Deep Learning Using SAS Software
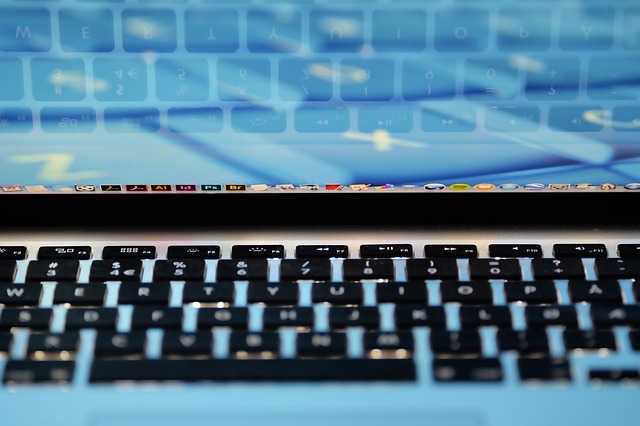
This course introduces the pivotal components of deep learning. You learn how to build deep feedforward, convolutional, recurrent networks, and variants of denoising autoencoders. The neural networks are used to solve problems that include traditional classification, image classification, and sequence-dependent outcomes. The course contains a healthy mix of theory and application. Hands-on demonstration and practice problems are included to reinforce key concepts. Hyperparameter search methods are described and demonstrated to find an optimal set of deep learning models. Transfer learning is covered in detail because of the emergence of this field has shown promise in deep learning. Lastly, you learn how to customize a SAS deep learning model to research new areas of deep learning.
Learn how to- Define and understand deep learning.
- Build models using deep learning techniques.
- Apply models to score (inference) new data.
- Modify data for better analysis results.
- Search the hyperparameter space of a deep learning model.
- Leverage transfer learning using supervised and unsupervised methods.
Target Audience
Machine learners and those interested in deep learning, computer vision, or natural language processing
--------------------------------------------------------------------------
Deep Learning Using SAS Software
- Introduction to neural networks.
- Introduction to deep learning.
- ADAM optimization.
- Dropout.
- Batch normalization.
- Autoencoders.
- Building level-specific autoencoders (self-study).
- Applications.
- Input layers.
- Convolutional layers.
- Padding.
- Pooling layers.
- Traditional layers.
- Types of skip-layer connections.
- Image pre-processing and data enrichment.
- Training convolutional neural networks.
- Introduction.
- Recurrent neural networks overview.
- Sub-types of recurrent neural networks.
- Time series analysis using recurrent neural networks.
- Sentiment analysis using recurrent neural networks.
- Selecting hyperparameters.
- Hyperband.
- Types of transfer learning.
- Transfer learning basics.
- Transfer learning strategies.
- Transfer learning with unsupervised pretraining.
- Customizations with FCMP.