Data Visualization in R
You learn about R plot colors effectively and creating and saving complex plots in R.Preview Data Visualization in R course
View Course Curriculum Price Match Guarantee Full Lifetime Access Access on any Device Technical Support Secure Checkout   Course Completion Certificate93% Started a new career BUY THIS COURSE (
USD 17 USD 41 )-
97% Got a pay increase and promotion
Students also bought -
-
- Data Science with R
- 25 Hours
- USD 17
- 479 Learners
-
- Career Path - Business Analyst
- 120 Hours
- USD 45
- 612 Learners
-
- R Programming (basic to advanced)
- 20 Hours
- USD 17
- 361 Learners
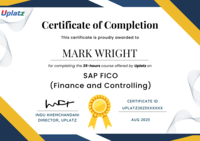
R is an open-sourced, well developed and simple programming language for statistical computing, data analysis, etc. R has inbuilt visualisation libraries that help to present & visualize data in a brief manner with the help of ggplot2, lattice, high charter, leaflets etc. ggplot2 makes it easier to build custom plots & help in producing multi-layered graphics. Lattice help in visualizing multi-varied data while high charters is a visualisation library in JavaScript. Data visualisation in R helps to build maps, 3D plots with the help of RGL and to understand the sequence of events.
R is an amazing platform for data analysis, capable of creating almost any type of graph. R is a language and environment for statistical computing and graphics. R is also extremely flexible and easy to use when it comes to creating visualisations. One of its capabilities of R is to produce good quality plots with minimum code.
Data visualization is an art of how to turn numbers into useful knowledge. R Programming lets you learn this art by offering a set of inbuilt functions and libraries to build visualizations and present data.
R supports four different graphics systems: base graphics, grid graphics, lattice graphics, and ggplot2. Base graphics is the default graphics system in R, the easiest of the four systems to learn to use, and provides a wide variety of useful tools, especially for exploratory graphics where we wish to learn what is in an unfamiliar dataset.
In this Data Visualization in R course by Uplatz, you will be able to learn the basics and introduction of fundamentals of how R uses an inbuilt visualization library to read multi-varied data, time-series data sets, maps etc. and makes it easier for the business to extract valuable information.
This course provides a comprehensive introduction on how to plot data with R’s default graphics system, base graphics.
After an introduction to base graphics, we look at a number of R plotting examples, from simple graphs such as scatterplots to plotting correlation matrices. The course finishes with exercises in plot customization. This includes using R plot colors effectively and creating and saving complex plots in R.
Course/Topic 1 - Data Visualization in R - all lectures
-
In this introductory tutorial on Data Visualization in R Programming, you will learn about what is data visualization, the type of graph or chart one should select for data visualization, what is the importance and benefits of data visualization and finally what are the applications of data visualization.
-
In this video, you will learn how to work on the Histogram, which falls under different Chart types used in Data Visualization in R Programming; along with working on the bar chart, box plot and heat map. You will be seeing a detailed explanation by the instructor on the complete workaround of these by taking different examples.
-
In this video, you will learn what is density plot and how you can create the density plot by taking different examples for it. You will also learn about the different applications being used in the density plot under Data Visualization with R Programming.
-
In this tutorial, you will learn about Data Visualization with GGPLOT2 Package where inside it you will learn the overview of GGPLOT2, iteratively building plots, univariate distributions and bar plot, annotation with GGPLOT2, axis manipulation and the density plot. You will get a complete understanding of the theoretical concept along with the implementation of each of these.
-
In this second part of the video tutorial, you will learn about Plotting with GGPLOT2 and building your plots iteratively, along with the importance of the ‘+’ symbol and its use in the GGPLOT2 work process. You will be seeing a detailed explanation from the instructor by taking different examples.
-
In this video you will learn about the complete theoretical and practical implementation of Univariate Distribution and Bar Plot, which can be seen explained in complete details by the instructor throughout the tutorial.
-
In this tutorial, you will learn about annotation with ggplot2, along with geom text () and adding labels with geom label () with complete explanation on this by the instructor with the help of different examples.
-
In this tutorial, you will learn about Axis Manipulation with ggplot2, its complete overview and in-depth concepts along with the different functions used during the process. You will be seeing explaining the topic in complete details by the instructor by taking examples and working in R studio.
-
In this section, you will learn about Text Mining and Word Cloud, along with the Radar Chart, Waffle Chart, Area Chart and the Correlogram. In this first part of the video, you will learn about the Text Mining and Word Cloud, the different reasons behind using Word Cloud for text data, who is using Word Clouds and the various steps involved in creating word clouds.
