecture. We will also start mathplotlib in python. We will learn the different types of mathplotlib by using jupyter.
-
In this module we will learn about loan calculator using tkinter. We will also learn how to use the loan calculator. We will solve an example to understand its usage.
-
In this module we will continue with the previous lecture. We will learn how to compute payments using functions. We will also learn about the function getmonthlypayment.
-
In this module we will learn about numpy function. We will also learn about mathematical and logical operations using numpy. We will also be explained about different numpy arrays.
-
In this module we will continue with the previous lecture. We will learn about different numpy attributes. We will solve examples using the different attributes and slicing an array.
-
In this module we will learn about advanced slicing of an array. We will use jupyter to do array slicing. We will understand detail how array slicing works.
-
In this module we will learn about using jupyter notebook online. We will also learn about ranges. We will learn about creating arrays from ranges. We will also learn about linear space.
-
In this module we will learn about the average function. We will also learn about the different averages. We will solve examples to understand the function.
-
In this module we will learn about generating random strings and passwords. We will also learn about generating a string of lower and upper case letters. We will solve examples using the different strings.
-
In this module we will learn about generating strings. We will also learn about upper case letters and only printing specific letter. We will also learn about alpha numeric letters.
-
In this module we will learn about the unique function. We will continue using arrays. We will solve example using unique functions in arrays.
-
In this module we will learn about array manipulation function, We will learn about the delete function in numpy. We will solve examples for better understanding.
-
In this module we will learn about the insert function in numpy. We will also learn about flattened array. We will solve examples.
-
In this module we will learn about examples with two dimension arrays. We will also learn about the ravel function. We will also learn about the rollaxis function, swapaxes function.
-
In this module we will learn about statistical functions. We will also learn about min and max values. We will solve examples using the functions.
-
In this module we will learn about functions for rounding. We will also learn about round off function, floor function and ceil function. We will solve examples using the functions.
-
In this module we will learn about numpy append function. We will also learn about resize function. We will solve examples.
-
In this module we will learn about numpy nonzero function. We will also learn about the where function. We will solve examples using the different functions.
-
In this module we will learn about matrix library. We will also learn about the different matlib functions We will solve different examples using the matlib function. vvvv
-
In this module we will learn about the basic operations that can be done on numpy arrays. We will also learn about arithmetic operations and functions. We will do examples with arithmetic operations.
-
In this module we will learn about numpy filter array. We will do programs on numpy filter array. We will solve examples using the filter array.
-
In this module we will learn about array manipulation functions. We will see how the array manipulation functions work. We will learn about the different manipulation functions.
-
In this module we will learn about broadcasting function in numpy. We will also learn about reshape in numpy. We will also learn about removing function in numpy.
-
In this module we will learn about indexing. We will also learn about slicing. We will solve examples to understand the concept.
-
In this module we will learn about numpy append function. We will also learn about resize function. We will solve examples using the functions.
-
In this module we will learn about conversion of numpy dtypes to native python types. We will also learn to create 4*4 matrix in which 0 and 1 are staggered with zero on the main diagonal. We will also learn to create 10*10 matrix elements on the borders will be equal to 1 and inside 0.
-
In this module we will learn how to use a python program to find the maximum and minimum value of a flattened array. We will also see the function called flat and flatten to make the array flattened. We will learn about function import numpy as np and array-np.arrange( )
-
In this module we will learn how to generate a random string of a given length. Tutor will address the issues faced in generating random strings. Further in the video, we will discuss the various ways in which generation of a random staring can be performed.
-
In this video we will be covering on creating a simple project. We will see the practical on how tutor creates a simple project. We will also see some examples on how to create a simple project. The video talks about how to get common items between 2 python numpy arrays.
-
In this video we will talk about another function in python programming called the split function. The function split divides the arrays into sub arrays. The split() method splits a string into an array of substrings. The split() method returns the new array. The split() method does not change the original string. If (" ") is used as separator, the string is split between words.
-
This video is a sequel of explanation of spilt function. We will discuss the three types of split functions – 1. Normal split, 2. Horizontal split and 3. Vertical Split. Further we will discuss the roles of split function and what do they do.
-
In this video we will learn about the numpy filter array. We will further see what is filtering of array. Getting some elements out of an existing array and creating a new array out of them is called filtering of array, using a bullion index list.
-
In this video we will learn about an important topic in Python, i.e Python file handling. We will see what is a file and the type of executable files. Further we will see what is output and how to view the output. Different access modes that can be opened with the file.
