Overview Course - Deep Learning
You will learn about a wide range of other courses flesh out the possibilities of deep learning in image classification and recognition.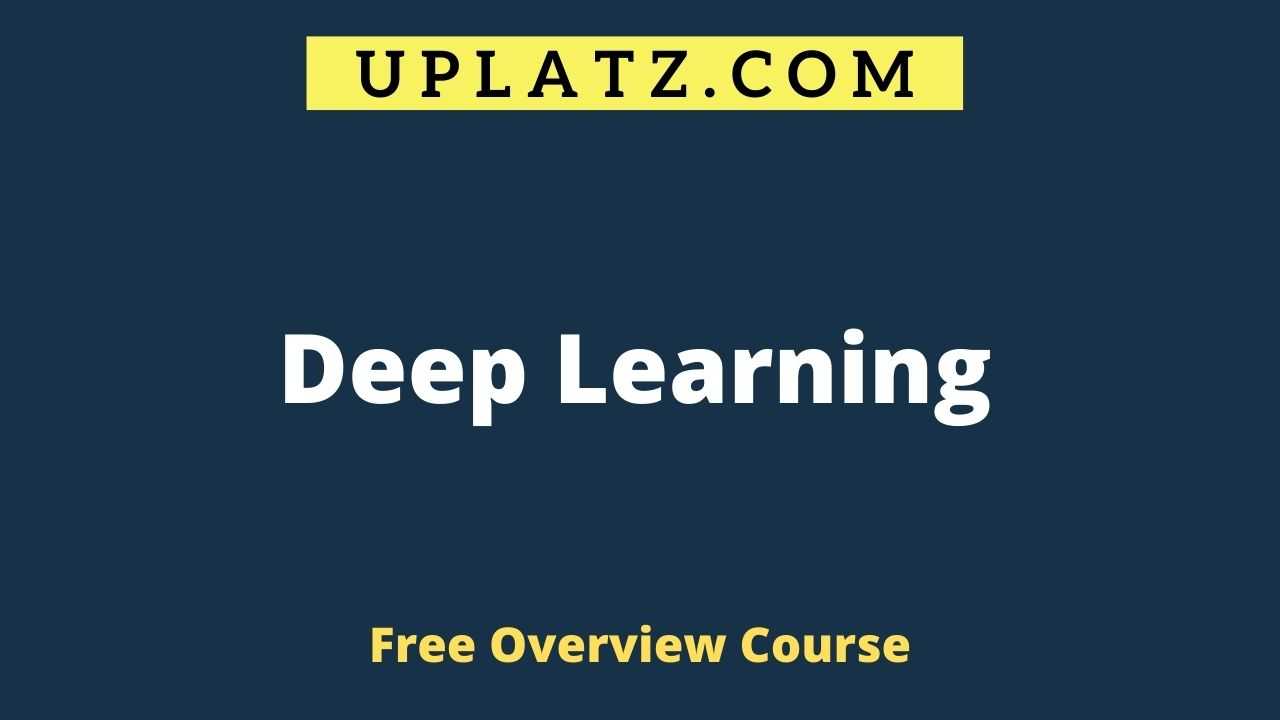
96% Started a new career BUY THIS COURSE (
USD 2 USD 14 )-
98% Got a pay increase and promotion
Students also bought -
-
- Deep Learning Foundation
- 10 Hours
- USD 12
- 1061 Learners
-
- Deep Learning with Keras
- 15 Hours
- USD 12
- 732 Learners
-
- Deep Learning with TensorFlow
- 50 Hours
- USD 12
- 333 Learners
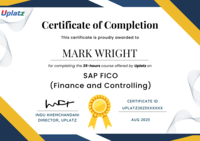
This is an overview course on Deep Learning while the complete detailed-level Deep Learning course is available at -
1. Deep Learning Foundation - https://training.uplatz.com/online-it-course.php?id=deep-learning-foundation-deep-learning-foundation-304
2. Deep Learning with Keras - https://training.uplatz.com/online-it-course.php?id=deep-learning-with-keras-deep-learning-with-keras-312
3. Deep Learning with TensorFlow - https://training.uplatz.com/online-it-course.php?id=deep-learning-with-tensorflow-deep-learning-with-tensorflow-132
Deep Learning is one of the most highly sought-after skills in AI. Uplatz will help you to become good at Deep Learning. In Uplatz training, you will learn the foundations of Deep Learning, understand how to build neural networks, and learn how to lead successful machine learning projects. You will learn about the difficult networks like RNNs, LSTM, Adam, Dropout, Batch Norm, Xavier/He initialization, and more. You will work on case studies from healthcare, autonomous driving, sign language reading, music generation, and natural language processing. You will master not only the theory, but also see how it is applied in industry.
