Bundle Multi (2-in-1) - R Programming
Master the art of Data Analysis & Visualization with R. Dive into the world of Statistical Computing, Data Science, Machine Learning, & VisualizationsPreview Bundle Multi (2-in-1) - R Programming course
Price Match Guarantee Full Lifetime Access Access on any Device Technical Support Secure Checkout   Course Completion Certificate93% Started a new career BUY THIS COURSE (
USD 23 USD 69 )-
88% Got a pay increase and promotion
Students also bought -
-
- Bundle Course - Data Visualization with Python and R
- 35 Hours
- USD 23
- 1858 Learners
-
- Bundle Multi (4-in-1) - Python Programming
- 120 Hours
- USD 28
- 3779 Learners
-
- Career Path - IT Consultant
- 200 Hours
- USD 45
- 5851 Learners
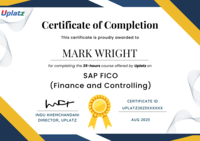
Courses included in Bundle Multi (2-in-1) - R Programming course by Uplatz are:
1) R programming
2) R programming (basic to advanced)
You will be awarded a Course Completion Certificate by Uplatz at the end of the course.
R is a programming language and software environment primarily used for statistical computing and graphics. It provides a wide range of tools and libraries for data manipulation, analysis, and visualization. R is highly popular among statisticians, data scientists, and researchers due to its extensive capabilities and open-source nature. R is a statistical analysis, graphical representation, and reporting computer language and software environment.
R is a programming language and an analytics tool that was developed in 1993 by Robert Gentleman and Ross Ihaka at the University of Auckland, Auckland, New Zealand. It is extensively used by Software Programmers, Statisticians, Data Scientists, and Data Miners. It is one of the most popular analytics tools used in Data Analytics and Business Analytics. It has numerous applications in domains like healthcare, academics, consulting, finance, media, and many more. Its vast applicability in Statistics, Data Visualization, and Machine Learning have given rise to the demand for certified trained professionals in R. It is used to make our data more understandable by representing data in graphical form. Visualization makes it easy to recognize patterns, find trends, and exceptions in our data. It enables us to convey information and results in a quick and visual way.
Here are some key features and concepts related to R programming:
1).Data Structures: R supports various data structures such as vectors, matrices, arrays, lists, and data frames. These structures allow you to store and manipulate different types of data efficiently.
2).Data Manipulation: R provides powerful tools for data manipulation, including subsetting, filtering, merging, and transforming datasets. The dplyr package is widely used for these tasks.
3).Data Analysis: R offers numerous packages for statistical analysis. Some popular ones include stats (for basic statistical functions), ggplot2 (for data visualization), and caret (for machine learning).
4).Packages: R has a vast ecosystem of packages contributed by the R community. Packages extend the functionality of R by providing additional tools and methods for specific tasks. You can install and load packages using the install.packages() and library() functions, respectively.
5).Data Visualization: R has excellent capabilities for creating static and interactive visualizations. The ggplot2 package is widely used for creating publication-quality plots, while packages like plotly and ggvis enable interactive and web-based visualizations.
6).R Markdown: R Markdown is an R-based framework that combines code, text, and visualizations in a single document. It allows you to create reproducible reports, presentations, and even web applications.
7).Machine Learning: R provides a wide range of machine learning algorithms and frameworks. The caret, randomForest, and e1071 packages are commonly used for building predictive models.
8).Community and Support: R has a large and active community of users who contribute to the development of packages, provide support, and share their knowledge through forums, blogs, and online tutorials.
In this R programming bundle course by Uplatz , you will master the basics of this widely used open source language i.e. R, including factors, lists, and data frames. With the knowledge gained in this course, you will be ready to undertake your first very own data analysis. Every year, the number of R users grows by about 40%, and an increasing number of organizations are using it in their day-to-day activities. Begin your journey to learn R with us today! This course is for software programmers, statisticians, and data miners who want to learn how to create statistical software using the R programming language.
R programming language as a beginner course will provide you with a sufficient grasp of virtually all of the language's principles, allowing you to progress to greater levels of competence. Because this R course is for beginners, no prior knowledge of computer programming terminology is required. This introductory course will assist you in grasping the fundamentals of R programming and progressing to more advanced topics.
