Bundle Combo - Data Science (with Python and R)
Become a top Data Scientist by using top programming languages - Python and R - in Data Science field. Learn how to do data science with R & Python.Preview Bundle Combo - Data Science (with Python and R) course
Price Match Guarantee Full Lifetime Access Access on any Device Technical Support Secure Checkout   Course Completion Certificate100% Started a new career BUY THIS COURSE (
USD 23 USD 69 )-
98% Got a pay increase and promotion
Students also bought -
-
- Data Science with Python
- 45 Hours
- USD 14
- 2931 Learners
-
- Data Science with R
- 25 Hours
- USD 14
- 479 Learners
-
- Career Path - Data Scientist
- 300 Hours
- USD 45
- 6978 Learners
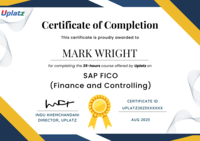
Courses included in Bundle Combo - Data Science with Python and R provided by Uplatz are the following:
1) Data Science with Python
Data science takes into account the whole process starting from understanding the business requirements to preparing the data for model building and deploying the insights finally. The whole process is handled by different professionals including Data Analysts, Data Engineers, and Data Scientists. The role depends upon the size of the company, sometimes all the processes are done by just one professional. Let us try to understand why python is the right programming language for Data Science. Python for Data Science is a must-learn skill for professionals in the Data Analytics domain. With the growth in the IT industry, there is a booming demand for skilled Data Scientists and Python has evolved as the most preferred programming language for data-driven development. Through this course, you will learn the basics, how to analyze data, and then create some beautiful visualizations using Python.
2) Data Science with R
R is a powerful language used widely for data analysis and statistical computing. It was developed in the early 90s. Since then, endless efforts have been made to improve R’s user interface. The journey of R language from a rudimentary text editor to interactive R Studio and has engaged many data science communities across the world. This was possible only because of generous contributions by R users globally. Inclusion of powerful packages in R has made it more and more powerful with time. Packages such as dplyr, tidyr, readr, data.table, SparkR, ggplot2 have made data manipulation, visualization and computation much faster. In these data science courses, you’ll learn how to use the R language to access databases, clean, analyze, and visualize data with R. This is an action-packed learning path for data science enthusiasts who want to work with real world problems using R.
This Bundle Combo course on Data Science with Python & R will help you to apply data science to real-world problems by using Python or R programming languages.
Unlike some other programming languages, in Python, there is generally a best way of doing something. The three best and most important Python libraries for data science are NumPy, Pandas, and Matplotlib. The important Python libraries for data science include:
a).NumPy - A library that makes a variety of mathematical and statistical operations easier; it is also the basis for many features of the pandas library.
b).Pandas - A Python library created specifically to facilitate working with data, this is the bread and butter of a lot of Python data science work.
c).Matplotlib - A visualization library that makes it quick and easy to generate charts from your data.
d).scikit-learn - The most popular library for machine learning work in Python.
NumPy and Pandas are great for exploring and playing with data. Matplotlib is a data visualization library that makes graphs like you’d find in Excel or Google Sheets.
Gain the career-building R skills you need to succeed as a data scientist. In this course, you’ll learn how R, a versatile language, allows you to import, clean, manipulate, and visualize data—all integral skills for any aspiring data professional or researcher. You'll learn some of the most popular R packages, including ggplot2 and tidyverse packages like dplyr and readr. You’ll also work with real-world datasets to learn the statistical and machine learning techniques you need to write your own functions and perform cluster analysis. With this course, you will not only grow your R skills, but will begin your journey to becoming a confident data scientist.
Course/Topic 1 - Data Science with Python - all lectures
-
In this video tutorial we will get introduced to Data Science and the integration of Python in Data Science. Furthermore, we will look into the importance of Data Science and its demand and the application of Data Science.
-
In this video we will learn, all the concepts of Python programming related to Data Science. We will also learn about the Introduction to Python Programing, what is Python Programming and its History, Features and Application of Python along with its setup. Further we will see how to get started with the first python program.