-
In this video we will see an example on how to open and file in view mode, by giving the name of the file. File statement in Python is used in exception handling to make the code cleaner and much more readable. It simplifies the management of common resources like file streams. ... with open ( 'file_path' , 'w' ) as file : file .
-
This video is a continuation of file system tutorial. Here we will see to use the append mode and what is append mode. Python has a built-in open() function to open a file. This function returns a file object, also called a handle, as it is used to read or modify the file accordingly. We can specify the mode while opening a file. In mode, we specify whether we want to read r , write w or append a to the file.
-
In this module we will start a new topic known as random module which is a very important part in numpy. Further we will discuss the functionalities of random module to generate random numbers.
-
In this module we will see how to generate the arrays on float and hot generate a single floating value from 0 to 1. Further we will see taking array as a parameter and randomly return one of the values.
-
In this module we will learn the random module in continuations. The random is the module present in the numpy library. This module contains simple random generation methods.
-
In this module how random module contains functions used to generate random numbers. We will also see some permutations and distribution functions.
-
In this module we will see the choice functions and the different variants of choice function. Further we will see how to randomly select multiple choices from the list. Random.sample or random.choices are the functions used to select multiple choices or set.
-
In this module we will see the difference between the sample function and the choices functions. Further, we will do a random choice from asset with Python, by converting it to tuple.
-
In this module we will learn about the random Boolean in Python, using random.choice. In order to generate Random boolean, we use the nextBoolean() method of the java. util. Random class. This returns the next random boolean value from the random generator sequence
-
In this module we will learn about the library available in python that is called Pandas. We will see how Pandas is one of the important tools available in Python. Further we will see how Pandas makes sense to list the things.
-
In this module we will learn about the basics of Pandas. Further we will see how this an important tool for Data scientist and Analysts and how pandas is the back bone of most of the data projects.
-
This module is a sequel of the previous tutorial on Pandas. In this module we will see practical project on pandas using series and dataframes. Lastly we will learn how to handle duplicate and how to handle information method and shape attribute.
-
In this video we will see about column clean and how to clean the column. Further we will see how to rename the columns by eliminating symbols and other different ways.
-
In this module we will learn about how to work with the missing values or null values. Further we will see if the dataset is inconsistent or has some missing values then how to deal with the missing values when exploring the data.
-
In this video we will see how to perform the imputation on column, i.e., metascore which has some null values. Further we will see how to use describe function on the genre column of the dataset.
-
In this module we will learn about the frequency of columns. Further we will see about the functio0n called value counts. The value counts function when used on the genre column tells us the frequency of all the columns.
-
In this video we will learn about the methods of slicing, selecting and extracting. If these methods are not followed properly then we will receive attribute errors. Further we will learn to manipulate and extract data using column headings and index locations.
-
2.7 MATPLOTLIB BASICS
-
2.7.1 MATPLOTLIB BASICS
-
2.7.2 MATPLOTLIB BASICS
-
2.7.3 MATPLOTLIB BASICS
-
2.7.4 MATPLOTLIB BASICS
-
2.7.5 MATPLOTLIB BASICS
-
2.7.6 MATPLOTLIB BASICS
-
2.7.7 MATPLOTLIB BASICS
-
2.7.8 MATPLOTLIB BASICS
-
2.7.9 MATPLOTLIB BASICS
-
2.7.9.1 MATPLOTLIB BASICS
-
2.7.9.11 MATPLOTLIB BASICS
-
2.8 AGE CALCULATOR APP
-
2.8.1 AGE CALCULATOR APP
-
2.8.2 AGE CALCULATOR APP
-
2.8.3 AGE CALCULATOR APP
-
3.1 MACHINE LEARNING BASICS
-
3.1.1 MACHINE LEARNING BASICS
-
3.1.2 MACHINE LEARNING BASICS
-
3.1.3 MACHINE LEARNING BASICS
-
3.1.4 MACHINE LEARNING BASICS