The R programming course covers practical issues in statistical computing which includes programming in R, reading data into R, accessing R packages, writing R functions, debugging, profiling R code, and organizing and commenting R code. Topics in statistical data analysis will provide working examples.
Course/Topic 1 - R Programming - all lectures
-
In this lecture session we learn about basic introduction of R programming for beginners and also talk about basic functions of R programming for beginners.
-
In this tutorial we learn about how we install r programming in our software and also talk about the best way of installing R programming for beginners.
-
In this lecture session we learn about R's basic data structures including the vector, list, matrix, data frame, and factors. Some of these structures require that all members be of the same data type (e.g. vectors, matrices) while others permit multiple data types (e.g. lists, data frames). Objects may have attributes, such as name, dimension, and class.
-
In this lecture session we learn about A vector is the basic data structure in R, or we can say vectors are the most basic R data objects.
-
In this lecture session we learn about R is an ideal tool when it comes to data wrangling. It allows the usage of several preprocessed packages that makes data wrangling a lot more easier. This is one of the main reasons as to why R is preferred in the Data Science community.
-
In this lecture session we learn about R packages are a collection of R functions, compiled code and sample data. They are stored under a directory called "library" in the R environment. By default, R installs a set of packages during installation. More packages are added later, when they are needed for some specific purpose.
-
In this tutorial we learn about R is an open-source programming language that is widely used as a statistical software and data analysis tool.
-
In this lecture session we learn that R can be used as a powerful calculator by entering equations directly at the prompt in the command console. Simply type your arithmetic expression and press ENTER. R will evaluate the expressions and respond with the result.
-
In this tutorial we learn about Conditional statements are those statements where a hypothesis is followed by a conclusion. It is also known as an " If-then" statement.
-
In this tutorial we learn about In coding, you ask your computer to check conditions by writing conditional statements. Conditional statements are the way computers can make decisions.
-
In this lecture session we learn about It is a type of control statement that enables one to easily construct a loop that has to run statements or a set of statements multiple times. For loop is commonly used to iterate over items of a sequence.
-
In this lecture session we learn about Repeat loop, unlike other loops, doesn't use a condition to exit the loop instead it looks for a break statement that executes if a condition within the loop body results to be true.
-
In this lecture session we learn that Sum of n natural numbers can be defined as a form of arithmetic progression where the sum of n terms are arranged in a sequence with the first term being.
-
In this lecture session we learn about The formula to find the sum of n terms in AP is Sn = n/2 (2a+(n−1)d), in which a = first term, n = number of terms, and d = common difference between consecutive terms.
-
In this tutorial we learn about A switch statement that allows a variable to be tested for equality against a list of values. Each value is called a case, and the variable being switched on is checked for each case.
-
In this lecture session we learn about Data preprocessing, a component of data preparation, describing any type of processing performed on raw data to prepare it for another data processing procedure.
-
In this tutorial we learn about Data preprocessing is essential before its actual use. Data preprocessing is the concept of changing the raw data into a clean data set. The dataset is preprocessed in order to check missing values, noisy data, and other inconsistencies before executing it to the algorithm.
-
In this lecture session we learn about Factor in R is a variable used to categorize and store the data, having a limited number of different values. It stores the data as a vector of integer values. Factor in R is also known as a categorical variable that stores both string and integer data values as levels.
-
In this tutorial we learn about A data frame is a table or a two-dimensional array-like structure in which each column contains values of one variable and each row contains one set of values from each column.
-
In this tutorial we learn about In R we use merge() function to merge two dataframes in R. This function is present inside the join() function of the dplyr package.
-
In this lecture session we learn about The R merge function allows merging two data frames by common columns or by row names. This function allows you to perform different database (SQL) joins, like left join, inner join, right join or full join, among others.
-
In this tutorial we learn about The two data frames must have the same variables, but they do not have to be in the same order.
-
In this lecture session we learn about merge is a generic function whose principal method is for data frames: the default method coerces its arguments to data frames and calls the "data. frame" method. By default the data frames are merged on the columns with names they both have, but separate specifications of the columns can be given by.
-
In this lecture session we learn about The functions which are already created or defined in the programming framework are known as a built-in function. R has a rich set of functions that can be used to perform almost every task for the user.
-
In this lecture session we learn about Melting and Casting are one of the interesting aspects in R programming to change the shape of the data and further, getting the desired shape.