-
This video talks about the Variable and Data Types in Python Programming. In this session we will learn What is variable, the declaration of variable and variable assignment. Further we will see the data types in python, checking data types and data type conversions.
-
This tutorial will help you to understand Data Types in python in depth. This video talks about the data types such as numbers, sequence type, Boolean, set and dictionary.
-
This tutorial talks about the Identifier, keyword, reading input and output formatting in Data Science. We will learn about what is an identifier and keywords. Further we will learn about reading input and taking multiple inputs from a user, Output formatting and Python end parameter.
-
This tutorial talks about taking multiple inputs from user and output formatting using format method, string method and module operator.
-
This tutorial talks about the Operators and type of operators. In this session we will learn about the types of operators such as arithmetic, Relational and Assignment Operators.
-
This tutorial talks further about the part 2 of operators and its types. In this session we will learn about the types of operators such as Logical, Membership, Identity and Bitwise Operators.
-
In this video you will learn about the process of decision making in Data Science. Furthermore, this tutorial talks about different types of decision-making statements and its application in Data Science.
-
In this video tutorial we will learn about the Loops in Python programing. We will cover further the different types of Loops in Python, starting with: For Loop.
-
In this session we will cover the further part of loops in Python programming. The type of loops explained in this video is: While loop and nested loop.
-
In this session we will cover the further part of loops in Python programming. The type of loops explained in this video is: break, continue and pass loops
-
In this video tutorial we will start explaining about the lists in Python Programming. This tutorial talks about accessing values in the list and updating the list in Data Science.
-
In this video tutorial we will look into the further parts about the lists in Python Programming. Deleting list elements, basic list operations, built in functions and methods and the features which are covered in this session.
-
This tutorial will cover the basics on Tuples and Dictionary function in Data Science. We will learn about accessing and deleting tuple elements. Further we will also cover the basic tuples operations and the built in tuple functions and its methods. At the end we will see the differences in list and tuple.
-
This tutorial will cover the advanced topics on Tuples and Dictionary function in Data Science. Further in this session we will learn about the Python Dictionary, how to access, update and delete dictionary elements. Lastly we will cover built in functions and methods.
-
In this session we will learn about the functions and modules used in Data science. After watching this video, you will be able to understand what is a function, the definition of function and calling a function.
-
In this session we will learn about the further functions and modules used in Data science. After watching this video, you will be able to understand the ways to write a function, Types of functions, Anonymous Functions and Recursive functions.
-
In this session we will learn about the advanced functions and modules used in Data science. After watching this video, you will be able to understand what is a module, creating a module, import statement and locating modules.
-
This tutorial talks about the features of working with files. In this video we will learn about opening and closing file, the open function, the file object Attributes, the close method, reading and writing files.
-
This tutorial talks about the advanced features of working with files. In this video we will learn about file positions, renaming and deleting files.
-
In this session we will learn about the regular expression. After this video you will be able to understand what is a regular expression, meta characters, match function, search function, Re- match vs research, split function and sub function.
-
This video introduces you to the Data Science Libraries. In this video you will learn about the Data science libraries: libraries for data processing, modelling and data visualization.
-
In this session we will teach about the components of python ecosystem in Data Science. This video talks about the Components of Python Ecosystem using package Python distribution Anaconda and jupyter notebook.
-
This tutorial talks about the basics of analyzing data using numpy and pandas. The Pandas module mainly works with the tabular data, whereas the NumPy module works with the numerical data. We will further see what is Numpy and why we use numpy.
-
This tutorial talks about the later part of analyzing data using numpy and pandas. In this tutorial we will learn how to install numpy.
-
This tutorial talks about the advanced part of analyzing data using numpy and pandas. In this session we will learn what is Pandas and the key features of Pandas. We will also learn about the Python Pandas environment setup.