-
3.1.5 MACHINE LEARNING BASICS
-
3.1.6 MACHINE LEARNING BASICS
-
3.1.7 MACHINE LEARNING BASICS
-
3.1.8 MACHINE LEARNING BASICS
-
3.1.9 MACHINE LEARNING BASICS
-
3.1.9.1 MACHINE LEARNING BASICS
-
3.2 MACHINE LEARNING BASICS
-
4.1 TYPES OF MACHINE LEARNING
-
4.1.1 TYPES OF MACHINE LEARNING
-
4.1.2 TYPES OF MACHINE LEARNING
-
4.1.3 TYPES OF MACHINE LEARNING
-
4.1.4 TYPES OF MACHINE LEARNING
-
4.1.5 TYPES OF MACHINE LEARNING
-
4.1.6 TYPES OF MACHINE LEARNING
-
5.1 TYPES OF MACHINE LEARNING
-
5.1.1 TYPES OF MACHINE LEARNING
-
5.1.2 TYPES OF MACHINE LEARNING
-
5.1.3 TYPES OF MACHINE LEARNING
-
5.1.4 TYPES OF MACHINE LEARNING
-
5.1.5 TYPES OF MACHINE LEARNING
-
5.1.6 TYPES OF MACHINE LEARNING
-
5.1.7 TYPES OF MACHINE LEARNING
-
5.1.8 TYPES OF MACHINE LEARNING
-
5.2 MULTIPLE REGRESSION
-
5.2.1 MULTIPLE REGRESSION
-
5.2.2 MULTIPLE REGRESSION
-
5.2.3 MULTIPLE REGRESSION
-
5.2.4 MULTIPLE REGRESSION
-
5.2.5 MULTIPLE REGRESSION
-
5.2.6 MULTIPLE REGRESSION
-
5.2.7 MULTIPLE REGRESSION
-
5.3 KNN INTRO
-
5.3.1 KNN ALGORITHM
-
5.3.2 KNN ALGORITHM
-
5.3.3 INTRODUCTION TO CONFUSION MATRIX
-
5.3.4 INTRODUCTION TO SPLITTING THE DATASET USING TRAINTESTSPLIT
-
5.3.5 KNN ALGORITHM
-
5.3.6 KNN ALGORITHM
-
5.4 INTRODUCTION TO DECISION TREE
-
5.4.1 INTRODUCTION TO DECISION TREE
-
5.4.2 DECISION TREE ALGORITHM
-
5.4.3 DECISION TREE ALGORITHM
-
5.4.4 DECISION TREE ALGORITHM
-
5.5 UNSUPERVISED LEARNING
-
5.5.1 UNSUPERVISED LEARNING
-
5.5.2 UNSUPERVISED LEARNING
-
5.5.3 UNSUPERVISED LEARNING
-
5.5.4 AHC ALGORITHM
-
5.5.5 AHC ALGORITHM
-
5.6 KMEANS CLUSTERING
-
5.6.1 KMEANS CLUSTERING
-
5.6.2 KMEANS CLUSTERING
-
5.6.3 DBSCAN ALGORITHM
-
5.6.4 DBSCAN PROGRAM
-
5.6.5 DBSCAN PROGRAM
Course Objectives
• Explain Machine Learning concepts, classifications of Machine Learning and write simple programs using python.
• Describe Supervised Learning concepts.
• Explain Support Vector Machine concepts.
• Describe unsupervised learning concepts and dimensionality reduction techniques.
• Discuss simple Machine Learning applications in a range of real-world applications using Python programming
Machine Learning Basics – Course Syllabus
Introduction :
1. Getting Started with Machine Learning
2. An Introduction to Machine Learning
3. What is Machine Learning ?
4. Introduction to Data in Machine Learning
5. Demystifying Machine Learning
6. ML – Applications
7. Best Python libraries for Machine Learning
8. Artificial Intelligence | An Introduction
9. Machine Learning and Artificial Intelligence
10. Difference between Machine learning and Artificial Intelligence
11. Agents in Artificial Intelligence
12. 10 Basic Machine Learning Interview Questions
Data and It’s Processing:
1. Introduction to Data in Machine Learning
2. Understanding Data Processing
3. Python | Create Test DataSets using Sklearn
4. Python | Generate test datasets for Machine learning
5. Python | Data Preprocessing in Python
6. Data Cleansing
7. Feature Scaling – Part 1
8. Feature Scaling – Part 2
9. Python | Label Encoding of datasets
10. Python | One Hot Encoding of datasets
11. Handling Imbalanced Data with SMOTE and Near Miss Algorithm in Python
Supervised learning :
1. Getting started with Classification
2. Basic Concept of Classification
3. Types of Regression Techniques
4. Classification vs Regression
5. ML | Types of Learning – Supervised Learning
6. Multiclass classification using scikit-learn
7. Gradient Descent :
• Gradient Descent algorithm and its variants
• Stochastic Gradient Descent (SGD)
• Mini-Batch Gradient Descent with Python
• Optimization techniques for Gradient Descent
• Introduction to Momentum-based Gradient Optimizer
8. Linear Regression :
• Introduction to Linear Regression
• Gradient Descent in Linear Regression
• Mathematical explanation for Linear Regression working
• Normal Equation in Linear Regression
• Linear Regression (Python Implementation)
• Simple Linear-Regression using R
• Univariate Linear Regression in Python
• Multiple Linear Regression using Python
• Multiple Linear Regression using R
• Locally weighted Linear Regression
• Python | Linear Regression using sklearn
• Linear Regression Using Tensorflow
• A Practical approach to Simple Linear Regression using R
• Linear Regression using PyTorch
• Pyspark | Linear regression using Apache MLlib
• ML | Boston Housing Kaggle Challenge with Linear Regression
9. Python | Implementation of Polynomial Regression
10. Softmax Regression using TensorFlow
11. Logistic Regression :
• Understanding Logistic Regression
• Why Logistic Regression in Classification ?