Course/Topic 2 - R Programming (basic to advanced) - all lectures
-
In this lecture session we learn about basic introduction to R programming and also talk about some key features of R programming.
-
In this lecture session we learn about the setup of R language in your system and also talk about the importance of R programming.
-
In this lecture session we learn about variables and data types in R language and also talk about types of variables and data types in R programming.
-
In this lecture session we learn about uses of variable and data types in our programs and also talk about some key features of variables and data types.
-
In this lecture session we learn about input - output features and also talk about features of input - output features.
-
In this lecture session we learn about posted function () in input output features and also talk about features of posted functions().
-
In this lecture session we learn about operators in R and also talk about features of operators in R programming.
-
In this lecture session we learn about different types of operators in R language and also talk about features of all types of operators in R.
-
In this lecture session we learn about vectors in data structure in R programming and also talk about features of vectors in data structures in brief.
-
In this lecture session we learn about the importance of vectors in data structure and also talk about vectors in data structures in brief.
-
In this lecture session we learn about list data structure in R programming and also talk about features of list in data structure.
-
In this lecture session we learn about more operations on the list and also talk about features of List in data structures in brief.
-
In this lecture session we learn about matrix in R programming and also talk about features of matrix in data structure in R language.
-
In this lecture session we learn about matrix in R programming and also talk about features of matrix in data structure in R language.
-
In this lecture session we learn about matrix data structure in R programming and also talk about some key features of matrix and data structure.
-
In this lecture session we learn about arrays in R programming and also talk about features of arrays.
-
In this lecture session we learn about different types of arrays in data structure and also talk about features of Arrays in data structure in brief.
-
In this lecture session we learn about data frame in R programming and also talk about function of data frame.
-
In this lecture session we learn about data frame features in R programming and also talk about the importance of data structure.
-
In this lecture session we learn about the importance of Data frame in brief and also talk about function of data frame in R programming.
-
In this lecture session we learn about data frame key features of data frame in data structure in brief.
-
In this lecture session we learn about factors data structures in R programming and also talk about the importance of factors.
-
In this lecture session we learn about factors of data structure in R programming and also talk about different types of factors in R language.
-
In this lecture session we learn about decision making in R programming and also talk about features of decision making in R.
-
In this lecture session we learn about different types of decision making statements and also talk about features of all decision statements.
-
In this lecture session we learn about decision making using integers and also talk about functions of integers.
-
In this lecture session we learn about Loops in R programming and also talk about factors of Loops in R language.
-
In this lecture session we learn about functions of Loops and why we need Loop statement in R programming and also talk about key features of Loop statement.
-
In this lecture session we learn about different types of Loops in R programming and also talk about features of For loop, while loop and do while loop.
-
In this lecture session we learn about functions in R programming language and also talk about features of functions in R language.
-
In this lecture session we learn about different types of functions in R programming and also talk about the importance of functions.
-
In this lecture session we learn about string in R programming and also talk about features of string function in R.
-
In this lecture session we learn about why we need strings in R programming and also talk about the importance of strings.
-
In this lecture session we learn about packages in R programming and also talk about features of packages in R.
-
In this lecture session we learn about data and file management in R programming and also talk about functions of data and file management.
-
In this lecture session we learn about how we manage the data and file in R programming and also talk about the importance of data and file management.
-
In this lecture session we learn about Line chart in R programming and also talk about features of line chart in brief.
-
In this lecture session we learn about scatterplot in R language and also talk about functions of scatters plot.
-
In this lecture session we learn about Pie chart in R programming and also talk about features of Pie Chart in brief.
-
In this lecture session we learn about bar charts in R language and also talk about features of Bar chart in brief.
-
In this lecture session we learn about how we use bar charts in R programming and also talk about features of Bar charts.
-
In this lecture session we learn about histogram in R programming and also talk about features of histogram.
-
In this lecture session we learn about Boxplots in R programming and also talk about features of Boxplot in R language.
You will learn about;
a).R Introduction
b).R Installation
c).R Data Structures
d).R Packages
e).Condition Statements in R
f).Sum Recursion
This course provides a comprehensive introduction to R programming, focusing on data analysis and statistical modeling. Participants will learn the fundamentals of R, data manipulation, visualization, and advanced statistical techniques.