-
This tutorial talks about the advanced part of analyzing data using numpy and pandas. In this session we will learn about Pandas data structure with example.
-
This the last session on Analysing Data using Numpy and Pandas. In this session we will learn data analysis using Pandas
-
In this video tutorial we will learn about the Data Visualization using Matpotlib. This video talks about what is data visualisation, introduction to matplotlib and installation of matplotlib.
-
In this session we will see the part 2 of Data Visualization with Matplotlib. This video talks about the types of data visualization charts and line chart scatter plot
-
This tutorial covers part 3 of Data Visualization with Matplotlib. This session covers the types of data visualisation charts: bar chart histogram, area plot pie chart and box plot contour plot.
-
This session talks about the Three-Dimensional Plotting with Matplotlib . In this we will learn about plot 3D scatter, plot 3D contour and plot 3D surface plot.
-
In this tutorial we will cover basics of Data Visualisation with Seaborn. Further we will cover Introduction to seaborn, seaborn functionalities, how to install seaborn and the different categories of plot in seaborn
-
In this tutorial we will cover the advanced topics of Data Visualisation with Seaborn. In this video we will see about exploring seaborn plots.
-
Introduction to Statistical Analysis is taught in this video. We will learn what is statistical analysis and introduction to math and statistics for data science. Further we will learn about the terminologies in statistics for data science and categories in statistics, its correlation and lastly mean median and mode quartile.
-
This video course talks about the basics of Data Science methodology. We will learn how to reach from problem to approach.
-
In this session we will see Data Science Methodology from requirements to collection and from understanding to preparation.
-
In this session we will learn advanced Data Science Methodology from modelling to evaluation and from deployment to feedback.
-
This video tutorial talks about the - Introduction to Machine Learning and its Types. In this session we will learn what is machine learning and the need for machine learning. Further we will see the application of machine learning and different types of machine learning. We will also cover topics such as supervised learning, unsupervised learning and reinforcement learning.
-
This video tutorial talks about the basics of regression analysis. We will cover in this video linear regression and implementing linear regression.
-
This video tutorial talks about the further topics of regression analysis. In this video we will learn about multiple linear regression and implementing multiple linear regression.
-
This video tutorial talks about the advanced topics of regression analysis. In this video we will learn about polynomial regression and implementing polynomial regression.
-
In this session we will learn about the classification in Data science. We will see what is classification, classification algorithms and Logistic regression. Also we will learn about implementing Logistic regression.
-
In this session we will learn about the further topics of classification in Data science, such as decision tree and implementing decision tree.
-
In this session we will learn about the advanced topics of classification in Data science, such as support vendor machine and implementing support vector machine.
-
This tutorial will teach you about what is clustering and clustering algorithms. Further we will learn what K means clustering and how does K means clustering work and also about implementing K means clustering.
-
In this session we will see the further topics of clustering, such as hierarchical clustering, agglomerative hierarchical clustering, how does agglomerative hierarchical clustering Work and divisive hierarchical clustering.
-
This video tutorial talks about the advanced topics of clustering, such as implementation of agglomerative hierarchical clustering.
-
This video will help you to understand basics of Association rule learning. In this session we will learn about the Apriori algorithm and the working of Apriori algorithm.
-
This video will help you to understand advanced topics of Association rule learning such as implementation of Apriori algorithm.
-
This is a session on the practical part of Data Science application. In this example we will see problem statement, data set, exploratory data analysis.
-
This is a session on the practical part of Data Science application.
-
This is a session on the practical part of Data Science application. In this we will see the implementation of the project.
-
This is a session on the practical part of Data Science application
-
This is a session on the practical part of Data Science application
Course/Topic 2 - Data Science with R - all lectures
-
In this lecture session we learn about introduction of data science and also talk about features of data science in R.
-
In this lecture session we learn about data collection and management and also talk about features of data collection and management in data science with R.
-
In this lecture session we learn about model deployment and maintenance and also talk about functions of model deployment and maintenance in data science with R.