-
In this video, you will learn how to execute data using redline function. Also, you will understand the usage of corpus function and content transformer function. Further, you will understand about the text stemming, Term Document Matrix function and the Max word’s function.
-
In this tutorial, you will learn about the Radar Chart, the function used in the Radar Chart which is gg Radar (), scales, mapping and the use label. Along with this, you will also learn how to create Radar Chart in R studio. Moreover, you will learn about the Waffle Chart in R and how to create vector data in Waffle Chart with the help of different examples.
-
In this last part of the session, you will learn about the Area Chart, its in-depth concepts and how to work on it. This will be seen explained in detail by the instructor. Moreover, you will also learn about the Correlogram in R, the correlation matrix, Mt cars and the work around on different visualization methods been used.
-
This is a project tutorial titled Visualizing COVID-19 where you will see the different scenarios being explained by the tutor on visualizing COVID-19 data and how it can be done through Data Visualization in R process. In this first part, you will understand the complete overview of the project, its description and the different tasks associated with it being done by the ggplot.
-
In this second part of the project video, you will learn about the “Annotate” process and the number of COVID cases being reported in China with the help of Data Visualization. You will be seeing the task performed on the dataset being provided by the WHO along with understanding the tribble function and how it will help during the entire work process.
-
In this last part of the session, you will understand the work around of the task being done with the help of plot. You will see a detailed explanation by the instructor seeking help of few examples to explain the complete process of plotting in respect to the COVID-19 project being implemented.
Course/Topic 2 - Project on Data Visualization with R - all lectures
-
This is a project tutorial titled Visualizing COVID-19 where you will see the different scenarios being explained by the tutor on visualizing COVID-19 data and how it can be done through Data Visualization in R process. In this first part, you will understand the complete overview of the project, its description and the different tasks associated with it being done by the ggplot.
-
In this second part of the project video, you will learn about the “Annotate” process and the number of COVID cases being reported in China with the help of Data Visualization. You will be seeing the task performed on the dataset being provided by the WHO along with understanding the tribble function and how it will help during the entire work process.
-
In this last part of the session, you will understand the work around of the task being done with the help of plot. You will see a detailed explanation by the instructor seeking help of few examples to explain the complete process of plotting in respect to the COVID-19 project being implemented.
• To be familiar with standard techniques for visualizing data, including heat maps, contour plots, etc.
• To be able to transform raw data into formats suitable for analysis
• To be able to perform basic exploratory analysis
• To be able to create data visualizations in R
• Manage different types of data (logical, numeric, text, integer).
• Manage different data structures / data form.
• Export, reshape and transform your data.
• R Programming focus on data processing, data visualization and data science.
• Import data in R from any sources (Excel, SPSS, Stata.. or Website/Internet).
• Compute some key descriptive statistics.
• Understand the basic principles behind effective data visualization.
• Know why some graphs and figures work well, while others may fail to inform or actively mislead.
• Know how to refine plots for effective presentation.
• Know how to create a wide range of plots in R.
• Know how to save, report and communicate your results.
Data Visualisation in R
· What is data visualization?
· Selecting right chart type
· Importance of data visualization & its benefits
· Applications of DATA Visualization
· R Programs for Scatterplot, Histogram, Bar & Stacked bar chart, boxplot, heatmap, line chart, density plot, pie chart
Data Visualisation with ggplot2 package
· What is ggplot2
- Plotting with ggplot2
- Building your plots iteratively
- Univariate distributions & bar plot
- Annotation with ggplot2
- Axis manipulation
- Density plot
More Data Visualisation tools in R
· Text mining and word cloud
· Radar chart
· Waffle chart
· Area Chart
· Correlogram
Project on Data Visualisation
Most positions require a Bachelor's degree, though some companies, particularly in education, require you to have a Master's degree. Actual degrees are the usual ones considered useful for lower level data science jobs, including analytics-focused business degrees, computer science, and statistics.
On completing this Data Visualization in R course receive Course Completion Certificate from Uplatz.
Career Path:
R has become the lingua franca of Data Science and statistics. It is the most popular analytic tool. The estimated R users are nearing approximately 2 million. With the emergence of R programming in Data Science and data itself, there is a pressing need for efficient Data Science tools that can accommodate the needs of their users. Due to its platform independence, diversity of packages, and robust graphical features, it has become the primary tool for the analytics industry. R programmers can avail various types of jobs in the Data Science industry. Since there is a dearth of Data Scientists, both novice and professional R programmers can enter the Data Science industry.