Deep Learning is inspired by the ways humans process information and then communicate through our own biological neural networks. These Deep learning methods are able to process vast amounts of data, taking those datasets and building essential meaning. It's an expansion of machine learning, allowing the user to develop broader, more in-depth solutions as opposed to highly task-specific ones because of its artificial neural networks.
Uplatz offers quite a collection of courses in partnership with some of the foremost universities in the field. After Deep Learning Foundation, a wide range of other courses flesh out the possibilities of deep learning in image classification and recognition, building hidden layers for unsupervised learning, and using computing power for state-of-the-art speech recognition. Uplatz courses offer you a rare chance to get inside the black box of deep learning and build your own solutions.
Course/Topic - Deep Learning overview - all lectures
-
In this session we will learn about the introduction to Deep Learning. This video talks about Deep Learning as a series introduction and what is a neural network. Furthermore, we will talk about the 3 reasons to go deep and your choice of Deep net.
-
In this video tutorial we will discuss about the neural networks and the 3 reasons to go Deep. Further we will also learn about the use of GPU in artificial intelligence and your choice of deep net.
-
In this first session we will be learning about introductory topics of Deep Learning. We will see about what is Deep learning and what is artificial neural network. Furthermore, in the introduction to deep learning with Keras we will see the overview, features and benefits of Keras. Lastly we will learn about the Keras installation.
-
Class 1 - TensorFlow Introduction
Certification - The Deep Learning Certification ensures you know planning, production and measurement techniques needed to stand out from the competition.
Deep learning is a type of machine learning and artificial intelligence (AI) that imitates the way humans gain certain types of knowledge. Deep learning is an important element of data science, which includes statistics and predictive modeling.
Deep learning is a machine learning technique that teaches computers to do what comes naturally to humans: learn by example. Deep learning is a key technology behind driverless cars, enabling them to recognize a stop sign, or to distinguish a pedestrian from a lamppost.
Deep Learning is called Deep because of the number of additional “Layers” we add to learn from the data. If you do not know it already, when a deep learning model is learning, it is simply updating the weights through an optimization function. A Layer is an intermediate row of so-called “Neurons”.
Deep learning, when applied to data science, can offer better and more effective processing models. Its ability to learn unsupervised drives continuous improvement in accuracy and outcomes. It also offers data scientists with more reliable and concise analysis results.
Uplatz online training guarantees the participants to successfully go through the Deep Learning Certification provided by Uplatz. Uplatz provides appropriate teaching and expertise training to equip the participants for implementing the learnt concepts in an organization.
Course Completion Certificate will be awarded by Uplatz upon successful completion of the Deep Learning online course.
Career and Jobs - The Deep Learning draws an average salary of $120,000 per year depending on their knowledge and hands-on experience.
Machine learning is a good career path. According to a 2019 report by Indeed, Machine Learning Engineer is the top job in terms of salary, growth of postings, and general demand.
Deep learning known as neural organized learning or different leveled learning is part of a more extensive group with a wide range of jobs (like software engineer, research analyst, Data Analyst, Data Engineer, Bioinformation, Software developer, etc.)
Note that salaries are generally higher at large companies rather than small ones. Your salary will also differ based on the market you work in.
Deep Learning Engineer.