-
In this lecture session we learn about setting expectations and also talk about factors of setting expectations in brief.
-
In this lecture session we learn about loading data into R and also talk about features of loading data into R and also talk about the importance of loading data into R.
-
In this lecture session we learn about exploring data in data science and machine learning and also talk about features of exploring data in data science and machine learning.
-
In this lecture session we learn about features of exploring data using R and also talk about factors of exploring data using R.
-
In this lecture session we learn about benefits of data cleaning and also talk about features of benefits of data cleaning.
-
In this lecture session we learn about cross validation in R and also talk about features of validation in data science with R.
-
In these lecture sessions we learn about data transformation in data science with R and also talk about features of data transformation in brief.
-
In this lecture session we learn about modeling methods in data science with R and also talk about the importance of modeling methods.
-
In this lecture session we learn about solving classification problems and also talk about features of solving classification problems in brief.
-
In this lecture session we learn about working without known targets in data science with r and also talk about features of working without known targets.
-
In this lecture session we learn about evaluating models in data science with R and also talk about features of evaluating models in brief.
-
In this lecture session we learn about confusion matrix in indian accounting standards and also talk about features of confusion matrix.
-
In this lecture session we learn about introduction to linear regression and also talk about features of linear regression in indian accounting standards.
-
In this lecture session we learn about linear regression in R and also talk about features and functions of linear regression in brief.
-
In this lecture session we learn about linear regression in R in data science with r and also talk about features of linear regression in R language.
-
In this lecture session we learn about simple and multiple regression in data science with r and also talk about the basic difference between simple and multiple regression in brief.
-
In this lecture session we learn about linear and logistic regression in data science with r language and also talk about functions of linear and logistics regressions.
-
In this lecture session we learn about support vector machines (SVM) in R and also talk about features of support vector machines in data science with R language.
-
In this lecture session we learn about factors of support vectors machines in data science with R and also talk about features of support vectors machines.
-
In this lecture session we learn about unsupervised methods in data science with R and also talk about functions of unsupervised methods in data science.
-
In this lecture session we learn about clustering in data science with R language and also talk about features of clustering in data science.
-
In this lecture session we learn about K-means algorithms in R and also talk about all types of algorithms in data science with R language.
-
In this lecture session we learn about hierarchical clustering in data science with R language and also talk about features of hierarchical clustering.
-
In this lecture session we learn about libraries in data science with R and also talk about libraries of hierarchical clustering in brief.
-
In this lecture session we learn about the dendrogram of diana and also talk about all types of clustering in data science with R.
-
In this lecture session we learn about market basket analysis in data science with R and also talk about features of market basket analysis in data science with R.
-
In this lecture session we learn about MBA and association rule mining in data science with r language.
-
In this lecture session we learn about implementing MBA in data science with R and also talk about implementing MBA.
-
In this lecture session we learn about association rule learning in data science with R and also talk about features of association rule learning.
-
In this lecture session we learn about decision tree algorithms in data science with R and also talk about features of tree algorithms.
-
In this lecture session we learn about exploring advanced methods in tree algorithms in data science with R and also talk about features of exploring advanced methods.
-
In this lecture session we learn about using kernel methods and also talk about features of using kernel methods in data science with R.
-
In this lecture session we learn about documentation and deployment and also talk about features of documentation and deployment in data science with R.
a).How to use modules and create your own modules,
b).Data science and Data literacy concepts,
c).Fundamentals of Numpy for Data manipulation such as Numpy arrays and their features
d).How to do indexing and slicing on Arrays
e).The logic of Loops and control statements
f).Hierarchical indexing concept and theory
This course provides an in-depth understanding of data science concepts using Python and R, two of the most popular programming languages in the field. Participants will learn data manipulation, statistical analysis, machine learning, and data visualization techniques.