Part 1: Introduction to R Programming
Week 1: Getting Started with R
a) Overview of R and its applications in data analysis
b) Installing R and RStudio
c) Introduction to R syntax and data types (vectors, lists, matrices, data frames)
d) Hands-on exercise: Setting up R and exploring basic commands
Week 2: Data Structures and Basic Operations
a) Understanding data structures in R: vectors, lists, matrices, and data frames
b) Basic operations: indexing, subsetting, and modifying data structures
c) Importing and exporting data from various formats (CSV, Excel)
d) Hands-on exercise: Working with different data structures
Week 3: Data Manipulation with dplyr and tidyr
a) Introduction to the tidyverse ecosystem
b) Data manipulation with dplyr: filter, select, mutate, summarize, and arrange
c) Data cleaning and reshaping with tidyr: gather, spread, separate, and unite
d) Hands-on exercise: Cleaning and transforming a dataset using dplyr and tidyr
Week 4: Data Visualization with ggplot2
a) Introduction to data visualization principles
b) Creating basic plots with ggplot2: scatter plots, bar charts, histograms
c) Customizing plots: themes, labels, and colors
d) Hands-on exercise: Building a comprehensive visualization using ggplot2
Part 2: Statistical Analysis and Advanced R
Week 5: Statistical Foundations
a) Overview of descriptive and inferential statistics
b) Introduction to probability distributions (normal, binomial, Poisson)
c) Hypothesis testing concepts: t-tests, chi-squared tests, ANOVA
d) Hands-on exercise: Conducting statistical tests in R
Week 6: Regression Analysis
a) Understanding linear regression and its assumptions
b) Implementing linear regression models in R
c) Evaluating model performance and diagnostics
d) Hands-on exercise: Building and analyzing a linear regression model
Week 7: Advanced Statistical Modeling
a) Introduction to generalized linear models (GLMs)
b) Overview of logistic regression and its applications
c) Implementing GLMs in R and interpreting results
d) Hands-on exercise: Performing logistic regression analysis
Week 8: Working with Time Series Data
a) Introduction to time series analysis and forecasting
b) Handling time series data in R (ts, zoo, xts packages)
c) Implementing basic forecasting models (ARIMA, Exponential Smoothing)
d) Hands-on exercise: Analyzing and forecasting time series data
Part 3: Capstone Project and Real-World Applications
Week 9: Integrating R with Other Tools
a) Overview of R Markdown for report generation
b) Introduction to Shiny for building interactive web applications
c) Integrating R with databases and APIs
d) Hands-on exercise: Creating a simple Shiny app
Week 10: Capstone Project
a) Overview of capstone project objectives
b) Participants will design and implement a data analysis project using R
c) Presentation of projects and peer reviews
d) Discussion on challenges faced and lessons learned
Recommended Resources:
Textbooks:
1) "R for Data Science" by Hadley Wickham and Garrett Grolemund
2) "The Art of R Programming" by Norman Matloff
Online Resources:
1) DataCamp, Coursera, and edX for supplementary courses and exercises
2) RStudio documentation and community forums for support
Tools:
1) R and RStudio for hands-on practice
Assessment:
1) Weekly quizzes and assignments
2) Mid-term project focused on a specific data analysis technique
3) Final capstone project showcasing a comprehensive data analysis solution
The R Programming Certification ensures you know planning, production and measurement techniques needed to stand out from the competition.
R is a programming language for statistical computing and graphics that you can use to clean, analyze, and graph your data. It is widely used by researchers from diverse disciplines to estimate and display results and by teachers of statistics and research methods.
R programming is a relatively simple scripting language and learning to use R to get statistical packages is not hard. Also commonly used in data science, R has a simple syntax that is easy to learn. However, the R programming language has some inconsistencies, which can make learning hard.
Bundle Multi (2-in-1) - R Programming makes you an expert in data analytics using the R programming language. This data science with R course enables you to take your Data Science skills into a variety of companies, helping them analyze data and make more informed business decisions.
R is now considered one of the most popular analytics tools in the world. In this certificate program you will develop the skill set necessary to perform key aspects of data science efficiently.
Uplatz online training guarantees the participants to successfully go through the R Programming certification provided by Uplatz. Uplatz provides appropriate teaching and expertise training to equip the participants for implementing the learnt concepts in an organization.
Course Completion Certificate will be awarded by Uplatz upon successful completion of the R Programming online course.