AI - Deep Learning/Computer Vision.
Deep Learning Engineers.
Machine Learning Trainer.
Deep Learning Foundation - Syllabus
Module 1 - Introduction to Deep Learning
· Deep Learning: The Series Introduction
· What is a Neural Network
· Three reasons to go Deep
· Your choice of Deep Net
· Deep Learning Market
Module 2 - Deep Learning Models
· Restricted Boltzmann Machines
· Deep Belief Network
· Convolutional Network
· Recurrent Network
Module 3 - Additional Deep Learning Models
· Autoencoders
· Recursive Neural Tensor Network
· Generative Adversarial Networks
Module 4 - Deep Learning Platforms & Libraries
· What is a Deep Net Platform
· H2O.ai
· Dato GraphLab
· What is a Deep Learning Library
· Theano
· Caffe
· TensorFlow
· Keras
The Deep LearningCertification ensures you know planning, production and measurement techniques needed to stand out from the competition.
Deep learning is a type of machine learning and artificial intelligence (AI) that imitates the way humans gain certain types of knowledge. Deep learning is an important element of data science, which includes statistics and predictive modeling.
Deep learning is a machine learning technique that teaches computers to do what comes naturally to humans: learn by example. Deep learning is a key technology behind driverless cars, enabling them to recognize a stop sign, or to distinguish a pedestrian from a lamppost.
Deep Learning is called Deep because of the number of additional “Layers” we add to learn from the data. If you do not know it already, when a deep learning model is learning, it is simply updating the weights through an optimization function. A Layer is an intermediate row of so-called “Neurons”.
Deep learning, when applied to data science, can offer better and more effective processing models. Its ability to learn unsupervised drives continuous improvement in accuracy and outcomes. It also offers data scientists with more reliable and concise analysis results.
Uplatz online training guarantees the participants to successfully go through the Deep Learning Certification provided by Uplatz. Uplatz provides appropriate teaching and expertise training to equip the participants for implementing the learnt concepts in an organization.
Course Completion Certificate will be awarded by Uplatz upon successful completion of the Deep Learning online course.
The Deep Learning draws an average salary of $120,000 per year depending on their knowledge and hands-on experience.
Machine learning is a good career path. According to a 2019 report by Indeed, Machine Learning Engineer is the top job in terms of salary, growth of postings, and general demand.
Deep learning known as neural organized learning or different leveled learning is part of a more extensive group with a wide range of jobs (like software engineer, research analyst, Data Analyst, Data Engineer, Bioinformation, Software developer, etc.)
Note that salaries are generally higher at large companies rather than small ones. Your salary will also differ based on the market you work in.
Deep Learning Engineer.
AI - Deep Learning/Computer Vision.
Deep Learning Engineers.
Machine Learning Trainer.
1. What is Deep Learning?
If you are going for a deep learning interview, you definitely know what exactly deep learning is. However, with this question the interviewee expects you to give an in-detail answer, with an example. Deep Learning involves taking large volumes of structured or unstructured data and using complex algorithms to train neural networks. It performs complex operations to extract hidden patterns and features (for instance, distinguishing the image of a cat from that of a dog).
2. What is a Neural Network?
Neural Networks replicate the way humans learn, inspired by how the neurons in our brains fire, only much simpler.
The most common Neural Networks consist of three network layers:
1. An input layer
2. A hidden layer (this is the most important layer where feature extraction takes place, and adjustments are made to train faster and function better)
3. An output layer
Each sheet contains neurons called “nodes,” performing various operations. Neural Networks are used in deep learning algorithms like CNN, RNN, GAN, etc.
3. What Is a Multi-layer Perceptron(MLP)?
As in Neural Networks, MLPs have an input layer, a hidden layer, and an output layer. It has the same structure as a single layer perceptron with one or more hidden layers. A single layer perceptron can classify only linear separable classes with binary output (0,1), but MLP can classify nonlinear classes.