Week 1: Introduction to Data Science
a).What is Data Science?
b).Overview of the data science lifecycle
c).Key roles in data science
d).Introduction to Python and R for data science
Week 2: Data Exploration and Manipulation
a).Data types and structures in Python (NumPy, pandas) and R (data.frames, tibbles)
b).Data import and export: CSV, Excel, databases
c).Data cleaning and preprocessing techniques
d).Hands-on exercises: Cleaning a dataset
Week 3: Data Visualization
a).Importance of data visualization
b).Visualization libraries: Matplotlib and Seaborn (Python), ggplot2 (R)
c).Creating effective visualizations: Bar charts, histograms, scatter plots
d).Project: Visualizing data insights from a provided dataset
Week 4: Statistics for Data Science
a).Descriptive statistics: Measures of central tendency and variability
b).Probability distributions: Normal, binomial, Poisson
c).Inferential statistics: Hypothesis testing, confidence intervals
d).Hands-on exercises in Python and R
Week 5: Introduction to Machine Learning
a).Overview of machine learning concepts
b).Supervised vs. unsupervised learning
c).Key algorithms: Linear regression, decision trees
d).Hands-on: Building a simple predictive model in Python and R
Week 6: Advanced Machine Learning Techniques
a).Model evaluation metrics: Accuracy, precision, recall, F1-score
b).Cross-validation and hyperparameter tuning
c).Ensemble methods: Random forests, boosting
d).Implementing models using Scikit-learn (Python) and caret (R)
Week 7: Text Mining and Natural Language Processing
a).Introduction to text data
b).Text preprocessing techniques: Tokenization, stemming, and lemmatization
c).Basic NLP techniques: Sentiment analysis and topic modeling
d).Project: Analyzing text data (e.g., tweets, reviews)
Week 8: Big Data and Data Wrangling
a).Overview of big data technologies
b).Introduction to databases: SQL and NoSQL
c).Data wrangling with dplyr (R) and pandas (Python)
d).Hands-on exercises with large datasets
Week 9: Real-World Applications and Case Studies
a).Case studies across industries: Healthcare, finance, retail
b).Ethics in data science: Bias, fairness, and privacy
c).Effective communication of data findings
d).Group discussion on case studies
Week 10: Building a Data Science Portfolio
a).Importance of a portfolio in data science
b).Projects to include: Data analysis, machine learning models, visualizations
c).Best practices for presenting your work
d).Tips for job hunting and interview preparation
Recommended Resources:
1).Textbooks:
a)."Python for Data Analysis" by Wes McKinney
b)."R for Data Science" by Hadley Wickham and Garrett Grolemund
2).Online Courses:
a).Coursera, edX, or DataCamp for additional learning materials
3).Tools:
a).Jupyter Notebook, RStudio, Git for version control
4).Assessment:
1).Weekly assignments and quizzes
2).Mid-term project demonstrating data analysis skills
3).Final capstone project showcasing a comprehensive data science project
This Data Science (with Python and with R) Based Training course is designed for exploring the structure, concepts of Data Science (with Python and with R) modules.
“Data science” is just about as broad of a term as they come. It may be easiest to describe what it is by listing its more concrete components: Data exploration & analysis. Included here: Pandas; NumPy; SciPy; a helping hand from Python's Standard Library.
Learn how to use R to turn raw data into insight, knowledge, and understanding. This book introduces you to R, RStudio, and the tidyverse, a collection of R packages designed to work together to make data science.
Uplatz online training guarantees the participants to successfully go through the Data science certification provided by Uplatz. Uplatz provides appropriate teaching and expertise training to equip the participants for implementing the learnt concepts in an organization.
Course Completion Certificate will be awarded by Uplatz upon successful completion of the Data science online course.
The draws an average salary of $150,080 per year depending on the knowledge and hands-on experience. The Data scientist job roles are in high demand and make a rewarding career.
Developing and maintaining databases, data systems – reorganizing data in a readable format. Performing analysis to assess quality and meaning of data. Filter Data by reviewing reports and performance indicators to identify and correct code problems
Note that salaries are generally higher at large companies rather than small ones. Your salary will also differ based on the market you work in.