• Logistic Regression using Python
• Cost function in Logistic Regression
• Logistic Regression using Tensorflow
12. Naive Bayes Classifiers
13. Support Vector:
• Support Vector Machines(SVMs) in Python
• SVM Hyperparameter Tuning using GridSearchCV
• Support Vector Machines(SVMs) in R
• Using SVM to perform classification on a non-linear dataset
14. Decision Tree:
• Decision Tree
• Decision Tree Regression using sklearn
• Decision Tree Introduction with example
• Decision tree implementation using Python
• Decision Tree in Software Engineering
15. Random Forest:
• Random Forest Regression in Python
• Ensemble Classifier
• Voting Classifier using Sklearn
• Bagging classifier
The course is highly recommended for professionals and undergraduates to shape their careers. The course ensures businesses and individuals have an education and necessary training to succeed in the AI-powered future.The course provides a broad introduction to statistical pattern recognition and machine learning. Differentiates between supervised and unsupervised learning as well as learning theory, reinforcement learning, and control. Explores recent applications of machine learning and design and develops algorithms for machines.
This certification prepares students to expand their career prospects or change career paths by developing foundational data science skills.
Get an industry recognized Certificate by Uplatz upon successful completion of course.
Machine Learning is very popular as it reduces a lot of human efforts and increases machine performance by enabling machines to learn for them. Consequently, there are many career paths in Machine Learning that are popular and well-paying such as Machine Learning Engineer, Data Scientist, NLP Scientist, etc.
Some of the major skills required for this are Programming, Probability, and Statistics, Data Modeling, Machine Learning Algorithms, System Design, etc.
Job Titles:
1. Machine Learning Engineer
2. Data Scientist
3. NLP Scientist
4. Business Intelligence Developer
5. Human-Centered Machine Learning Designer
1. Why was Machine Learning Introduced?
The simplest answer is to make our lives easier. In the early days of “intelligent” applications, many systems used hardcoded rules of “if” and “else” decisions to process data or adjust the user input. Think of a spam filter whose job is to move the appropriate incoming email messages to a spam folder.
But with the machine learning algorithms, we are given ample information for the data to learn and identify the patterns from the data.
Unlike the normal problems we don’t need to write the new rules for each problem in machine learning, we just need to use the same workflow but with a different dataset.
The paper describes the “Imitation Game”, which includes three participants -
- Human acting as a judge,
- Another human, and
- A computer is an attempt to convince the judge that it is human.
The judge asks the other two participants to talk. While they respond the judge needs to decide which response came from the computer. If the judge could not tell the difference the computer won the game.
The test continues today as an annual competition in artificial intelligence. The aim is simple enough: convince the judge that they are chatting to a human instead of a computer chatbot program.
2. What are Different Types of Machine Learning algorithms?
There are various types of machine learning algorithms. Here is the list of them in a broad category based on:
- Whether they are trained with human supervision (Supervised, unsupervised, reinforcement learning)
- The criteria in the below diagram are not exclusive, we can combine them any way we like.
3. What is Supervised Learning?
Supervised learning is a machine learning algorithm of inferring a function from labeled training data. The training data consists of a set of training examples.
Example: 01
Knowing the height and weight identifying the gender of the person. Below are the popular supervised learning algorithms.
- Support Vector Machines
- Regression
- Naive Bayes
- Decision Trees
- K-nearest Neighbour Algorithm and Neural Networks.