Except for the input layer, each node in the other layers uses a nonlinear activation function. This means the input layers, the data coming in, and the activation function is based upon all nodes and weights being added together, producing the output. MLP uses a supervised learning method called “backpropagation.” In backpropagation, the neural network calculates the error with the help of cost function. It propagates this error backward from where it came (adjusts the weights to train the model more accurately).
4. What Is Data Normalization, and Why Do We Need It?
The process of standardizing and reforming data is called “Data Normalization.” It’s a pre-processing step to eliminate data redundancy. Often, data comes in, and you get the same information in different formats. In these cases, you should rescale values to fit into a particular range, achieving better convergence.
5. What is the Boltzmann Machine?
One of the most basic Deep Learning models is a Boltzmann Machine, resembling a simplified version of the Multi-Layer Perceptron. This model features a visible input layer and a hidden layer -- just a two-layer neural net that makes stochastic decisions as to whether a neuron should be on or off. Nodes are connected across layers, but no two nodes of the same layer are connected.
6. What Is the Role of Activation Functions in a Neural Network?
At the most basic level, an activation function decides whether a neuron should be fired or not. It accepts the weighted sum of the inputs and bias as input to any activation function. Step function, Sigmoid, ReLU, Tanh, and Softmax are examples of activation functions.
7. What Is the Cost Function?
Also referred to as “loss” or “error,” cost function is a measure to evaluate how good your model’s performance is. It’s used to compute the error of the output layer during backpropagation. We push that error backward through the neural network and use that during the different training functions.
8. What Is Gradient Descent?
Gradient Descent is an optimal algorithm to minimize the cost function or to minimize an error. The aim is to find the local-global minima of a function. This determines the direction the model should take to reduce the error.
9. What Do You Understand by Backpropagation?
This is one of the most frequently asked deep learning interview questions. Backpropagation is a technique to improve the performance of the network. It backpropagates the error and updates the weights to reduce the error.
10. What Is the Difference Between a Feedforward Neural Network and Recurrent Neural Network?
In this deep learning interview question, the interviewee expects you to give a detailed answer.
A Feedforward Neural Network signals travel in one direction from input to output. There are no feedback loops; the network considers only the current input. It cannot memorize previous inputs
A Recurrent Neural Network’s signals travel in both directions, creating a looped network. It considers the current input with the previously received inputs for generating the output of a layer and can memorize past data due to its internal memory.
11. What Are the Applications of a Recurrent Neural Network (RNN)?
The RNN can be used for sentiment analysis, text mining, and image captioning. Recurrent Neural Networks can also address time series problems such as predicting the prices of stocks in a month or quarter.
12. What Are the Softmax and ReLU Functions?
Softmax is an activation function that generates the output between zero and one. It divides each output, such that the total sum of the outputs is equal to one. Softmax is often used for output layers.
ReLU (or Rectified Linear Unit) is the most widely used activation function. It gives an output of X if X is positive and zeros otherwise. ReLU is often used for hidden layers.
13. What Are Hyperparameters?
This is another frequently asked deep learning interview question. With neural networks, you’re usually working with hyperparameters once the data is formatted correctly. A hyperparameter is a parameter whose value is set before the learning process begins. It determines how a network is trained and the structure of the network (such as the number of hidden units, the learning rate, epochs, etc.).
14. What Will Happen If the Learning Rate Is Set Too Low or Too High?
When your learning rate is too low, training of the model will progress very slowly as we are making minimal updates to the weights. It will take many updates before reaching the minimum point.
If the learning rate is set too high, this causes undesirable divergent behavior to the loss function due to drastic updates in weights. It may fail to converge (model can give a good output) or even diverge (data is too chaotic for the network to train).
15. What Is Dropout and Batch Normalization?
Dropout is a technique of dropping out hidden and visible units of a network randomly to prevent overfitting of data (typically dropping 20 percent of the nodes). It doubles the number of iterations needed to converge the network.
Batch normalization is the technique to improve the performance and stability of neural networks by normalizing the inputs in every layer so that they have mean output activation of zero and standard deviation of one.