Q1).Explain about data import in R language
Ans-R Commander is used to import data in R language. To start the R commander GUI, the user must type in the command Rcmdr into the console. There are 3 different ways in which data can be imported in R language-
a).Users can select the data set in the dialog box or enter the name of the data set (if they know).
b).Data can also be entered directly using the editor of R Commander via Data->New Data Set. However, this works well when the data set is not too large.
c).Data can also be imported from a URL or from a plain text file (ASCII), from any other statistical package or from the clipboard.
Q2).Two vectors X and Y are defined as follows – X <- c(3, 2, 4) and Y <- c(1, 2). What will be output of vector Z that is defined as Z <- X*Y.
Ans-In R language when the vectors have different lengths, the multiplication begins with the smaller vector and continues till all the elements in the larger vector have been multiplied.
The output of the above code will be –
Z <- (3, 4, 4)
Q3).How missing values and impossible values are represented in R language?
Ans-NaN (Not a Number) is used to represent impossible values whereas NA (Not Available) is used to represent missing values. The best way to answer this question would be to mention that deleting missing values is not a good idea because the probable cause for missing value could be some problem with data collection or programming or the query. It is good to find the root cause of the missing values and then take necessary steps handle them.
Q4).R language has several packages for solving a particular problem. How do you make a decision on which one is the best to use?
Ans-CRAN package ecosystem has more than 6000 packages. The best way for beginners to answer this question is to mention that they would look for a package that follows good software development principles. The next thing would be to look for user reviews and find out if other data scientists or analysts have been able to solve a similar problem.
Q5).Which function in R language is used to find out whether the means of 2 groups are equal to each other or not?
t.tests ()
Q6).What is the best way to communicate the results of data analysis using R language?
Ans-The best possible way to do this is combine the data, code and analysis results in a single document using knitr for reproducible research. This helps others to verify the findings, add to them and engage in discussions. Reproducible research makes it easy to redo the experiments by inserting new data and applying it to a different problem.
Q7).How many data structures does R Language have?
Ans-R language has Homogeneous and Heterogeneous data structures. Homogeneous data structures have same type of objects – Vector, Matrix ad Array. Heterogeneous data structures have different type of objects – Data frames and lists.
Q8).What is the value of f (2) for the following R code?
Ans-
b <- 4
f <- function (a)
{
b <- 3
b^3 + g (a)
}
g <- function (a)
{
a*b
}
The answer to the above code snippet is 35. The value of “a” passed to the function is 2 and the value for “b” defined in the function f (a) is 3. So the output would be 3^3 + g (2). The function g is defined in the global environment and it takes the value of b as 4(due to lexical scoping in R) not 3 returning a value 2*4= 8 to the function f. The result will be 3^3+8= 35.
Q9).What is the process to create a table in R language without using external files?
Ans-MyTable= data.frame ()
edit (MyTable)
The above code will open an Excel Spreadsheet for entering data into MyTable.
Q10).Explain about the significance of transpose in R language
Ans-Transpose t () is the easiest method for reshaping the data before analysis.
Q11).What are with () and BY () functions used for?
Ans-With () function is used to apply an expression for a given dataset and BY () function is used for applying a function each level of factors.
Q12).In base graphics system, which function is used to add elements to a plot?
boxplot () or text ()
Q13).What are the different type of sorting algorithms available in R language?
Ans-
a).Bucket Sort
b).Selection Sort
c).Quick Sort
d).Bubble Sort
e).Merge Sort
Q14).What is the command used to store R objects in a file?
Ans-save (x, file=”x.Rdata”)
Q15).What is the best way to use Hadoop and R together for analysis?
Ans-HDFS can be used for storing the data for long-term. MapReduce jobs submitted from either Oozie, Pig or Hive can be used to encode, improve and sample the data sets from HDFS into R. This helps to leverage complex analysis tasks on the subset of data prepared in R.