The R Programming draws an average salary of $105,080 per year depending on their knowledge and hands-on experience. The R Programming job roles are in high demand and make a rewarding career.
R programmers are most in-demand, especially in emerging startups. Some of the positions that are available for the R programmers are as follows: Data Scientist. Business Analyst.
Every job related to data is found to be a good fit for professionals who possess sound knowledge of the R programming language. There is a huge demand for R programmers in the market, and you can expect to get a decent job with a high salary in different fields.
Note that salaries are generally higher at large companies rather than small ones. Your salary will also differ based on the market you work in.
The following are the job titles:
a).R programmer.
b).Data Scientist.
c).Data Analyst.
d).Data Architect.
e).Data Visualization Analyst.
f).Geo Statisticians.
g).Database Administrator.
h).Quantitative Analysis with R.
Q1)Explain what is R?
Ans-R is data analysis software which is used by analysts, quants, statisticians, data scientists and others.
Q2)List out some of the function that R provides?
Ans-The function that R provides are
a).Mean
b).Median
c).Distribution
d).Covariance
e).Regression
f).Non-linear
g).Mixed Effects
h).GLM
i).GAM. etc.
Q3)Explain how you can start the R commander GUI?
Ans-Typing the command, ("Rcmdr") into the R console starts the R commander GUI.
Q4)In R how you can import Data?
Ans-You use R commander to import Data in R, and there are three ways through which you can enter data into it
a).You can enter data directly via Data à New Data Set
b).Import data from a plain text (ASCII) or other files (SPSS, Minitab, etc.)
c).Read a data set either by typing the name of the data set or selecting the data set in the dialog box
Q5)Mention what does not ‘R’ language do?
Ans-
a).Though R programming can easily connects to DBMS is not a database
b).R does not consist of any graphical user interface
c).Though it connects to Excel/Microsoft Office easily, R language does not provide any spreadsheet view of data
Q6)Explain how R commands are written?
Ans-In R, anywhere in the program you have to preface the line of code with a #sign, for example
a).subtraction
b).division
c).note order of operations exists
Q7)How can you save your data in R?
Ans-To save data in R, there are many ways, but the easiest way of doing this is
Go to Data > Active Data Set > Export Active Data Set and a dialogue box will appear, when you click ok the dialogue box let you save your data in the usual way.
Q8)Mention how you can produce co-relations and covariances?
Ans-You can produce co-relations by the cor () function to produce co-relations and cov () function to produce covariances.
Q9)Explain what is t-tests in R?
Ans-In R, the t.test () function produces a variety of t-tests. T-test is the most common test in statistics and used to determine whether the means of two groups are equal to each other.
Q10)Explain what is With () and By () function in R is used for?
Ans-
1).With() function is similar to DATA in SAS, it apply an expression to a dataset.
2).BY() function applies a function to each level of factors. It is similar to BY processing in SAS.
Q11)What are the data structures in R that is used to perform statistical analyses and create graphs?
Ans-R has data structures like:
a).Vectors
b).Matrices
c).Arrays
d).Data frames
Q12)Explain general format of Matrices in R?
Ans-General format is
Mymatrix< - matrix (vector, nrow=r , ncol=c , byrow=FALSE,
dimnames = list ( char_vector_ rowname, char_vector_colnames))
Q13)In R how missing values are represented ?
Ans-In R missing values are represented by NA (Not Available), why impossible values are represented by the symbol NaN (not a number).
Q14)Explain what is transpose?
Ans-For re-shaping data before, analysis R provides various method and transpose are the simplest method of reshaping a dataset. To transpose a matrix or a data frame t () function is used.
Q15)Explain how data is aggregated in R?
Ans-By collapsing data in R by using one or more BY variables, it becomes easy. When using the aggregate() function the BY variable should be in the list.
Q16)What is the function used for adding datasets in R?
Ans-rbind function can be used to join two data frames (datasets). The two data frames must have the same variables, but they do not have to be in the same order.
Q17)What is the use of subset() function and sample() function in R?
Ans-In R, subset() functions help you to select variables and observations while through sample() function you can choose a random sample of size n from a dataset.
Q18)Explain how you can create a table in R without external file?
Ans-Use the code
myTable = data.frame()
edit(myTable)
This code will open an excel like spreadsheet where you can easily enter your data.