Example: 02
If you build a T-shirt classifier, the labels will be “this is an S, this is an M and this is L”, based on showing the classifier examples of S, M, and L.
4. What is Unsupervised Learning?
Unsupervised learning is also a type of machine learning algorithm used to find patterns on the set of data given. In this, we don’t have any dependent variable or label to predict. Unsupervised Learning Algorithms:
- Clustering,
- Anomaly Detection,
- Neural Networks and Latent Variable Models.
Example:
In the same example, a T-shirt clustering will categorize as “collar style and V neck style”, “crew neck style” and “sleeve types”.
5. What is ‘Naive’ in a Naive Bayes?
The Naive Bayes method is a supervised learning algorithm, it is naive since it makes assumptions by applying Bayes’ theorem that all attributes are independent of each other.
Bayes’ theorem states the following relationship, given class variable y and dependent vector x1 through xn:
P(yi | x1,..., xn) =P(yi)P(x1,..., xn | yi)(P(x1,..., xn)
Using the naive conditional independence assumption that each xiis independent: for all I this relationship is simplified to:
P(xi | yi, x1, ..., xi-1, xi+1, ...., xn) = P(xi | yi)
Since, P(x1,..., xn) is a constant given the input, we can use the following classification rule:
P(yi | x1, ..., xn) = P(y) ni=1P(xi | yi)P(x1,...,xn) and we can also use Maximum A Posteriori (MAP) estimation to estimate P(yi)and P(yi | xi) the former is then the relative frequency of class yin the training set.
P(yi | x1,..., xn) P(yi) ni=1P(xi | yi)
y = arg max P(yi)ni=1P(xi | yi)
The different naive Bayes classifiers mainly differ by the assumptions they make regarding the distribution of P(yi | xi): can be Bernoulli, binomial, Gaussian, and so on.
6. What is PCA? When do you use it?
Principal component analysis (PCA) is most commonly used for dimension reduction.
In this case, PCA measures the variation in each variable (or column in the table). If there is little variation, it throws the variable out, as illustrated in the figure below:
Principal component analysis (PCA)
Thus making the dataset easier to visualize. PCA is used in finance, neuroscience, and pharmacology.
It is very useful as a preprocessing step, especially when there are linear correlations between features.
7. Explain SVM Algorithm in Detail
A Support Vector Machine (SVM) is a very powerful and versatile supervised machine learning model, capable of performing linear or non-linear classification, regression, and even outlier detection.
Suppose we have given some data points that each belong to one of two classes, and the goal is to separate two classes based on a set of examples.
In SVM, a data point is viewed as a p-dimensional vector (a list of p numbers), and we wanted to know whether we can separate such points with a (p-1)-dimensional hyperplane. This is called a linear classifier.
There are many hyperplanes that classify the data. To choose the best hyperplane that represents the largest separation or margin between the two classes.
If such a hyperplane exists, it is known as a maximum-margin hyperplane and the linear classifier it defines is known as a maximum margin classifier. The best hyperplane that divides the data in H3
We have data (x1, y1), ..., (xn, yn), and different features (xii, ..., xip), and yiis either 1 or -1.
The equation of the hyperplane H3 is the set of points satisfying:
w. x-b = 0
Where w is the normal vector of the hyperplane. The parameter b||w||determines the offset of the hyperplane from the original along the normal vector w
So for each i, either xiis in the hyperplane of 1 or -1. Basically, xisatisfies:
w . xi - b = 1 or w. xi - b = -1
8. What are Support Vectors in SVM?
A Support Vector Machine (SVM) is an algorithm that tries to fit a line (or plane or hyperplane) between the different classes that maximizes the distance from the line to the points of the classes.
In this way, it tries to find a robust separation between the classes. The Support Vectors are the points of the edge of the dividing hyperplane as in the below figure.
9. What are Different Kernels in SVM?
There are six types of kernels in SVM:
- Linear kernel - used when data is linearly separable.
- Polynomial kernel - When you have discrete data that has no natural notion of smoothness.
- Radial basis kernel - Create a decision boundary able to do a much better job of separating two classes than the linear kernel.
- Sigmoid kernel - used as an activation function for neural networks.
10. What is Cross-Validation?
Cross-validation is a method of splitting all your data into three parts: training, testing, and validation data. Data is split into k subsets, and the model has trained on k-1of those datasets.
The last subset is held for testing. This is done for each of the subsets. This is k-fold cross-validation. Finally, the scores from all the k-folds are averaged to produce the final score.