R has become the lingua franca of Data Science and statistics. It is the most popular analytic tool. The estimated R users are nearing approximately 2 million. With the emergence of R programming in Data Science and data itself, there is a pressing need for efficient Data Science tools that can accommodate the needs of their users. Due to its platform independence, diversity of packages, and robust graphical features, it has become the primary tool for the analytics industry. R programmers can avail various types of jobs in the Data Science industry. Since there is a dearth of Data Scientists, both novice and professional R programmers can enter the Data Science industry.
There are various companies like Facebook, Google, and Twitter as well as emerging startups that are using R to meet their analytical goals. At the same time, organizations expect many of the new hires to be already equipped with knowledge of R. They want them to be familiar with the R tool and how to use it for Data Analysis.
Job Titles:
• Data Scientist
• Business Analyst
• Data Analyst
• Data Visualization Expert
• Quantitative Analyst
Q1.Compare R & Python.
Ans:
R programming Language |
Python programming language |
Model Building is similar to Python. |
Model Building is similar to R. |
Model Interpretability is good. |
Model Interpretability is not good. |
Production is not better than Python. |
Production is good. |
R has good community support over Python. |
Community Support is not better than R. |
Data Science Libraries are same as Python. |
Data Science Libraries are same as R. |
R has good data visualizations libraries and tools. |
Data visualization is not better than R. |
R has a steep learning curve. |
Learning Curve in Python is easier than learning R. |
Q2.Explain the data import in R language.
Ans:
R provides to import data in R language. To begin with the R commander GUI, user should type the commands in the command Rcmdr into the console. Data can be imported in R language in 3 ways such as:-
· Select the data set in the dialog box or enter the name of the data set as required.
· Data is entered directly using the editor of R Commander via Data->New Data Set. This works good only when the data set is not too large.
· Data can also be imported from a URL or from plain text file (ASCII), or from any statistical package or from the clipboard.
Q3.Explain how to communicate the outputs of data analysis using R language.
Ans:
Combine the data, code and analysis results in a single document using knitr for Reproducible research done. Helps to verify the findings, add to them and engage in conversations. Reproducible research makes it easy to redo the experiments by inserting new data values and applying it to different various problems.
4.What is R?
Ans:
R is a programming language which is used for developing statistical software and data analysis. It is being increasingly deployed for machine learning applications as well.
5.How R commands are written?
Ans:
By using # at the starting of the line of code like #division commands are written.
6.What is t-tests() in R?
Ans:
It is used to determine that the means of two groups are equal or not by using t.test() function.
7.What are the disadvantages of R Programming?
Ans:
The disadvantages are:-
· Lack of standard GUI.
· Not good for big data.
· Does not provide spreadsheet view of data.
8.What is the use of With () and By () function in R?
Ans:
1. with() function applies an expression to a dataset.
2. #with(data,expression)
3. By() function applies a function t each level of a factors.
4. #by(data,factorlist,function)
9.In R programming, how missing values are represented?
Ans:
In R missing values are represented by NA which should be in capital letters.
10.What is the use of subset() and sample() function in R?
Ans:
Subset() is used to select the variables and observations and sample() function is used to generate a random sample of the size n from a dataset.
11.Explain what is transpose.
Ans:
Transpose is used for reshaping of the data which is used for analysis. Transpose is performed by t() function.
12.What are the advantages of R?
Ans:
The advantages are:-
· It is used for managing and manipulating of data.
· No license restrictions.
· Free and open source software.
· Graphical capabilities of R are good.
· Runs on many Operating system and different hardware and also run on 32 & 64 bit processors etc.
13.What is the function used for adding datasets in R?
Ans:
For adding two datasets rbind() function is used but the column of two datasets must be same.
Syntax: rbind(x1,x2……) where x1,x2:
14.How you can produce co-relations and covariances?
Ans:
Cor-relations is produced by cor() and covariances is produced by cov() function.
15.What is difference between matrix and dataframes?
Ans:
Dataframe can contain different type of data but matrix can contain only similar type of data. Here are the different types of data structures in R:
16.What is difference between lapply and sapply?
Ans:
lapply is used to show the output in the form of list whereas sapply is used to show the output in the form of vector or data frame.
17.What is the difference between seq(4) and seq_along(4)?
Ans:
Seq(4) means vector from 1 to 4 (c(1,2,3,4)) whereas seq_along(4) means a vector of the length(4) or 1(c(1)).
18.Explain how you can start the R commander GUI.
Ans:
rcmdr command is used to start the R commander GUI.
19.What is the memory limit of R?
Ans:
In 32 bit system memory limit is 3Gb but most versions limited to 2Gb and in 64 bit system memory limit is 8Tb.
20.Explain how data is aggregated in R.
Ans:
There are two methods that is collapsing data by using one or more BY variable and other is aggregate() function in which BY variable should be in list.